Learning Probabilistic Piecewise Rigid Atlases of Model Organisms via Generative Deep Networks.
IPMI(2023)
摘要
Atlases are crucial to imaging statistics as they enable the standardization of inter-subject and inter-population analyses. While existing atlas estimation methods based on fluid/elastic/diffusion registration yield high-quality results for the human brain, these deformation models do not extend to a variety of other challenging areas of neuroscience such as the anatomy of C. elegans worms and fruit flies. To this end, this work presents a general probabilistic deep network-based framework for atlas estimation and registration which can flexibly incorporate various deformation models and levels of keypoint supervision that can be applied to a wide class of model organisms. Of particular relevance, it also develops a deformable piecewise rigid atlas model which is regularized to preserve inter-observation distances between neighbors. These modeling considerations are shown to improve atlas construction and key-point alignment across a diversity of datasets with small sample sizes including neuron positions in C. elegans hermaphrodites, fluorescence microscopy of male C. elegans, and images of fruit fly wings. Code is accessible at https://github.com/amin-nejat/Deformable-Atlas.
更多查看译文
关键词
probabilistic piecewise rigid atlases,model organisms,networks,learning
AI 理解论文
溯源树
样例
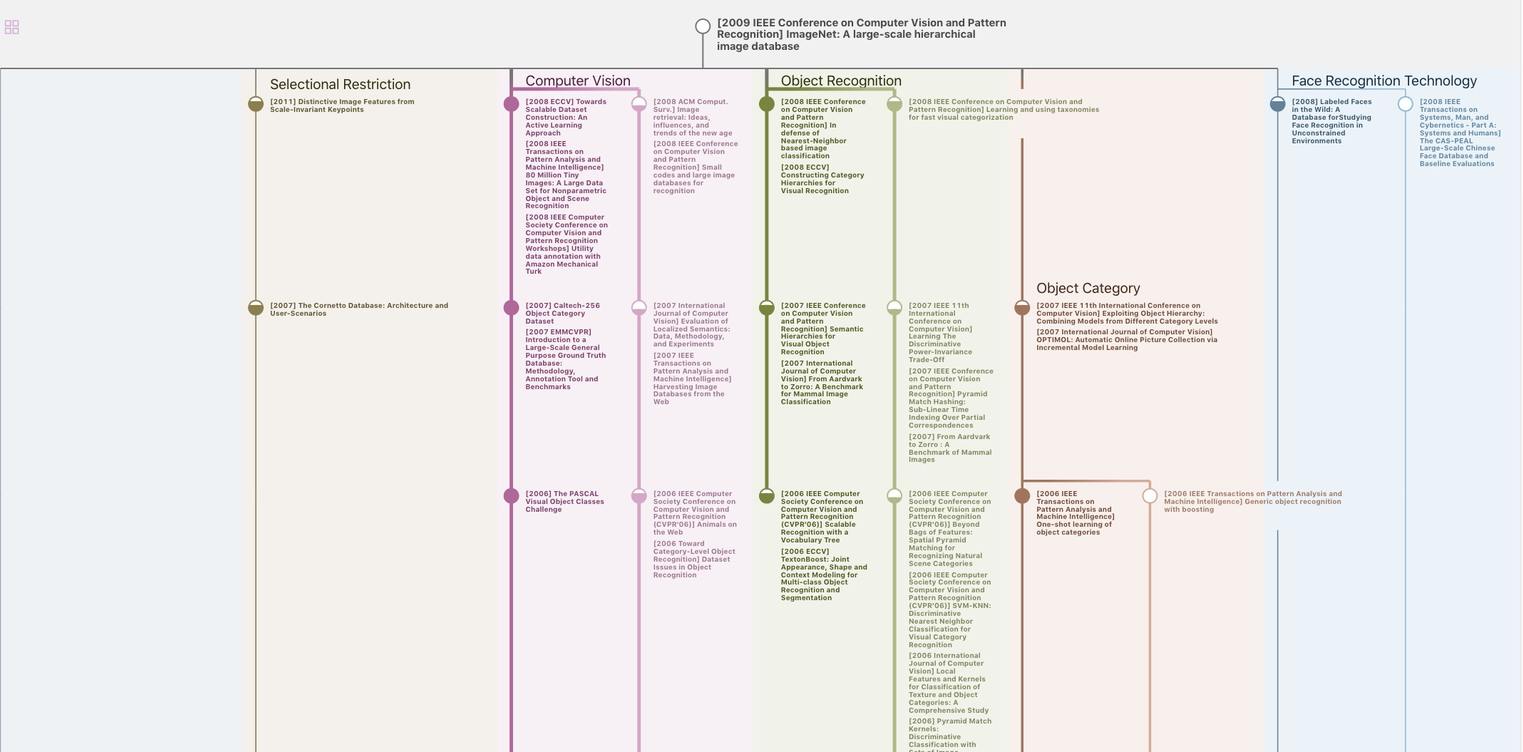
生成溯源树,研究论文发展脉络
Chat Paper
正在生成论文摘要