Portfolio Selection Strategy: A Teaching–Learning-Based Optimization (TLBO) Approach
Proceedings of International Joint Conference on Advances in Computational Intelligence(2023)
摘要
In portfolio selection, increasing the returns and reducing the risks were always among the most significant problems of interest to investors in the stock markets. In recent years, various solutions and recommendations have been proposed concerning the frequency of portfolio optimization problems, with the maximum return at the lowest potential risk. Sharpe ratio is one of the most referenced risk-return measures used in finance. Here, the teaching–learning-based optimization (TLBO) algorithm proposed in 2011 is one of the most efficient population-based approaches that has been considered to address the portfolio optimization problem. According to the framework of William Sharpe, in this research, the author aims to answer the portfolio selection issue by employing the TLBO algorithm to optimize the Sharpe ratio. For this purpose, the data associated with the monthly returns of 3 listed firms in the BSE 500 from June 2018 to May 2019 is gathered. The results of data sets have demonstrated the various processes, namely the teaching and learning phases of the proposed TLBO algorithm, and shown that it is effective and efficient in solving complex portfolio selection problems.
更多查看译文
关键词
optimization,strategy,selection,learning-based
AI 理解论文
溯源树
样例
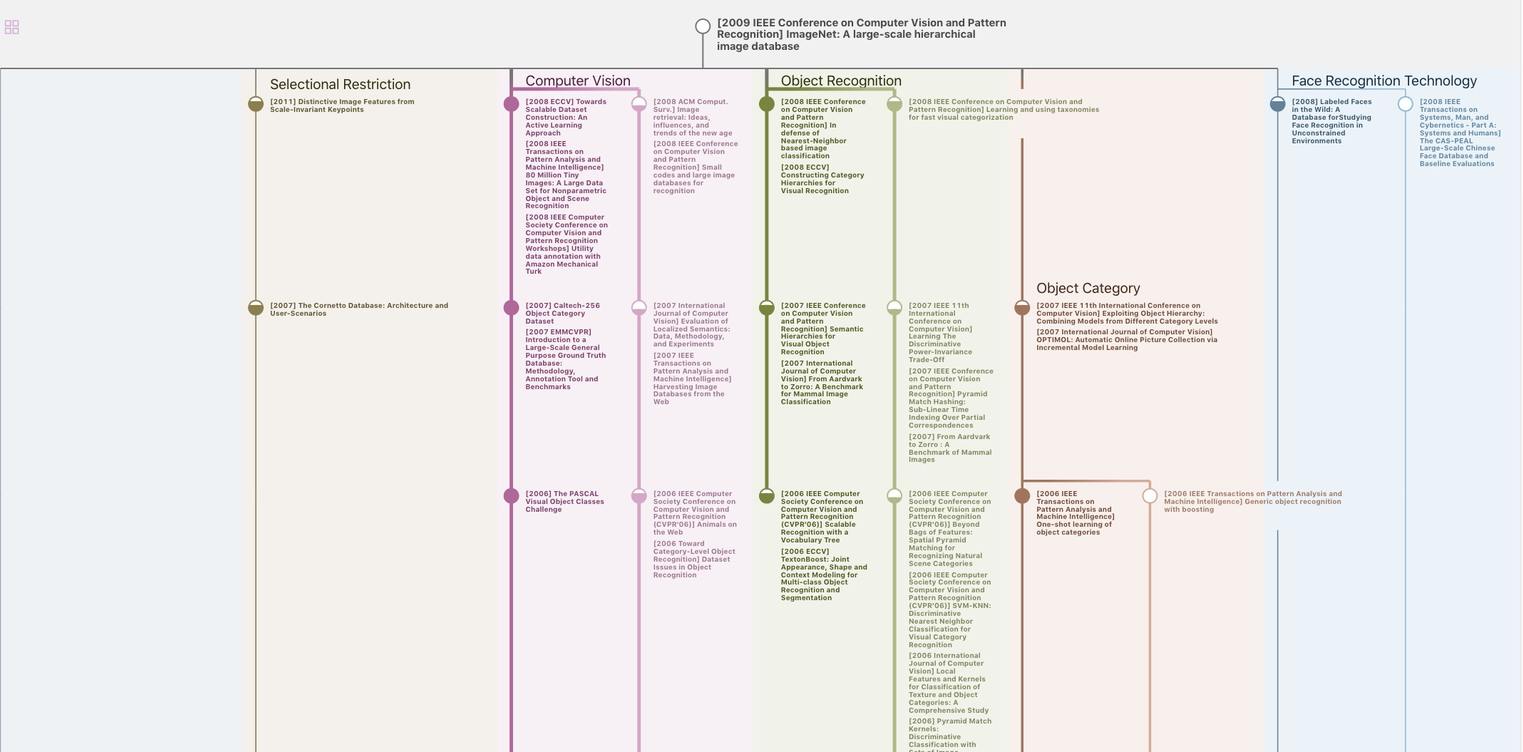
生成溯源树,研究论文发展脉络
Chat Paper
正在生成论文摘要