Image Quantization Tradeoffs in a YOLO-based FPGA Accelerator Framework.
ISQED(2023)
摘要
Until recently, FPGA-based acceleration of convolutional neural networks (CNNs) has remained an open-ended research problem. Herein, we evaluate one new method for rapidly implementing CNNs using industry-standard frameworks within Xilinx UltraScale+ FPGA devices. Within this workflow, referred to as Framework for Accelerating YOLO-Based ML on Edge-devices (FAYME), a TensorFlow model of the You Only Look Once version 4 (YOLOv4) object detection algorithm is realized using Xilinx’s Vitis AI toolchain. We test various levels of model bit-quantization and evaluate performance while simultaneously analyzing the utilization of available memory and processing elements. We also implement a ResNet-50 model to provide additional comparisons. In this paper, we present our YOLO model, which achieves a mAP of 0.581, and our ResNet model, which achieves a Top-5 accuracy of 0.950. Furthermore, we demonstrate that these results are possible while utilizing less than 25% of the throughput offered by a single hardware accelerator in an UltraScale+ FPGA.
更多查看译文
关键词
Field Programmable Gate Array (FPGA),Machine Learning Accelerator,Convolutional Neural Network (CNN),You Only Look Once (YOLO)
AI 理解论文
溯源树
样例
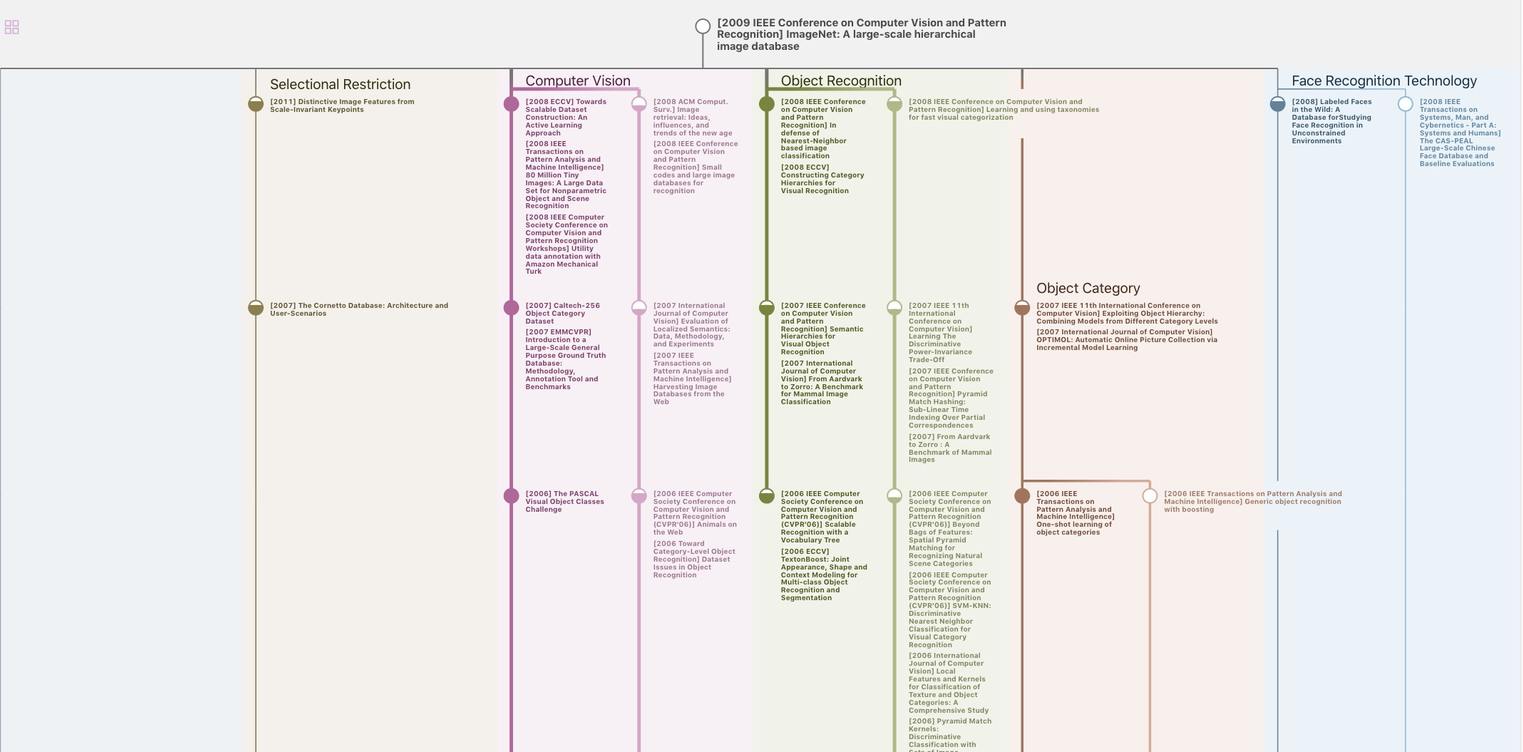
生成溯源树,研究论文发展脉络
Chat Paper
正在生成论文摘要