Unsupervised Embedding of Trajectories Captures the Latent Structure of Mobility
Research Square (Research Square)(2021)
摘要
Abstract Human mobility drives major societal phenomena including epidemics, economies, and innovation. Historically, mobility was constrained by geographic distance, however, in the globalizing world, language, culture, and history are increasingly important. Here, we show a mathematical equivalence between word2vec model and the gravity model of mobility and demonstrate that, by using three human trajectory datasets, word2vec encodes nuanced relationships between locations into a systematic and meaningful vector-space, providing a functional distance between locations, as well as a representation for studying the many dimensions of mobility. Focusing on the case of scientific mobility, we show that embeddings implicitly learn cultural, linguistic, and hierarchical relationships at multiple levels of granularity. Connecting neural embeddings to the gravity model opens up new avenues for the study of mobility.
更多查看译文
关键词
trajectories captures,mobility,latent structure
AI 理解论文
溯源树
样例
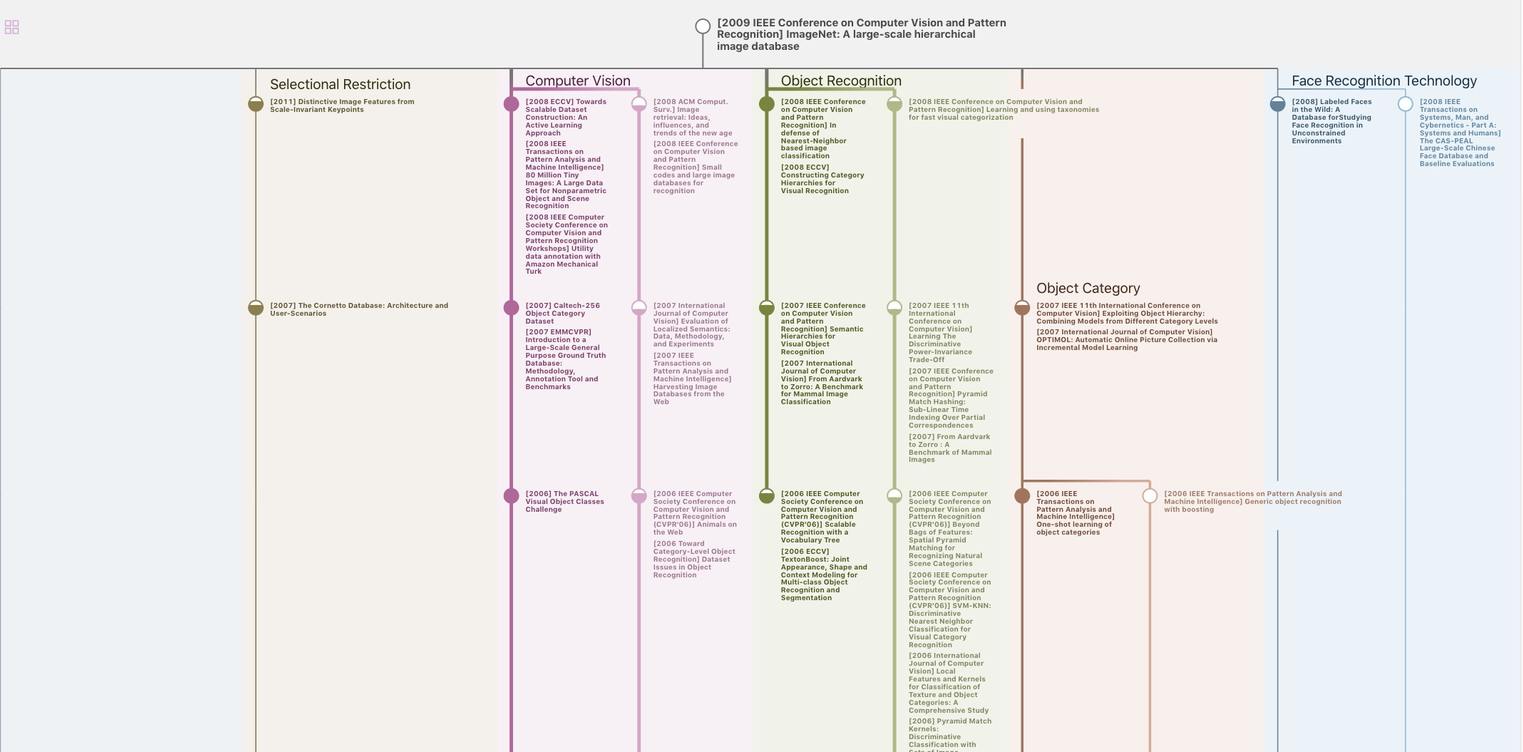
生成溯源树,研究论文发展脉络
Chat Paper
正在生成论文摘要