A Global View-Guided Autoregressive Residual Network for Irregular Time Series Classification
Advances in Knowledge Discovery and Data Mining(2023)
摘要
Irregularly sampled multivariate time series classification tasks become prevalent due to widespread application of sensors. However, different collection frequencies or sensor failures presents nontrivial challenges since mainstream methods generally assume aligned measurements across sensors (variables). Besides, most existing studies fail to account for the relationship between misaligned patterns and classification tasks. To this end, we propose a Global view-guided Autoregressive Residual Network (GARNet), which mainly adopts a generation-and-sampling strategy to deal with the partially observed data at each timestamp. Specifically, we first leverage a Structure-augmented Global Information Extractor (SGIE) to capture the global semantic information in the whole conditioning window. Then, a Global view-guided Autoregressive Recurrent Neural Network (GARNN) is developed to capture the local temporal dynamics hidden in latent factors. Finally, a Masked Temporal Information Aggregator (MTIA) is proposed to attentively aggregate the extracted latent factors at each timestamp for the classification task. Experimental results on two real-world datasets show that GARNet outperforms state-of-the-art methods.
更多查看译文
关键词
Irregularly Sampled Multivariate Time Series, Graph Neural Network, Autoregressive Residual Network
AI 理解论文
溯源树
样例
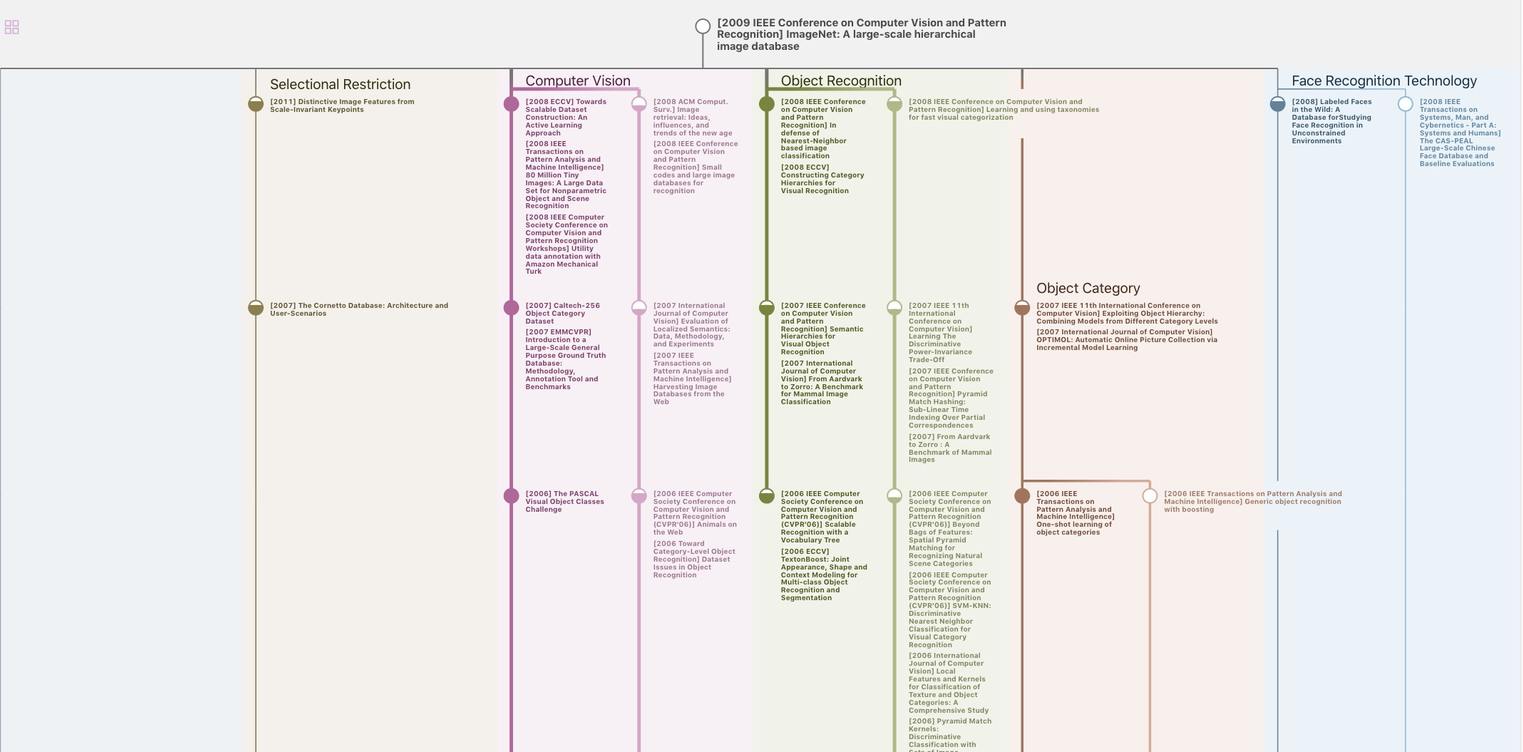
生成溯源树,研究论文发展脉络
Chat Paper
正在生成论文摘要