Semi-supervised segmentation of individual buildings from SAR imagery.
JURSE(2023)
摘要
Buildings are essential geo-targets that contribute to the monitoring of urban development. Synthetic aperture radar (SAR) provides excellent opportunities for building segmentation as it is insensitive to sunlight illumination and weather conditions. Nevertheless, the majority of existing approaches that exploit convolutional neural networks (CNNs), need to collect an enormous quantity of annotations for network training. Therefore, we propose an innovative semi-supervised method for individual building segmentation from SAR imagery. Our approach has three modules: a weights-shared encoder, a main decoder as well as an auxiliary decoder. For unlabeled samples, given the perturbation added to the encoder’s output, we enforce the consistency between the feature and output of the auxiliary decoder and those of the main decoder. This allows for the use of abundant unlabeled samples to make up for a lack of supervisory information. The experiments are carried out on a SAR dataset that is collected from the city of Berlin, Germany. Quantitative and qualitative results suggest that our approach is superior to other competitors.
更多查看译文
关键词
synthetic aperture radar(SAR),semi-supervised learning,building segmentation,convolutional neural network (CNN),urban
AI 理解论文
溯源树
样例
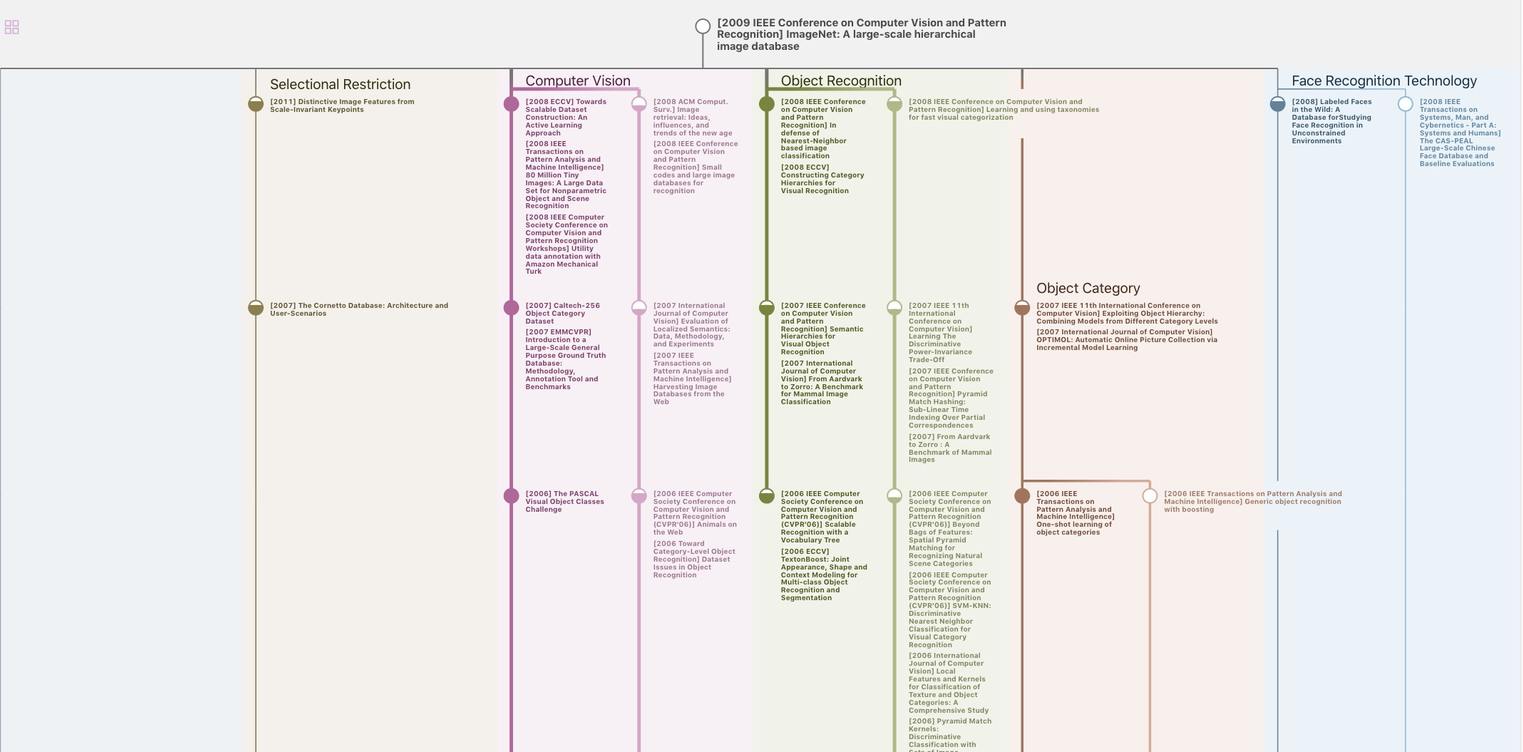
生成溯源树,研究论文发展脉络
Chat Paper
正在生成论文摘要