Phyllis: Physics-Informed Lifelong Reinforcement Learning for Data Center Cooling Control
PROCEEDINGS OF THE 2023 THE 14TH ACM INTERNATIONAL CONFERENCE ON FUTURE ENERGY SYSTEMS, E-ENERGY 2023(2023)
摘要
Deep reinforcement learning (DRL) has shown good performance in data center cooling control for improving energy efficiency. The main challenge in deploying the DRL agent to real-world data centers is how to quickly adapt the agent to the ever-changing system with thermal safety compliance. Existing approaches rely on DRL's native fine-tuning or a learned data-driven dynamics model to assist the adaptation. However, they require long-term unsafe exploration before the agent or the model can capture a new environment. This paper proposes Phyllis, a physics-informed reinforcement learning approach to assist the DRL agent's lifelong learning under evolving data center environment. Phyllis first identifies a transition model to capture the data hall thermodynamics in the offline stage. When the environment changes in the online stage, Phyllis assists the adaptation by i) supervising safe data collection with the identified transition model, ii) fitting power usage and residual thermal models, iii) pretraining the agent by interacting with these models, and iv) deploying the agent for further fine-tuning. Phyllis uses known physical laws to inform the transition and power models for improving the extrapolation ability to unseen states. Extensive evaluation for two simulated data centers with different system changes shows that Phyllis saves 5.7% to 13.8% energy usage compared with feedback cooling control and adapts to new environments 8x to 10x faster than fine-tuning with at most 0.74 degrees C temperature overshoot.
更多查看译文
关键词
Data centers,cooling control optimization,lifelong reinforcement learning,safe exploration,domain adaptation
AI 理解论文
溯源树
样例
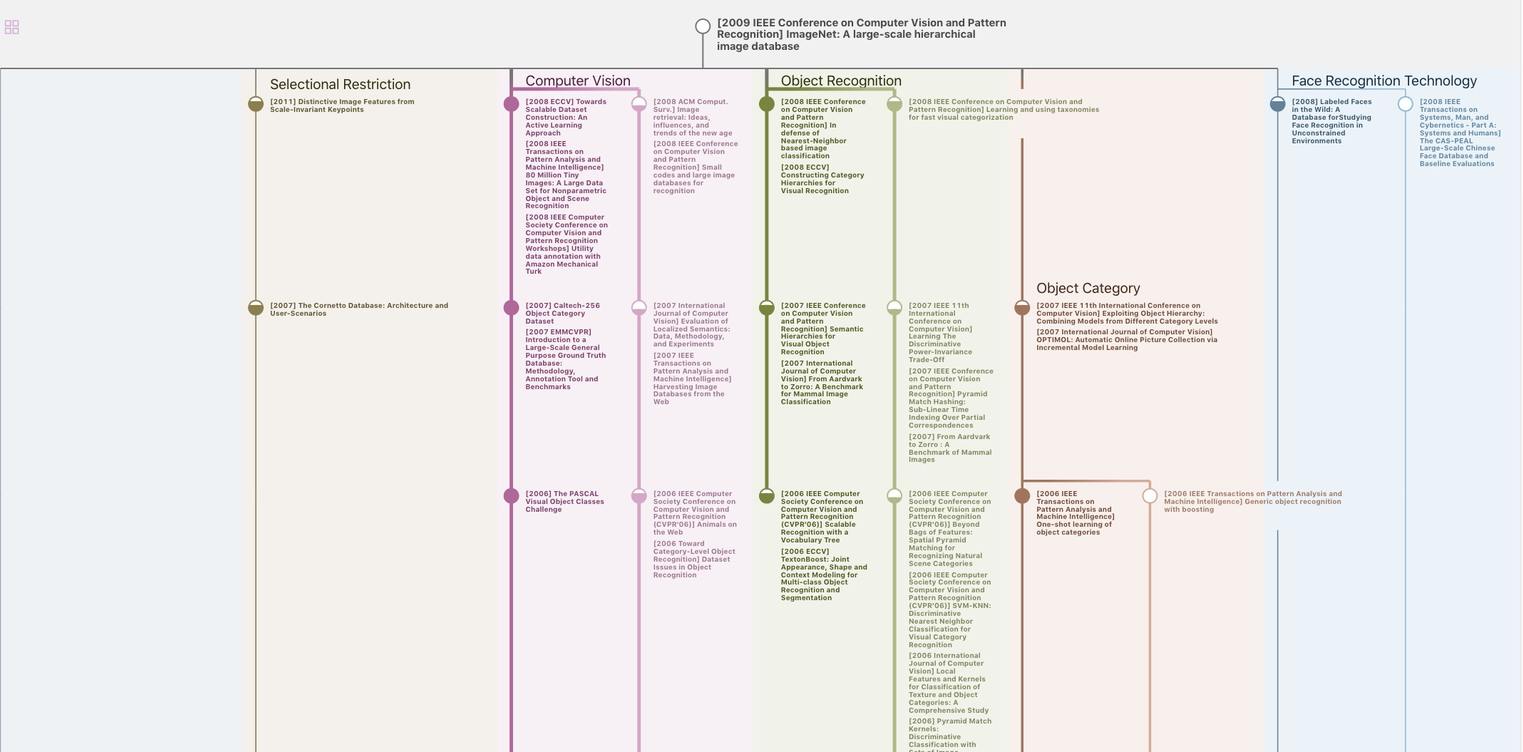
生成溯源树,研究论文发展脉络
Chat Paper
正在生成论文摘要