Targeted Attacks on Time Series Forecasting
Advances in Knowledge Discovery and Data Mining(2023)
摘要
Time Series Forecasting (TSF) is well established in domains dealing with temporal data to predict future events yielding the basis for strategic decision-making. Previous research indicated that forecasting models are vulnerable to adversarial attacks, that is, maliciously crafted perturbations of the original data with the goal of altering the model’s predictions. However, attackers targeting specific outcomes pose a substantially more severe threat as they could manipulate the model and bend it to their needs. Regardless, there is no systematic approach for targeted adversarial learning in the TSF domain yet. In this paper, we introduce targeted attacks on TSF in a systematic manner. We establish a new experimental design standard regarding attack goals and perturbation control for targeted adversarial learning on TSF. For this purpose, we present a novel indirect sparse black-box evasion attack on TSF, n Vita. Additionally, we adapt the popular white-box attacks Fast Gradient Sign Method (FGSM) and Basic Iterative Method (BIM). Our experiments confirm not only that all three methods are effective but also that current state-of-the-art TSF models are indeed susceptible to attacks. These results motivate future research in this area to achieve higher reliability of forecasting models.
更多查看译文
关键词
Adversarial Learning, Time Series, Targeted Attack, Forecasting
AI 理解论文
溯源树
样例
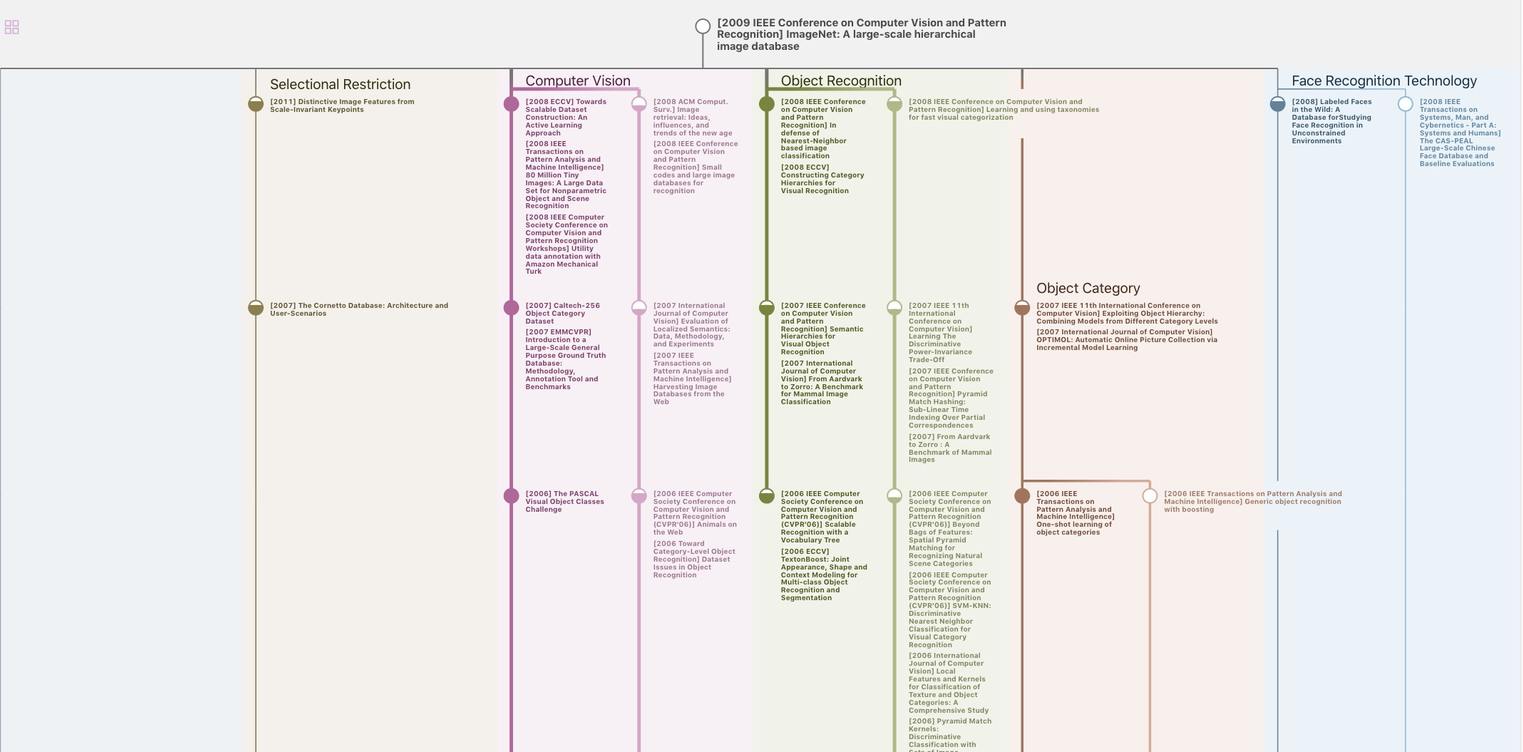
生成溯源树,研究论文发展脉络
Chat Paper
正在生成论文摘要