An Argumentative Framework for Generating Explainable Group Recommendations
2023 ADJUNCT PROCEEDINGS OF THE 31ST ACM CONFERENCE ON USER MODELING, ADAPTATION AND PERSONALIZATION, UMAP 2023(2023)
摘要
In the context of group recommender systems, explanations strategies have been proposed to improve recommendations perceived fairness, consensus, satisfaction, and to help the group members in the decision-making process. In general, such explanations try to clarify the underlying social chioce-based aggregation strategies used to generate the recommendations. However, results in the literature are conflicting, and the real benefit of such explanations seem to be limited. In this work, we propose a novel approach, which makes use of an argumentative framework built using information about the aspects that are connected to the recommended items. Such framework is used to generate recommendations, and related explanations. We provide a proof of concept on how to generate explanations for the group, as well as specific explanations for the group members, which use the information in the argumentative frameworks to enrich the explanations. Furthermore, we propose privacy-preserving versions for the explanations, as well as a graphical approach based on tag clouds. In future works, we plan to evaluate the quality of the provided recommendations in offline settings, as well as the impact of the proposed explanations in a series of user studies.
更多查看译文
关键词
Explainable AI,Explainable Recommender Systems,Group Recommender Systems,Argumentative Framework
AI 理解论文
溯源树
样例
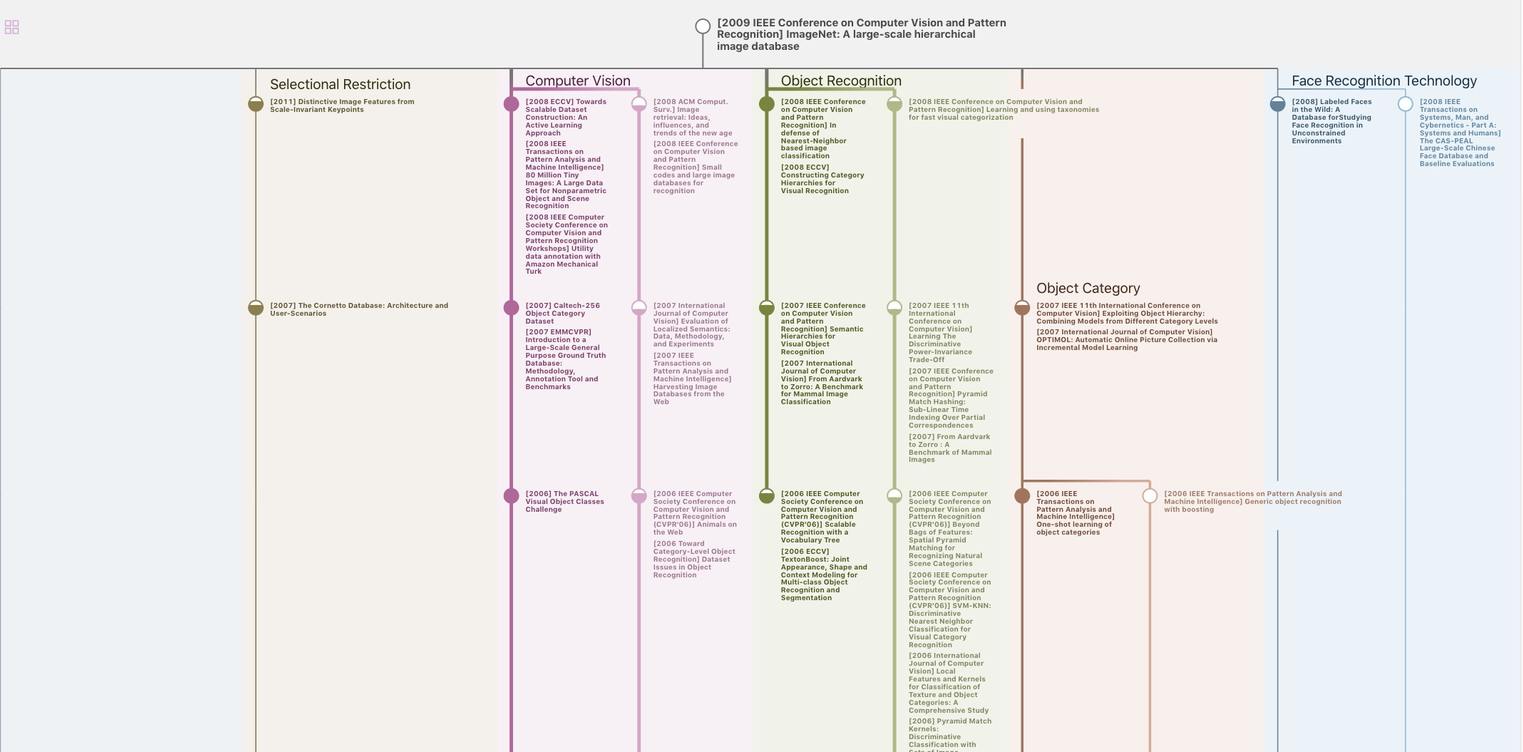
生成溯源树,研究论文发展脉络
Chat Paper
正在生成论文摘要