Predicting Progress in a Large-Scale Online Programming Course.
AIED(2023)
摘要
With vast amounts of educational data being generated in schools, educators are increasingly embracing data mining techniques to track student progress, especially in programming courses, a growing area of computer science education research. However, there are few accurate and interpretable methods to track student progress in programming courses. To bridge this gap, we propose a decision tree approach to predict student progress in a large-scale online programming course. We demonstrate that this approach is highly interpretable and accurate, with an overall average accuracy of 88% and average dropout accuracy of 82%. Additionally, we identify important slides such as problem slide which significantly impact student outcomes.
更多查看译文
关键词
programming,progress,large-scale
AI 理解论文
溯源树
样例
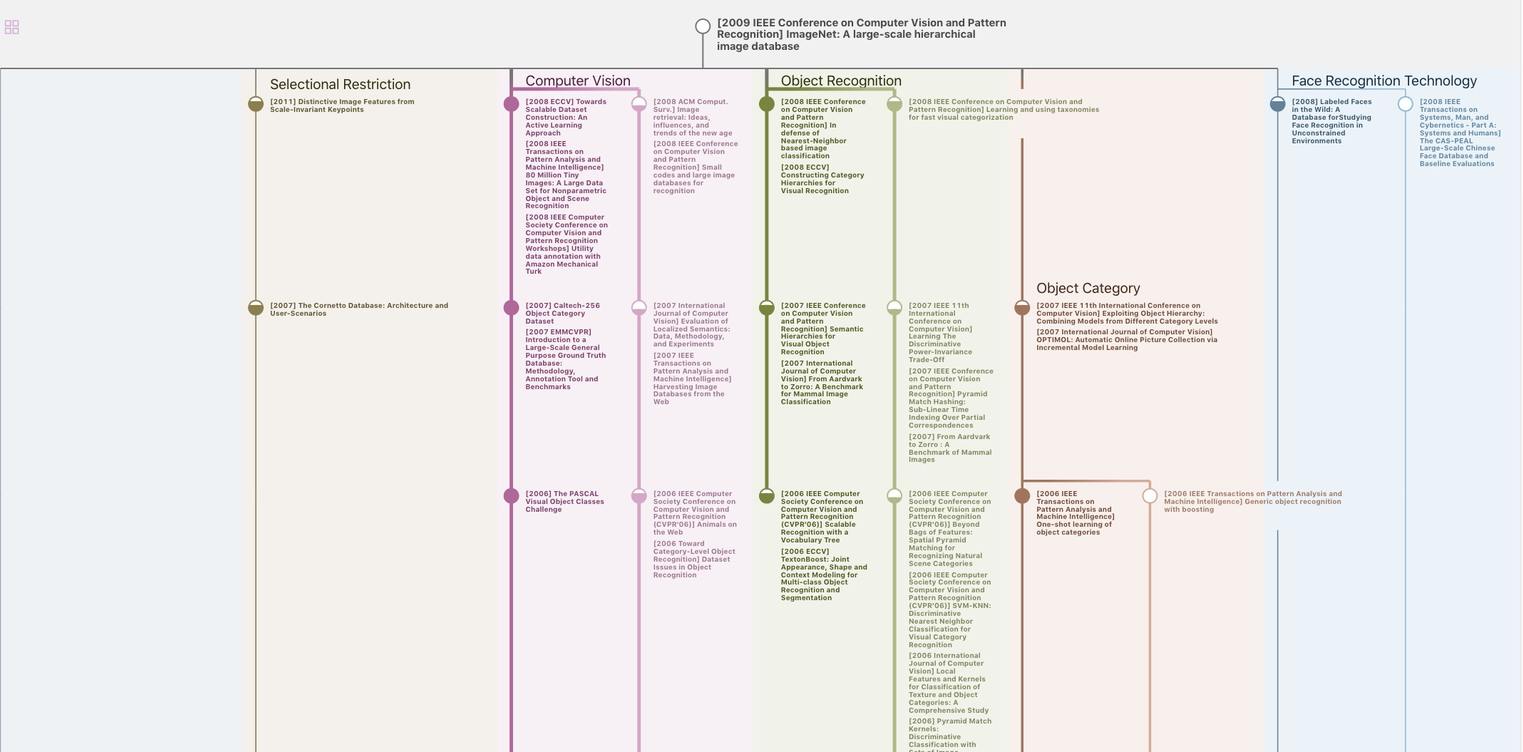
生成溯源树,研究论文发展脉络
Chat Paper
正在生成论文摘要