Implementation of Legal Documents Text Summarization and Classification by Applying Neural Network Techniques
Machine Intelligence Techniques for Data Analysis and Signal Processing(2023)
摘要
Rhetorical role labelling of legal judgements and text summarization of legal documents help in various downstream tasks like computing document similarity, legal documents search, etc. So, it is important to work on these important problems. In these problems of our project, the corpus contains judgements from the Supreme Court of India that are provided by the Artificial Intelligence for Legal Assistance (AILA). These problems are challenging as legal case documents are usually not well-structured and variation of opinions exists between legal experts in rhetorical roles labelling and assigning relevance to legal judgements. Many prior works in these domains used conditional random fields (CRF) over manually hand-crafted features. Our research objective is to analyse the performance of deep learning models, namely BERT, RoBERTa, and XLNet over the CRF method as well as observe the nature of the corpus. We used the BERT-extractive-summarizer to generate a summary from the relevant legal judgements. Our best-performing model for legal judgement classification achieves a macro-averaged F1-score of 0.58 and for legal document summarization achieves an accuracy of 0.64 in finding the relevance of judgements and an average F1-score of 0.62 in summary evaluation’s ROUGE-1 scores. This paper presents various methodologies for rhetorical role labelling multi-class classification and finding relevant sentences using binary classification.
更多查看译文
关键词
Legal case documents, Text classification, Text summarization, BERT, RoBERTa, XLNet, Rhetorical role, BERT-extractive-summarizer
AI 理解论文
溯源树
样例
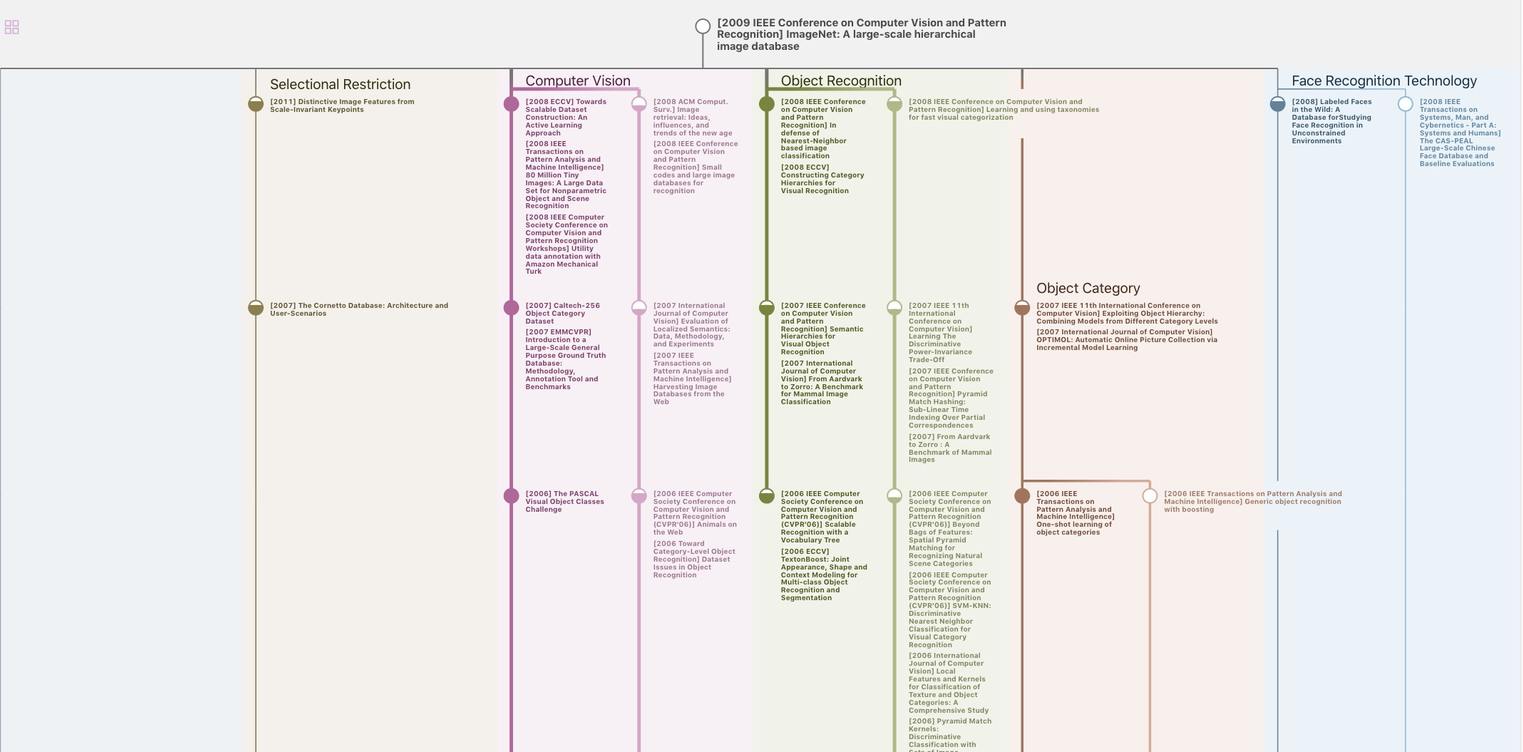
生成溯源树,研究论文发展脉络
Chat Paper
正在生成论文摘要