Quantitative Verification and Strategy Synthesis for BDI Agents.
NFM(2023)
摘要
Belief-Desire-Intention (BDI) agents feature probabilistic outcomes, e.g. the chance an agent tries but fails to open a door, and non-deterministic choices: what plan/intention to execute next? We want to reason about agents under both probabilities and non-determinism to determine, for example, probabilities of mission success and the strategies used to maximise this. We define a Markov Decision Process describing the semantics of the Conceptual Agent Notation (Can) agent language that supports non-deterministic event, plan, and intention selection, as well as probabilistic action outcomes. The model is derived through an encoding to Milner’s Bigraphs and executed using the BigraphER tool. We show, using probabilistic model checkers PRISM and Storm, how to reason about agents including: probabilistic and reward-based properties, strategy synthesis, and multi-objective analysis. This analysis provides verification and optimisation of BDI agent design and implementation.
更多查看译文
关键词
bdi agents,strategy synthesis
AI 理解论文
溯源树
样例
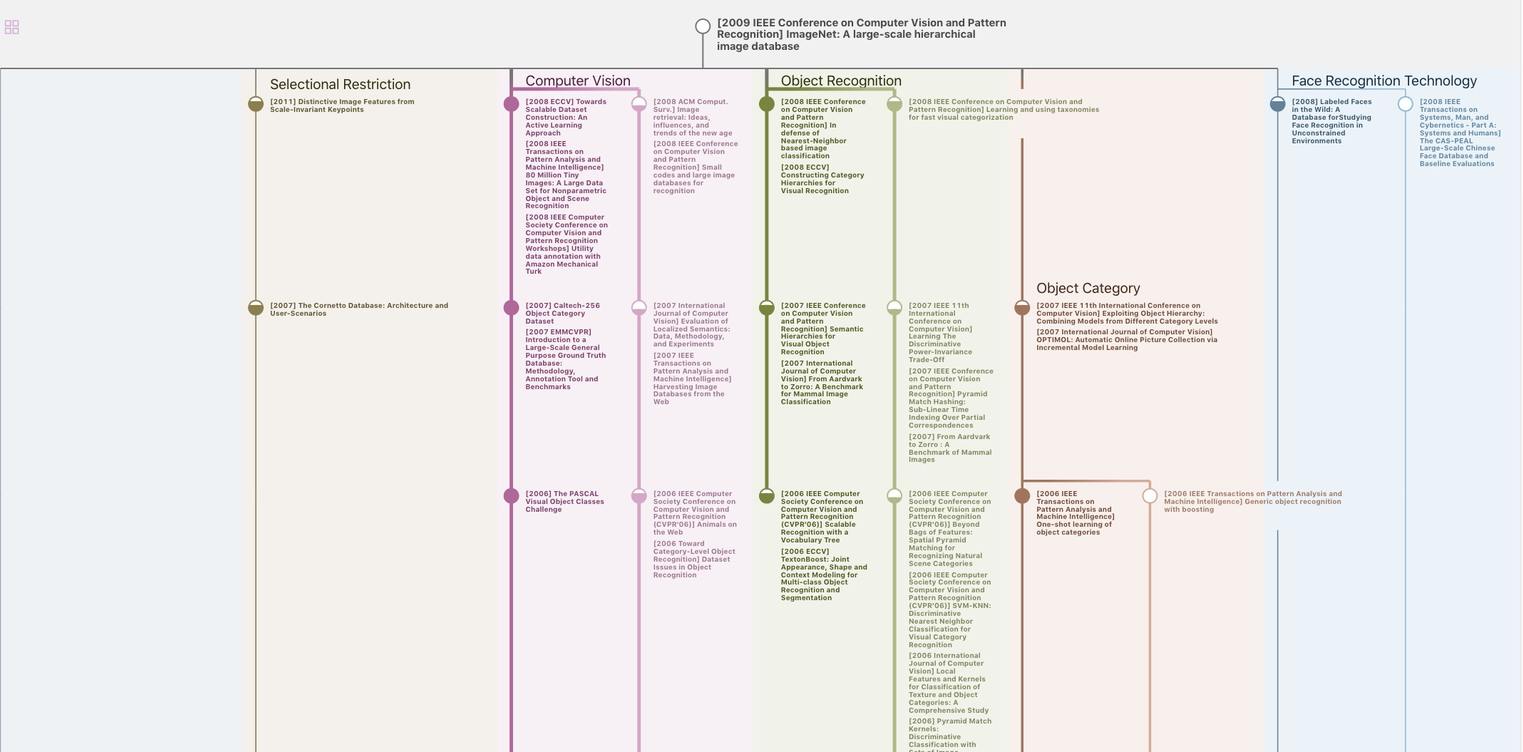
生成溯源树,研究论文发展脉络
Chat Paper
正在生成论文摘要