Readability Classification with Wikipedia Data and All-MiniLM Embeddings
Artificial Intelligence Applications and Innovations. AIAI 2023 IFIP WG 12.5 International Workshops(2023)
摘要
Evaluating the readability of text has been a critical step in several applications, ranging from text simplification, learning new languages, providing school children with appropriate reading material to conveying important medical information in an easily understandable way. A lot of research has been dedicated to evaluating readability on larger bodies of texts, like articles and paragraphs, but the application on single sentences has received less attention. In this paper, we explore several machine learning techniques - logistic regression, random forest, Naive Bayes, KNN, MLP, XGBoost - on a corpus of sentences from the English and simple English Wikipedia. We build and compare a series of binary readability classifiers using extracted features as well as generated all-MiniLM-L6-v2-based embeddings, and evaluate them against standard classification evaluation metrics. To the authors’ knowledge, this is the first time this sentence transformer is used in the task of readability assessment. Overall, we found that the MLP models, with and without embeddings, as well as the Random Forest, outperformed the other machine learning algorithms.
更多查看译文
关键词
Readability classification, Text simplification, Embeddings
AI 理解论文
溯源树
样例
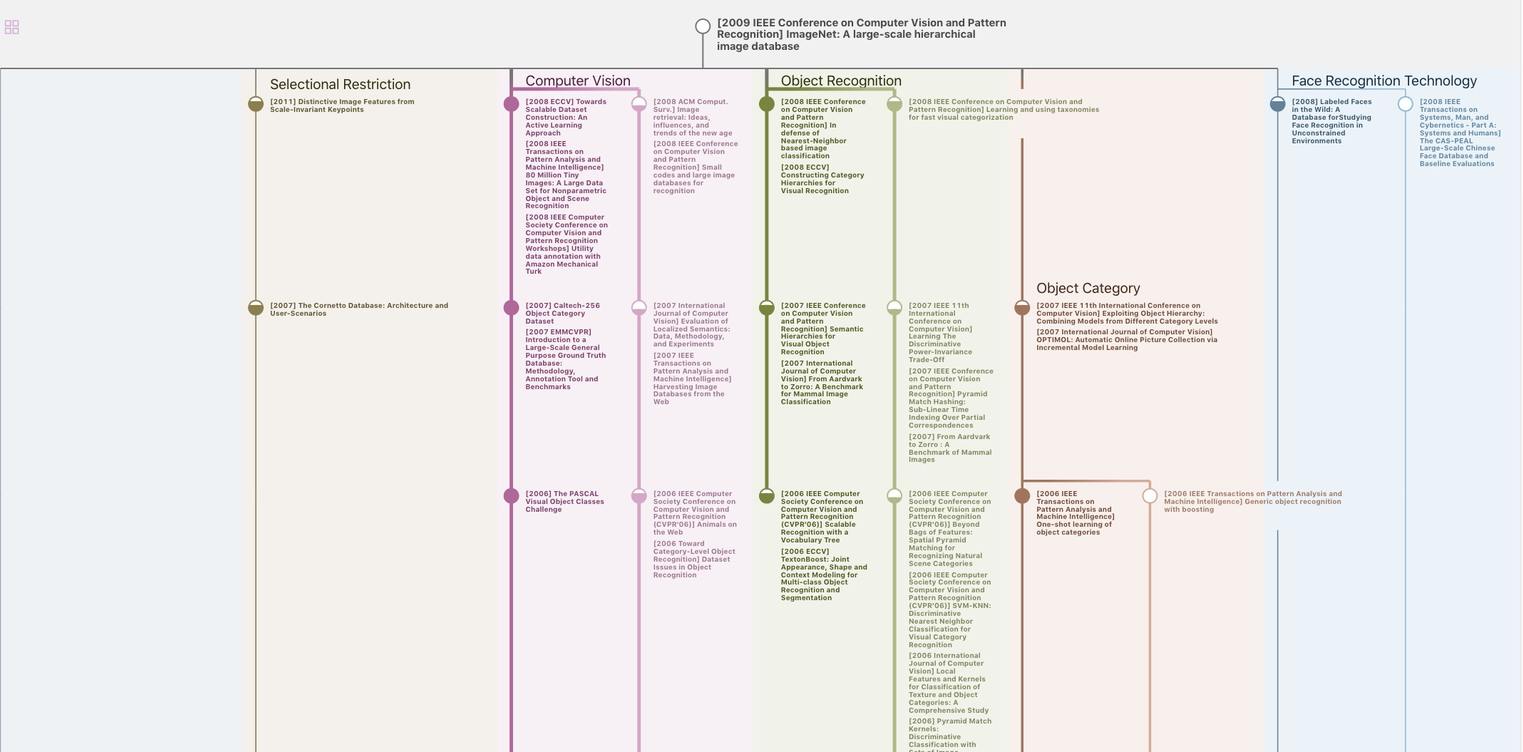
生成溯源树,研究论文发展脉络
Chat Paper
正在生成论文摘要