PRIVE: Efficient RRAM Programming with Chip Verification for RRAM-based In-Memory Computing Acceleration
2023 DESIGN, AUTOMATION & TEST IN EUROPE CONFERENCE & EXHIBITION, DATE(2023)
摘要
As deep neural networks (DNNs) have been successfully developed in many applications with continuously increasing complexity, the number of weights in DNNs surges, leading to consistent demands for denser memories than SRAMs. RRAM-based in-memory computing (IMC) achieves high density and energy-efficiency for DNN inference, but RRAM programming remains to be a bottleneck due to high write latency and energy consumption. In this work, we present the Progressive-wRite In-memory program-VErify (PRIVE) scheme, which we verify with an RRAM testchip for IMC-based hardware acceleration for DNNs. We optimize the progressive write operations on different bit positions of RRAM weights to enable error compensation and reduce programming latency/energy, while achieving high DNN accuracy. For 5-bit precision DNNs, PRIVE reduces the RRAM programming energy by 1.82x, while maintaining high accuracy of 91.91% (VGG-7) and 71.47% (ResNet-18) on CIFAR-10 and CIFAR-100 datasets, respectively.
更多查看译文
关键词
Deep neural network,resistive RAM (RRAM),in-memory computing,RRAM programming,write-verify
AI 理解论文
溯源树
样例
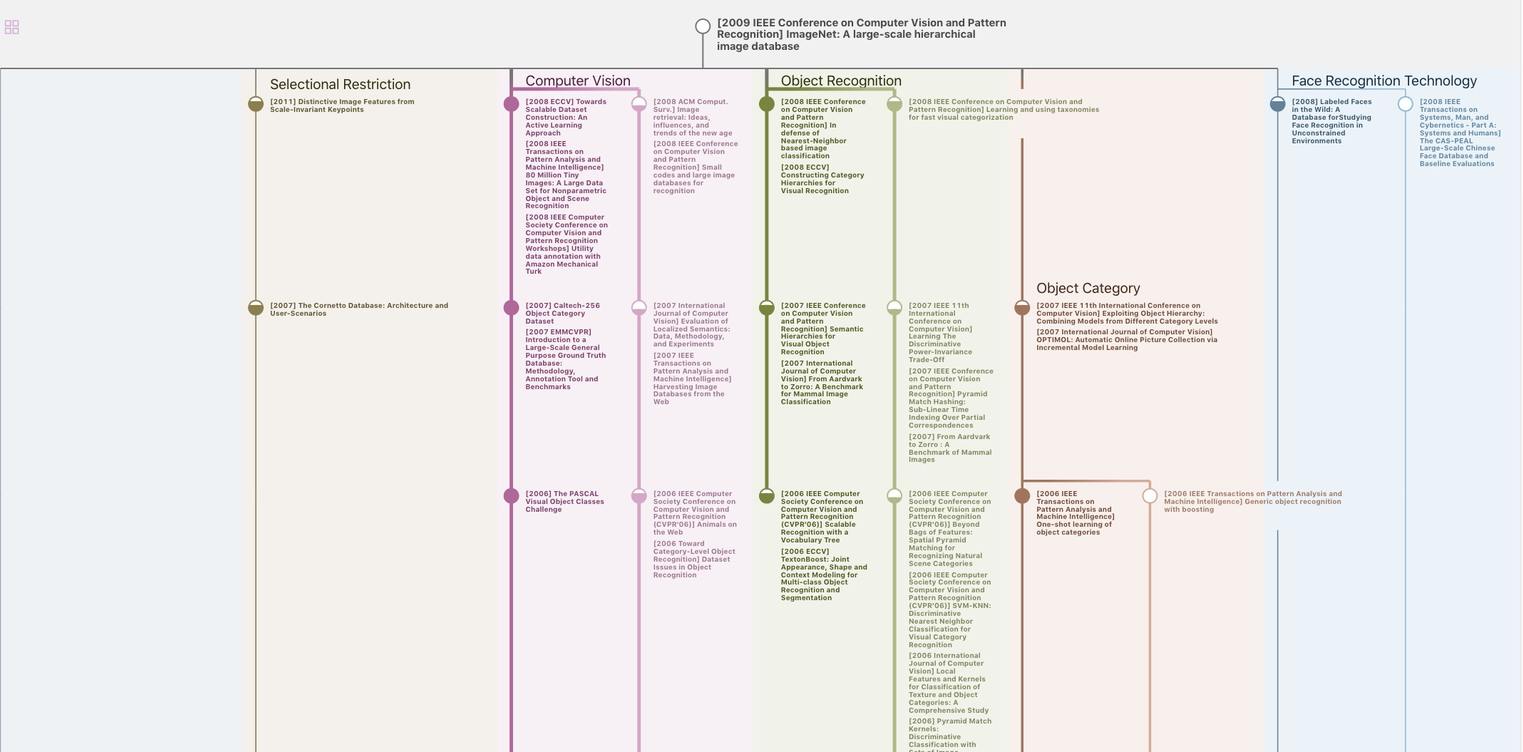
生成溯源树,研究论文发展脉络
Chat Paper
正在生成论文摘要