Multispectral Feature Fusion for Deep Object Detection on Embedded NVIDIA Platforms
2023 DESIGN, AUTOMATION & TEST IN EUROPE CONFERENCE & EXHIBITION, DATE(2023)
摘要
Multispectral images can improve object detection systems' performance due to their complementary information, especially in adverse environmental conditions. To use multispectral image data in deep-learning-based object detectors, a fusion of the information from the individual spectra, e.g., inside the neural network, is necessary. This paper compares the impact of general fusion schemes in the backbone of the YOLOv4 object detector. We focus on optimizing these fusion approaches for an NVIDIA Jetson AGX Xavier and elaborating on their impact on the device in physical metrics. We optimize six different fusion architectures in the network's backbone for the TensorRT framework and compare their inference time, power consumption, and object detection performance. Our results show that multispectral fusion approaches with little design effort can benefit resource usage and object detection metrics compared to individual networks.
更多查看译文
关键词
multispectral fusion,deep object detection,embedded hardware,NVIDIA Jetson
AI 理解论文
溯源树
样例
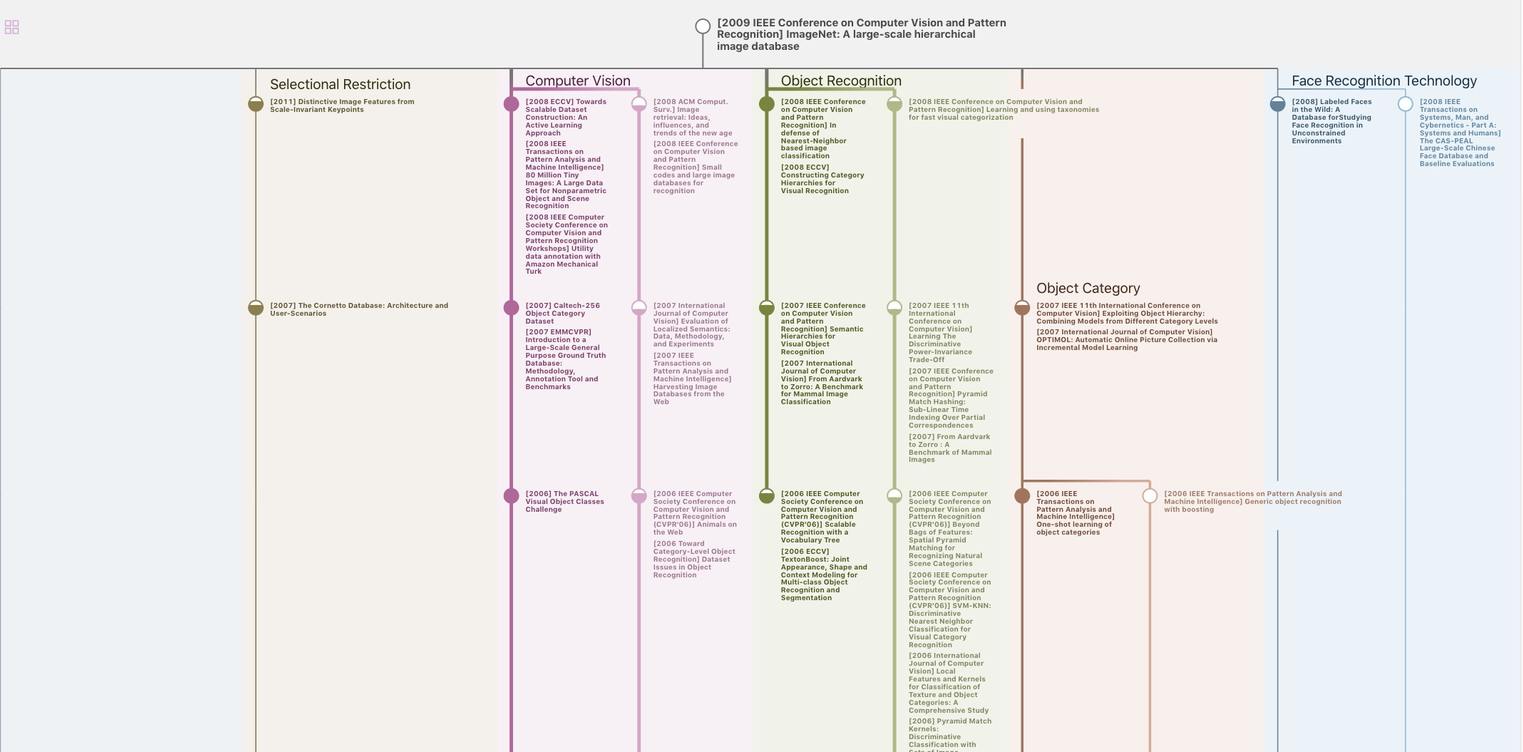
生成溯源树,研究论文发展脉络
Chat Paper
正在生成论文摘要