How Redundant are Redundant Encodings? Blindness in the Wild and Racial Disparity when Race is Unobserved.
FAccT(2023)
摘要
We address two emerging concerns in algorithmic fairness: (i) redundant encodings of race - the notion that machine learning models encode race with probability nearing one as the feature set grows - which is widely noted in theory, with little empirical evidence; and (ii) the lack of race and ethnicity data in many domains, where state-of-the-art remains (Naive) Bayesian Improved Surname Geocoding (BISG) that relies on name and geographic information. We leverage a novel and highly granular dataset of over 7.7 million patients' electronic health records to provide one of the first empirical studies of redundant encodings in a realistic health care setting and examine the ability to assess health care disparities when race may be missing. First, we show that machine learning (random forest) applied to name and geographic information can improve on BISG, driven primarily by better performance in identifying minority groups. Second, contrary to theoretical concerns about redundant encodings as undercutting anti-discrimination law's anti-classification principle, additional electronic health information provides little marginal information about race and ethnicity: race still remains measured with substantial noise. Third, we show how machine learning can enable the disaggregation of racial categories, responding to longstanding critiques of the government race reporting standard. Fourth, we show that an increasing feature set can differentially impact performance on majority and minority groups. Our findings address important questions for fairness in machine learning and algorithmic decision-making, enabling the assessment of disparities, tempering concerns about redundant encodings in one important setting, and demonstrating how bigger data can shape the accuracy of race imputations in nuanced ways.
更多查看译文
AI 理解论文
溯源树
样例
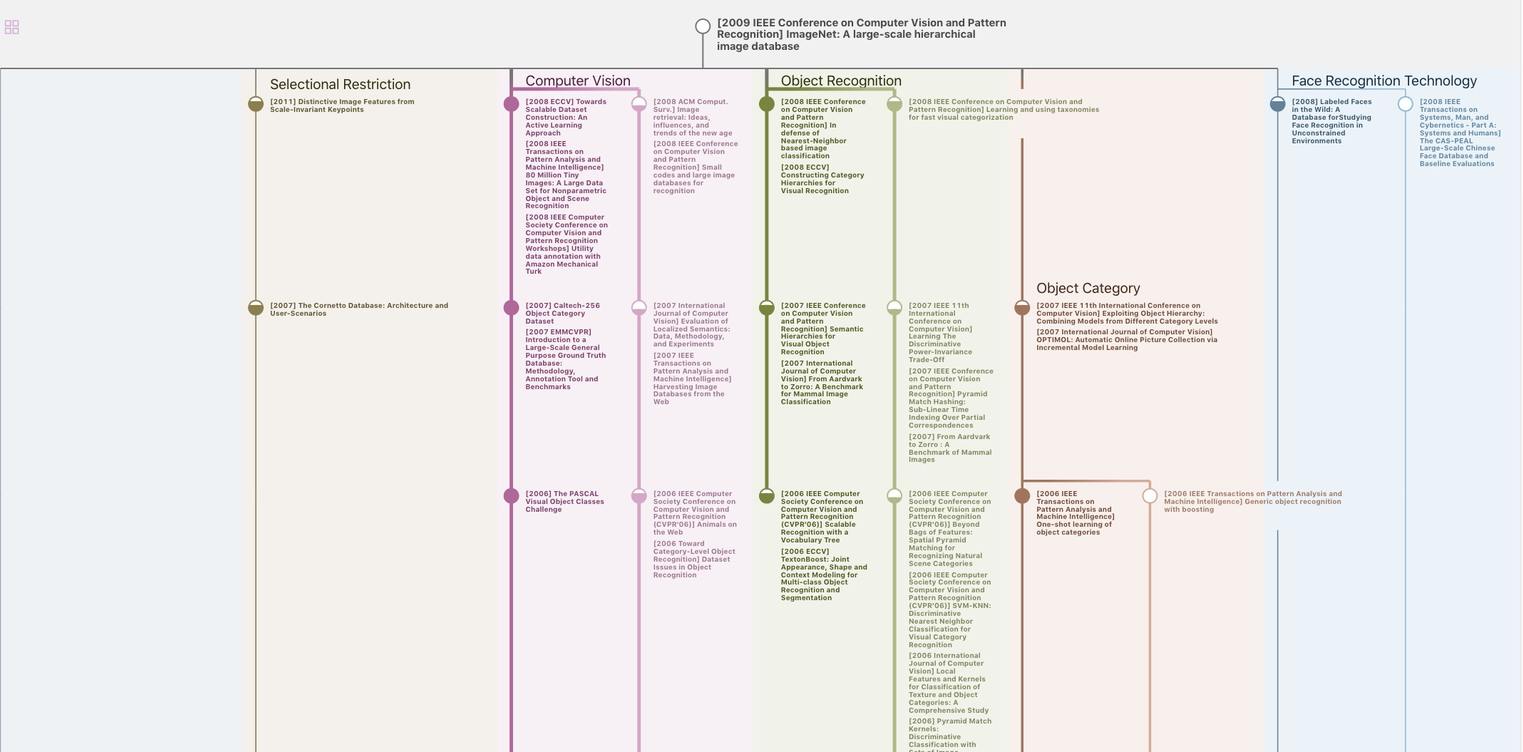
生成溯源树,研究论文发展脉络
Chat Paper
正在生成论文摘要