Machine learning assisted multipath signal parameter estimation and its evaluation under weak signal environment.
PLANS(2023)
摘要
Multipath is a major error source for global navigation satellite systems (GNSS) positioning, which is hard to be eliminated. This paper develops a machine learning (ML) assisted multipath signal parameter estimation to mitigate multipath interference. In this work, random forest (RF) is employed to operate on multiple samples with equal chip spacing of the autocorrelation function to obtain amplitude and code phase delay estimates of multipath. The direct-path signal is then restored by removing the estimated multipath components from the total received signal. The RF-based multipath estimation method is evaluated in one multipath scenario under weak signal environments with multipath estimation delay lock loop (MEDLL) as the benchmark. The simulation results show that the RF-based estimator has better parameter estimation and multipath mitigation performances than MEDLL in weak signal environments. It is also found that the proposed multipath signal parameter estimator performs well with limited number of correlators, demonstrating its feasibility.
更多查看译文
关键词
machine learning, random forest, multipath parameter estimation, weak signal environments
AI 理解论文
溯源树
样例
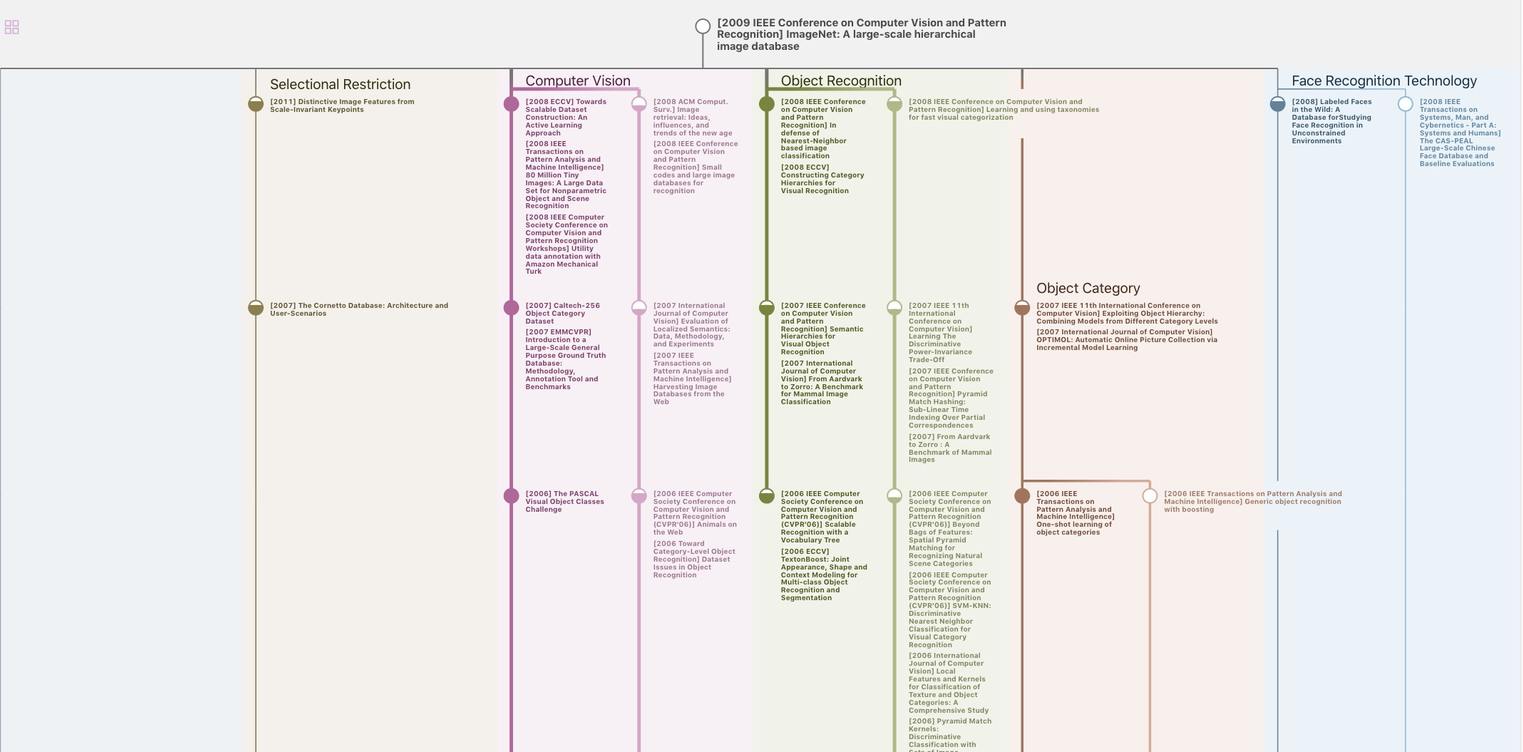
生成溯源树,研究论文发展脉络
Chat Paper
正在生成论文摘要