PEDAL: A Power Efficient GCN Accelerator with Multiple DAtafLows
2023 DESIGN, AUTOMATION & TEST IN EUROPE CONFERENCE & EXHIBITION, DATE(2023)
摘要
Graphs are ubiquitous in many application domains due to their ability to describe structural relations. Graph Convolutional Networks (GCNs) have emerged in recent years and are rapidly being adopted due to their capability to perform Machine Learning (ML) tasks on graph-structured data. GCN exhibits irregular memory accesses due to the lack of locality when accessing graph-structured data. This makes it hard for general-purpose architectures like CPUs and GPUs to fully utilize their computing resources. In this paper, we propose PEDAL, a power-efficient accelerator for GCN inference supporting multiple dataflows. PEDAL chooses the best-fit dataflow and phase ordering based on input graph characteristics and GCN algorithm, achieving both efficiency and flexibility. To achieve both high power efficiency and performance, PEDAL features a light-weight processing element design. PEDAL achieves 144.5x, 9.4x, and 2.6x speedup compared to CPU, GPU, and HyGCN, respectively, and 8856x, 1606x, 8.4x, and 1.8x better power efficiency compared to CPU, GPU, HyGCN, and EnGN, respectively.
更多查看译文
关键词
Accelerator,power-efficient,multiple dataflows
AI 理解论文
溯源树
样例
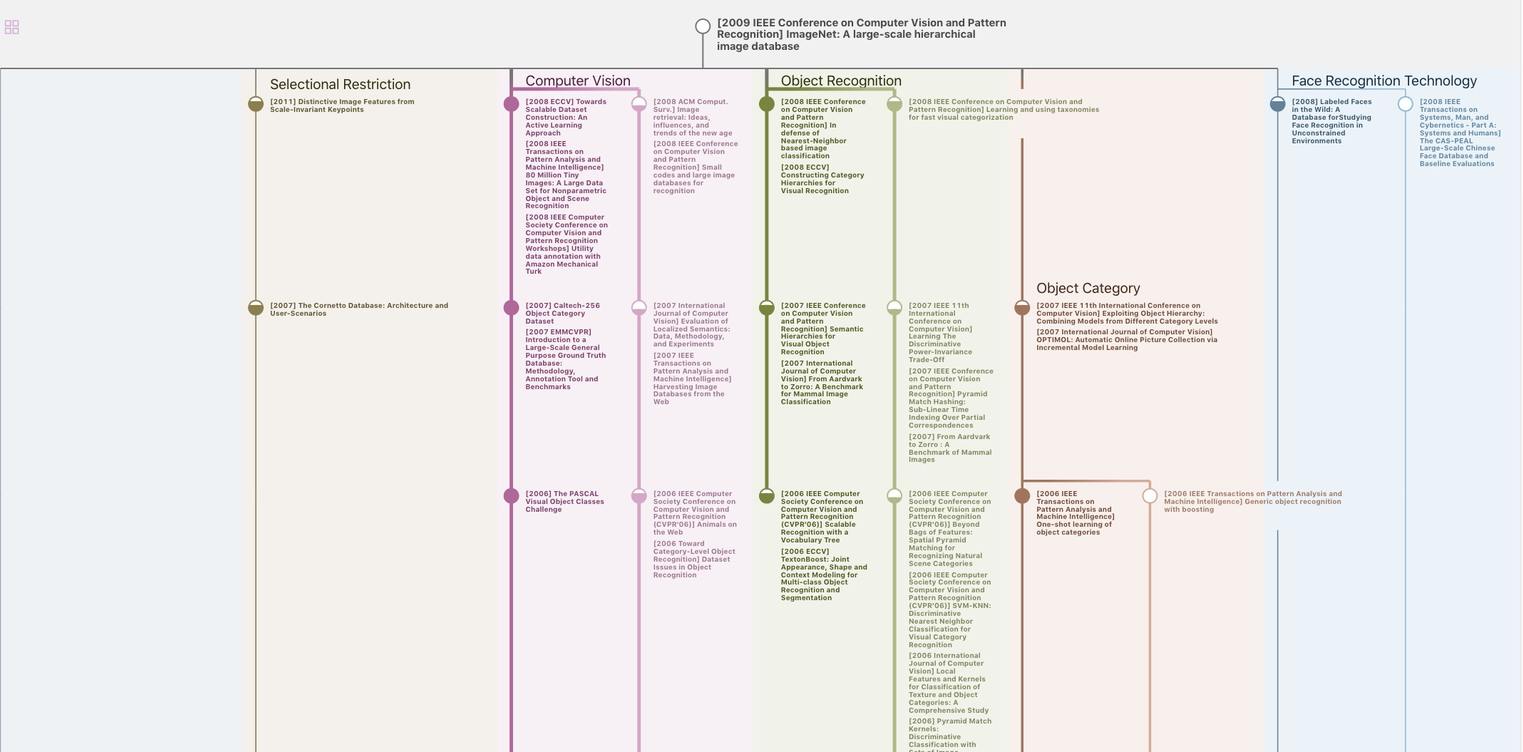
生成溯源树,研究论文发展脉络
Chat Paper
正在生成论文摘要