M5: Multi-modal Multi-task Model Mapping on Multi-FPGA with Accelerator Configuration Search
2023 DESIGN, AUTOMATION & TEST IN EUROPE CONFERENCE & EXHIBITION, DATE(2023)
摘要
Recent machine learning (ML) models have advanced from single-modality single-task to multi-modality multi-task (MMMT). MMMT models typically have multiple backbones of different sizes along with complicated connections, exposing great challenges for hardware deployment. For scalable and energy-efficient implementations, multi-FPGA systems are emerging as the ideal design choices. However, finding the optimal solutions for mapping MMMT models onto multiple FPGAs is non-trivial. Existing mapping algorithms focus on either streamlined linear deep neural network architectures or only the critical path of simple heterogeneous models. Direct extensions of these algorithms for MMMT models lead to sub-optimal solutions. To address these shortcomings, we propose M5, a novel MMMT Model Mapping framework for Multi-FPGA platforms. In addition to handling multiple modalities present in the models, M5 can flexibly explore accelerator configurations and possible resource sharing opportunities to significantly improve the system performance. For various computation-heavy MMMT models, experiment results demonstrate that M5 can remarkably outperform existing mapping methods and lead to an average reduction of 35%, 62%, and 70% in the number of low-end, mid-end, and high-end FPGAs required to achieve the same throughput, respectively. Code is publicly available(1).
更多查看译文
关键词
Multi-FPGA,DNN Model Mapping Framework
AI 理解论文
溯源树
样例
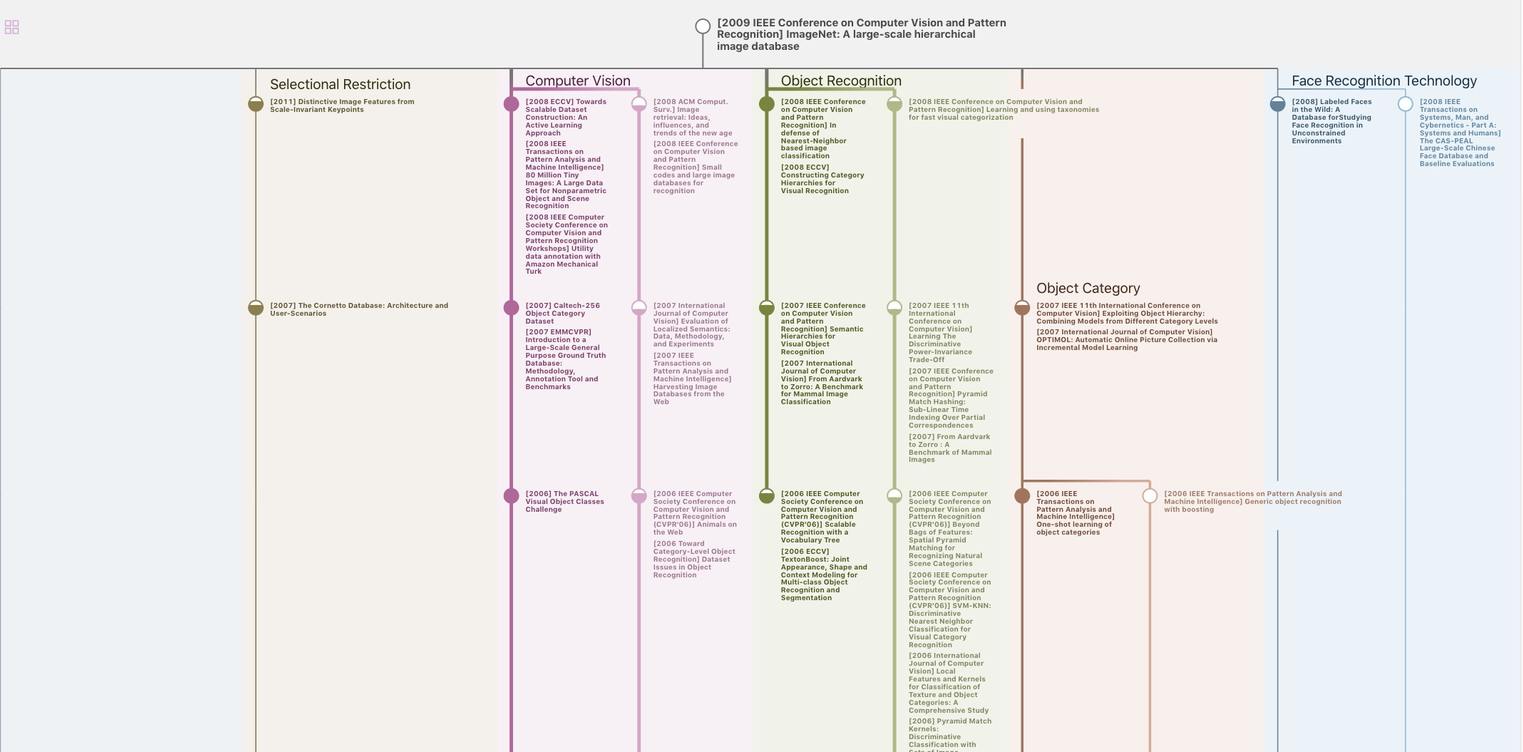
生成溯源树,研究论文发展脉络
Chat Paper
正在生成论文摘要