Solution Separation-based Integrity Monitor for Integrated GNSS/IMU/Camera Navigation: Constraining the Hypothesis Space With Deep Learning.
PLANS(2023)
摘要
This article introduces a method utilizing deep learning in typical Multiple Hypothesis Solution Separation (MHSS)-based Integrity Monitors (IMs) of autonomous vehicle navigation systems, when conventional sensors, such as GNSS and Inertial Measurement Unit (IMU), are integrated with a camera. It is an innovative methodology to reduce the hypothesis space of sensor faults. In the proposed method, the measurement subsets to evaluate in MHSS are generated from the IMU/GNSS measurement set only, so that Fault Detection and Exclusion (FDE) in camera measurements takes place separately. In the investigated approach, anomaly prediction in state estimate error due to camera faults is performed based on raw images with a Deep Neural Network (DNN), and IM input is required only during the online refinement of predicted anomaly locations to reflect anomalies in the IM test statistic. This opens for the possibility to evaluate environment features and conditions that cause specific detected or undetected sensor faults. Experiments on the IMU/GNSS/Camera integration demonstrated that Protection Level (PL) bounding performance of the proposed IM, with limited hypothesis space and the individual camera FDE, is comparable to the MHSS IM informed by the full set of fault hypotheses. Despite the use case of the camera, the method can be directly extended to integrations with multiple auxiliary sensors, where each auxiliary sensor is evaluated individually for faults.
更多查看译文
关键词
Integrity Monitor, Solution Separation, Deep Learning, Camera Navigation, Visual SLAM
AI 理解论文
溯源树
样例
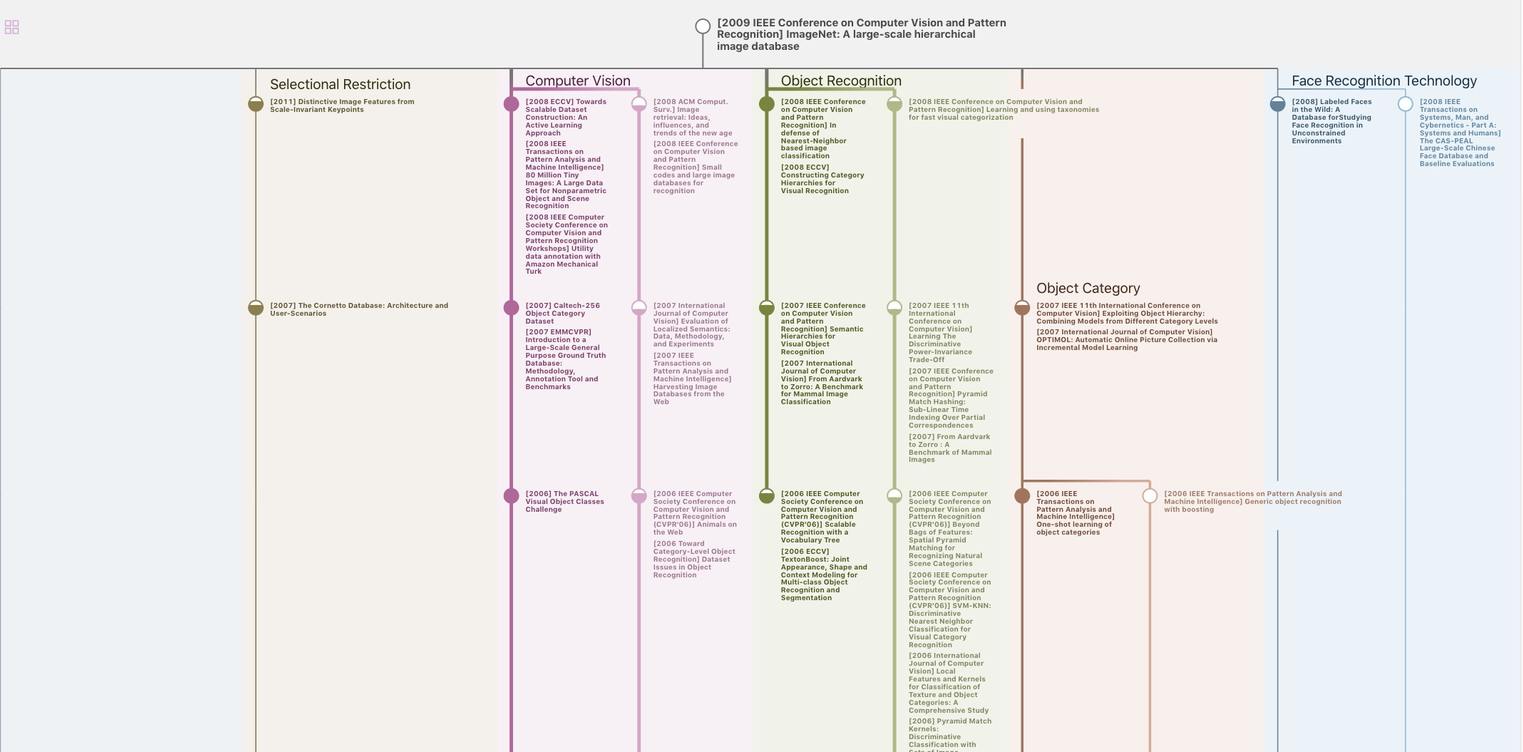
生成溯源树,研究论文发展脉络
Chat Paper
正在生成论文摘要