Parsing Causal Models - An Instance Segmentation Approach.
CAiSE Forum(2023)
摘要
The steadily growing number of publications in the field of information systems as well as the confusion arising from the naming of theoretical concepts, complicate the process of literature reviewing. While several knowledge repositories and databases are developed to combat this issue, a considerable amount of manual effort to populate the databases is required. The information these tools seek to present is often compactly summarized in causal models with a graph-like structure (e.g., structural equation models). Our work aims to develop a graph parsing method that reduces the amount of manual effort required and thus builds a foundation towards an augmentation of knowledge extraction from causal models. We contribute to the ongoing efforts in developing graph parsing tools by proposing a novel instance segmentation-based approach that leverages a new method to generate annotated synthetic graph images. Our solution is evaluated on a dataset of 166 images of structural equation models and outperforms existing graph parsing approaches in this use case.
更多查看译文
关键词
instance segmentation approach,causal models
AI 理解论文
溯源树
样例
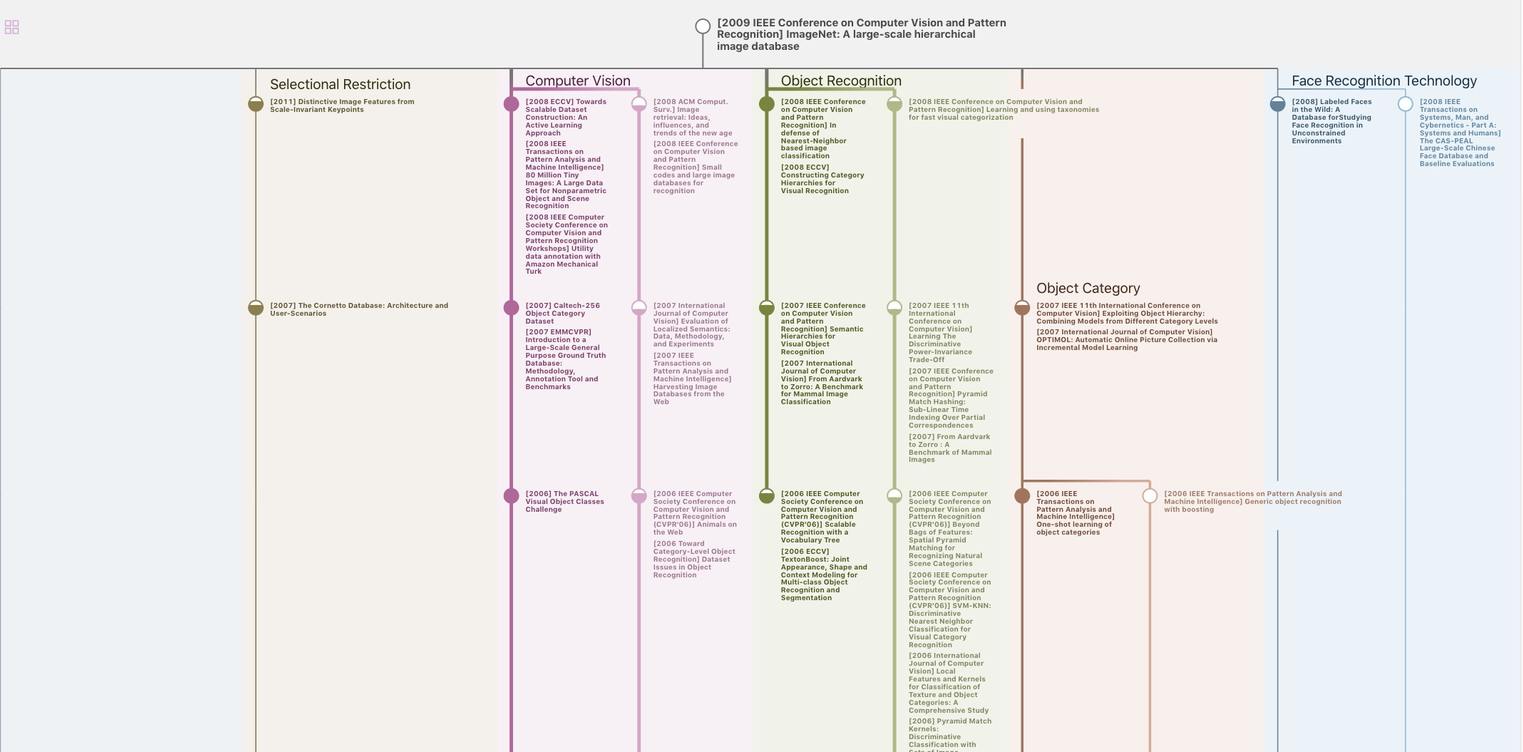
生成溯源树,研究论文发展脉络
Chat Paper
正在生成论文摘要