GGTWEAK: Gene Tagging with Weak Supervision for German Clinical Text.
AIME(2023)
摘要
Accurate extraction of biomolecular named entities like genes and proteins from medical documents is an important task for many clinical applications. So far, most gene taggers were developed in the domain of English-language, scientific articles. However, documents from other genres, like clinical practice guidelines, are usually created in the respective language used by clinical practitioners. To our knowledge, no annotated corpora and machine learning models for gene named entity recognition are currently available for the German language. In this work, we present GGTweak, a publicly available gene tagger for German medical documents based on a large corpus of clinical practice guidelines. Since obtaining sufficient gold-standard annotations of gene mentions for training supervised machine learning models is expensive, our approach relies solely on programmatic, weak supervision for model training. We combine various label sources based on the surface form of gene mentions and gazetteers of known gene names, with only partial individual coverage of the training data. Using a small amount of hand-labelled data for model selection and evaluation, our weakly supervised approach achieves an
$$F_1$$
score of 76.6 on a held-out test set, an increase of 12.4 percent points over a strongly supervised baseline. While there is still a performance gap to state-of-the-art gene taggers for the English language, weak supervision is a promising direction for obtaining solid baseline models without the need to conduct time-consuming annotation projects. GGTweak can be readily applied in-domain to derive semantic metadata and enable the development of computer-interpretable clinical guidelines, while the out-of-domain robustness still needs to be investigated.
更多查看译文
关键词
Clinical NLP, Gene Named Entity Recognition, German Language, Computer Interpretable Guidelines
AI 理解论文
溯源树
样例
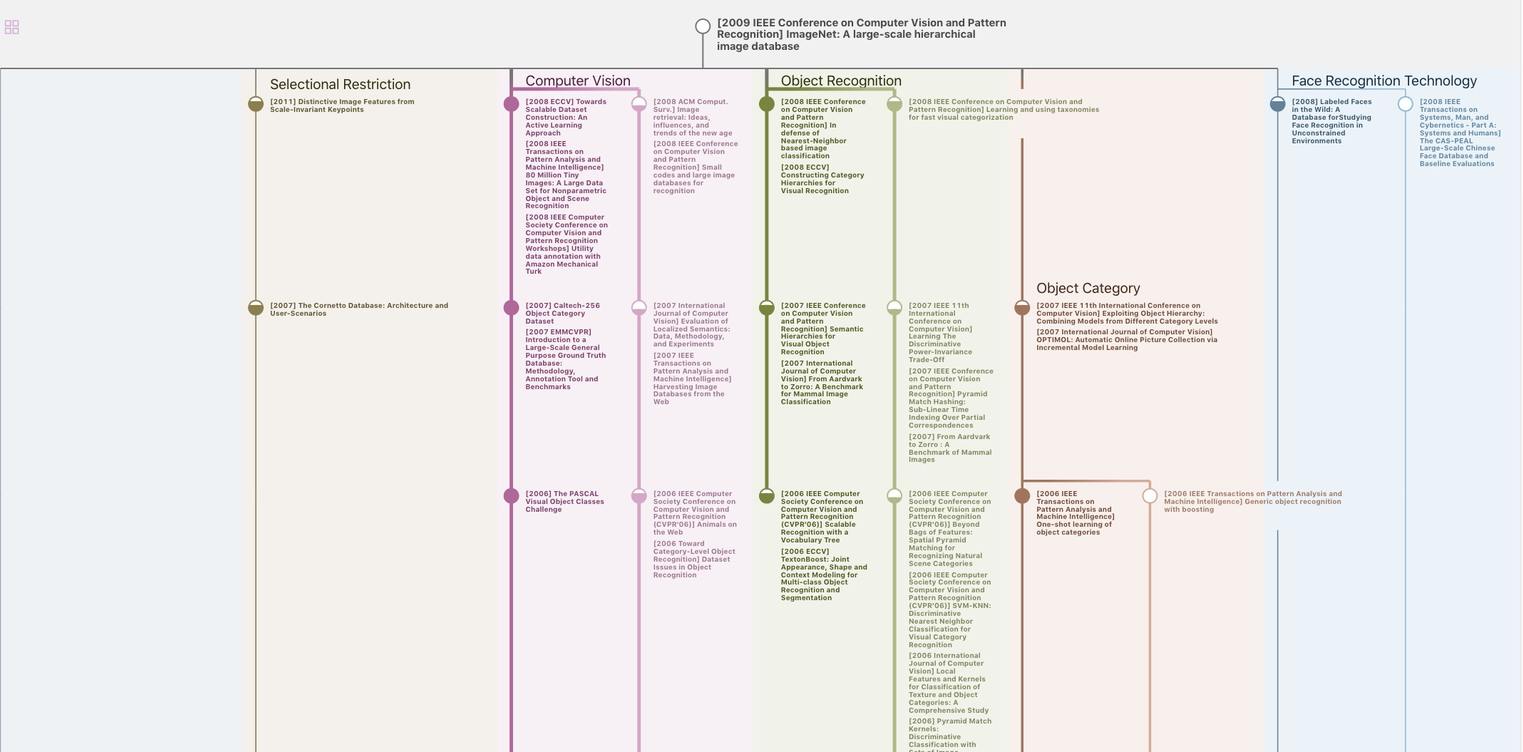
生成溯源树,研究论文发展脉络
Chat Paper
正在生成论文摘要