Phase Correction and Noise-to-Noise Denoising of Diffusion Magnetic Resonance Images Using Neural Networks
Computational Science – ICCS 2023(2023)
摘要
Diffusion magnetic resonance imaging (dMRI) is an important technique used in neuroimaging. It features a relatively low signal-to-noise ratio (SNR) which poses a challenge, especially at stronger diffusion weighting. A common solution to the resulting poor precision is to average signal from multiple identical measurements. Indeed, averaging the magnitude signal is sufficient if the noise is sampled from a distribution with zero mean value. However, at low SNR, the magnitude signal is increased by the rectified noise floor, such that the accuracy can only be maintained if averaging is performed on the complex signal. Averaging of the complex signal is straightforward in the non-diffusion-weighted images, however, in the presence of diffusion encoding gradients, any motion of the tissue will incur a phase shift in the signal which must be corrected prior to averaging. Instead, they are averaged in the modulus image space, which is associated with the effect of Rician bias. Moreover, repeated acquisitions further increase acquisition times which, in turn, exacerbate the challenges of patient motion. In this paper, we propose a method to correct phase variations using a neural network trained on synthetic MR data. Then, we train another network using the Noise2Noise paradigm to denoise real dMRI of the brain. We show that phase correction made Noise2Noise training possible and that the latter improved the denoising quality over averaging modulus domain images.
更多查看译文
关键词
diffusion magnetic resonance images,magnetic resonance images,magnetic resonance,noise-to-noise
AI 理解论文
溯源树
样例
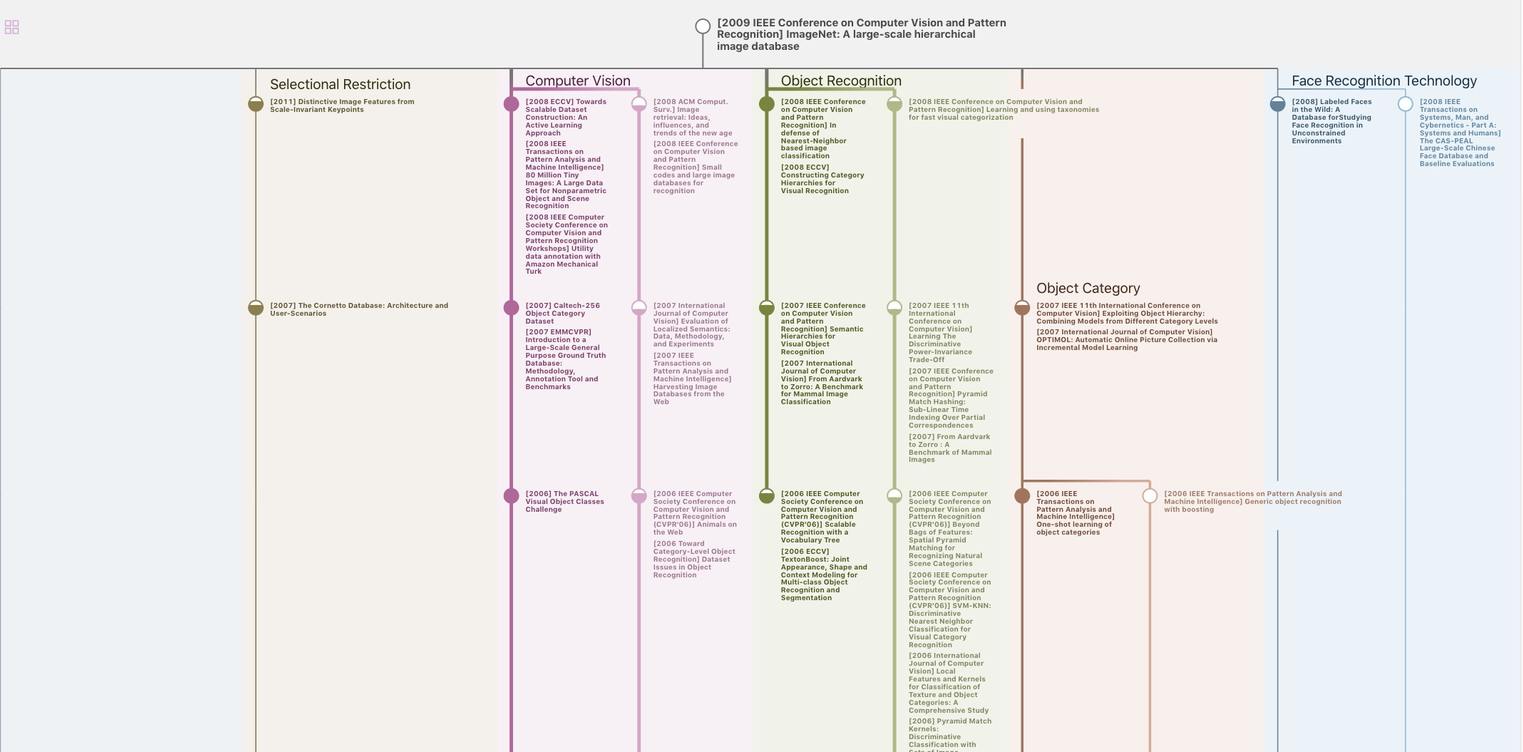
生成溯源树,研究论文发展脉络
Chat Paper
正在生成论文摘要