Multi-dimensional Learner Profiling by Modeling Irregular Multivariate Time Series with Self-supervised Deep Learning.
AIED(2023)
摘要
Personalised or intelligent tutoring systems are being rapidly adopted because they enable tailored learner choices in, for example, exercise materials, study time, and intensity (i.e., the number of chosen exercises) over extended periods of time. This, however, poses significant challenges for profiling the characteristics of learner behaviors, mostly due to the great diversity in each individual’s learning path, the timing of exercise accomplishments, and varying degrees of engagement over time. To address this problem, this paper proposes an innovative approach that uses self-supervised deep learning to consolidate learner behaviors and performance into compact representations via irregular multivariate time series modeling. These representations can be used to highlight learners’ multi-dimensional behavioral characteristics on a massive scale for self-directed learners who can freely pick exercises and study at their own pace. With experiments on a large-scale real-world dataset, we empirically show that our approach can effectively reveal learner individuality as well as commonality in characteristics.
更多查看译文
关键词
irregular multivariate time series,deep learning,multi-dimensional,self-supervised
AI 理解论文
溯源树
样例
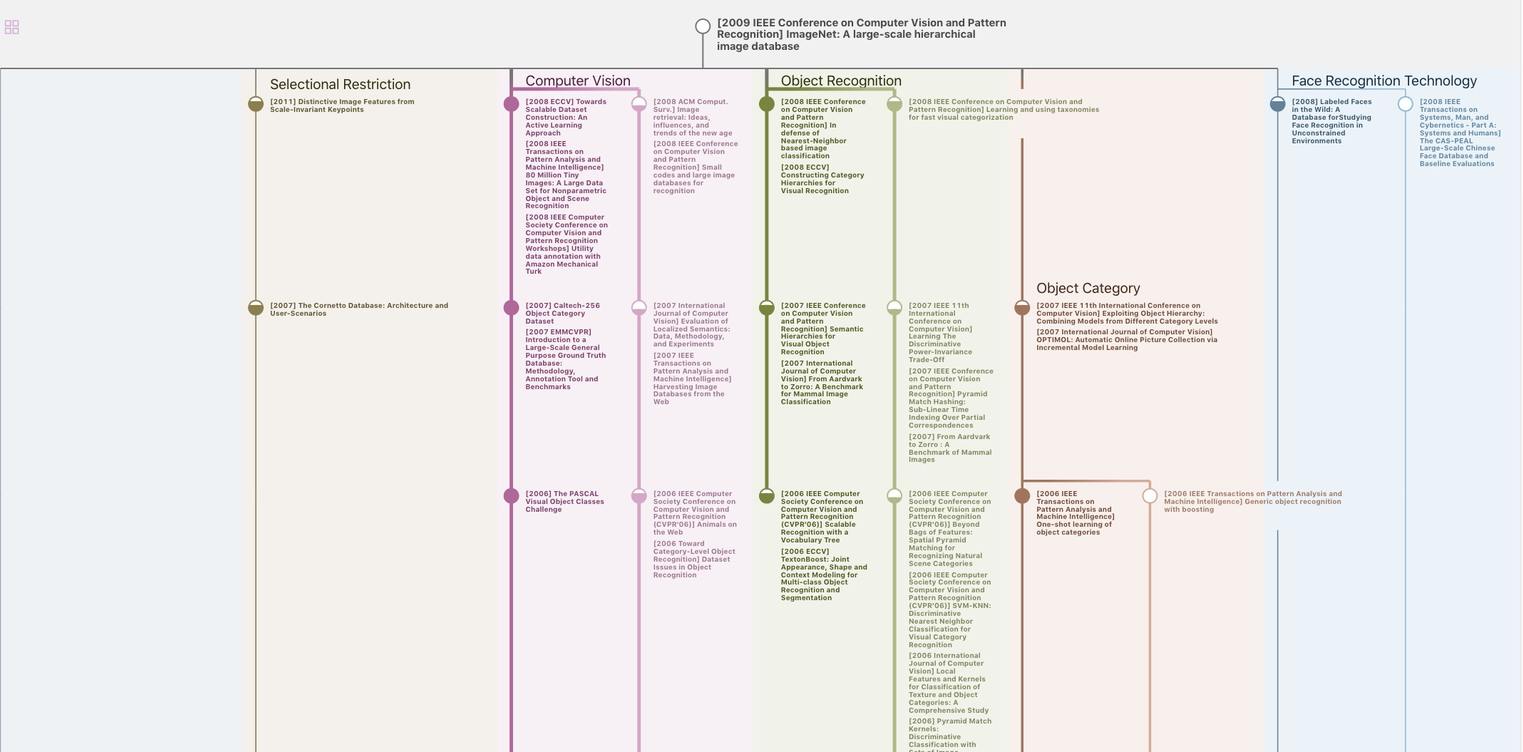
生成溯源树,研究论文发展脉络
Chat Paper
正在生成论文摘要