Comparison of Feature Selection Methods in Regression Modeling: A Simulation Study
Computational Science and Its Applications – ICCSA 2023 Workshops(2023)
摘要
This simulation study explores the impact of different undesirable scenarios (e.g., collinearity, Simpson’s paradox, variable interaction, Freedman’s paradox) on feature selection and coefficients’ estimation using traditional methodologies, such as automatic selection (e.g., stepwise using Akaike information criterion and Bayesian information criterion) and penalized regression (e.g., least absolute shrinkage and selection operator (LASSO), elastic net, relaxed LASSO, adaptive LASSO, minimax concave penalty and smoothly clipped absolute deviation penalty, penalized regression with second-generation p-values). Specifically, we compare wrapper and embedded methods regarding the feature selection, coefficients’ estimation and models’ performance. Our results show that the choice of the methodology can affect the number and the type of selected features, as well as accuracy and precision of coefficients’ estimates. Furthermore, we find that the performance can also depend on the characteristics of the data.
更多查看译文
关键词
feature selection methods,regression modeling
AI 理解论文
溯源树
样例
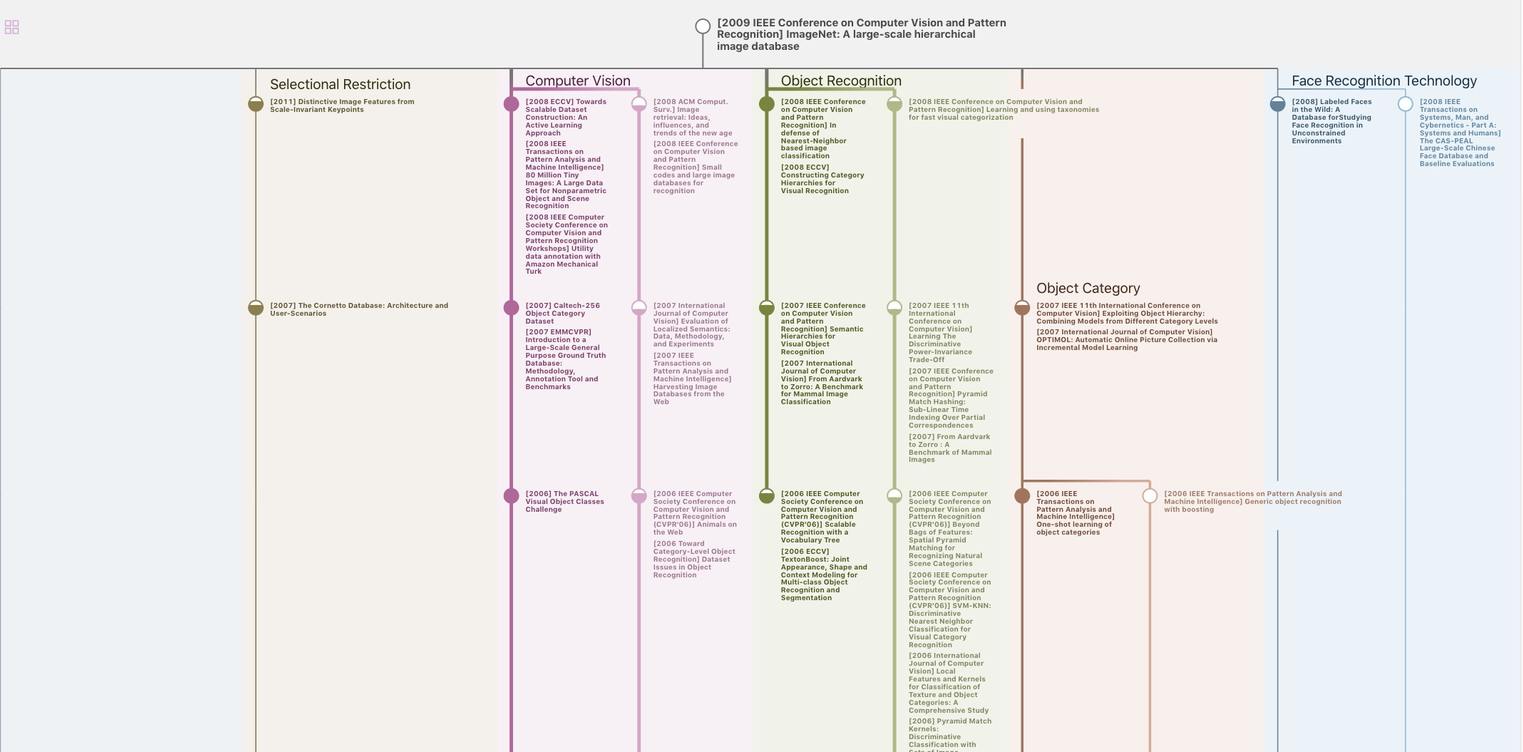
生成溯源树,研究论文发展脉络
Chat Paper
正在生成论文摘要