A Topological Data Analysis Framework for Computational Phenotyping.
AIME(2023)
摘要
Topological Data Analysis (TDA) aims to extract relevant information from the underlying topology of data projections. In the healthcare domain, TDA has been successfully used to infer structural phenotypes from complex data by linking patients who display demographic, clinical, and biomarker similarities. In this paper we propose pheTDA, a TDA-based framework to assist the computational definition of novel phenotypes. More in details, the pheTDA (i) guides the application of the Topological Mapper algorithm to derive a robust data representation as a topological graph; (ii) identifies relevant subgroups of patients from the topology; (iii) assess discriminative features for each subgroup of patients via predictive models. We applied the proposed tool on a population of 725 patients with suspected coronary artery disease (CAD). pheTDA identified five novel subgroups, one of which is characterized by the presence of diabetic patients showing high cardiovascular risk score. In addition, we compare the results obtained with existing clustering algorithms, showing that pheTDA obtains better performance when compared to spectral decomposition followed by k-means.
更多查看译文
关键词
topological data analysis framework,data analysis
AI 理解论文
溯源树
样例
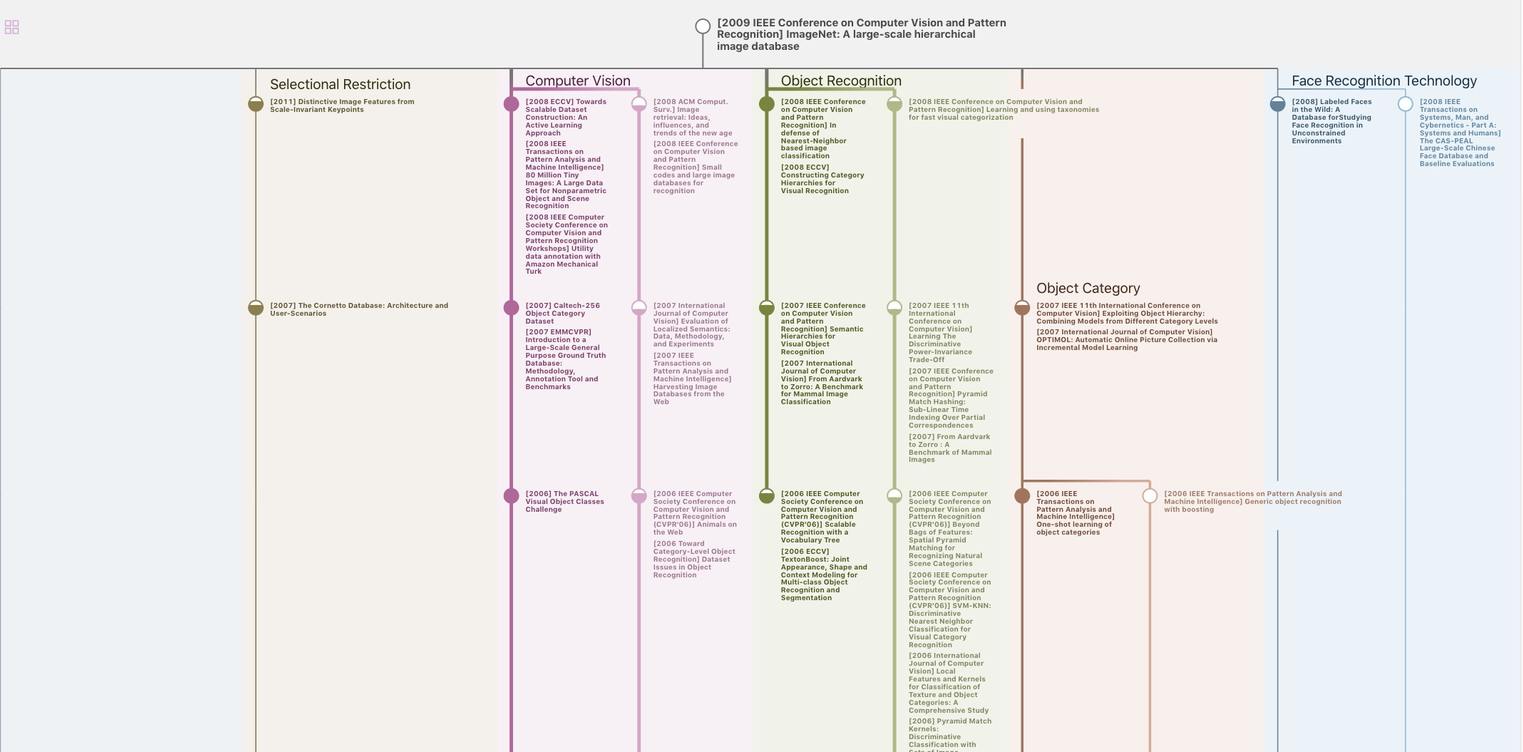
生成溯源树,研究论文发展脉络
Chat Paper
正在生成论文摘要