Exploration of Annotation Strategies for Automatic Short Answer Grading
Artificial Intelligence in Education(2023)
摘要
Automatic Short Answer Grading aims to automatically grade short answers authored by students. Recent work has shown that this task can be effectively reformulated as a Natural Language Inference problem. State-of-the-art is defined by the use of large pretrained language models fine-tuned in the domain dataset. But how to quantify the effectiveness of the models in small data regimes still remains an open issue. In this work we present a set of experiments to analyse the impact of different annotation strategies when not enough training examples for fine-tuning the model are available. We find that when annotating few examples, it is preferable to have more question variability than more answers per question. With this annotation strategy, our model outperforms state-of-the-art systems utilizing only 10% of the full-training set. Finally, experiments show that the use of out-of-domain annotated question-answer examples can be harmful when fine-tuning the models.
更多查看译文
关键词
Automatic Short Answer Grading, Natural Language Processing, Natural Language Inference, Transfer Learning
AI 理解论文
溯源树
样例
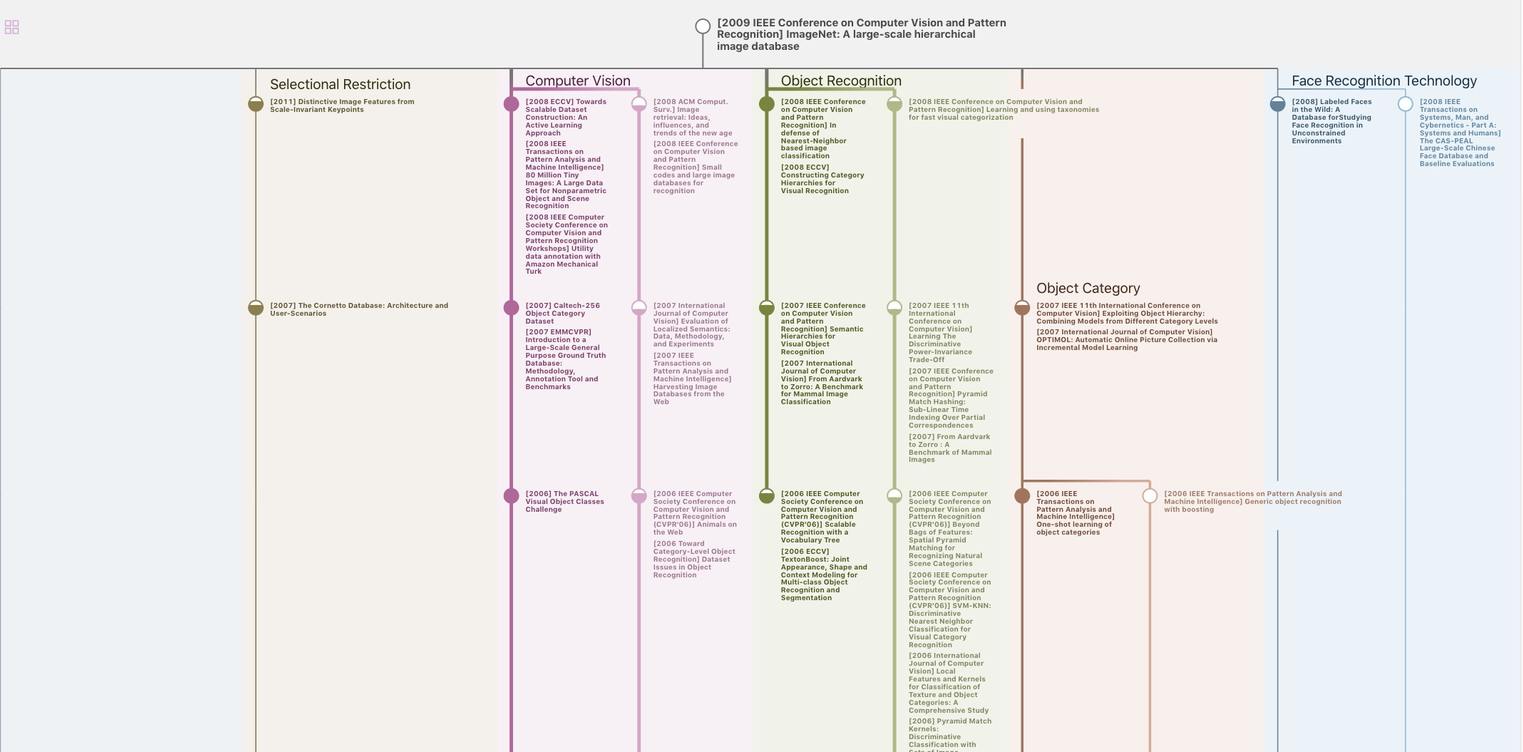
生成溯源树,研究论文发展脉络
Chat Paper
正在生成论文摘要