Improving the Identification of Abusive Language Through Careful Design of Pre-training Tasks
Pattern Recognition(2023)
摘要
The use of Deep Learning-based solutions has become popular in Natural Language Processing due to their remarkable performance in a wide variety of tasks. Specifically, Transformer-based models (e.g. BERT) have become popular in recent years due to their outstanding performance and their ease of adaptation (fine-tuning) in a large number of domains. Despite their outstanding results, the fine-tuning of these models under the presence of informal language writing, especially the one that contains offensive words and expressions, remains a challenging task, due to the lack of vocabulary coverage and proper task contextual information. To overcome this issue, we proposed the domain adaptation of the BERT language model to the abusive language detection task. In order to achieve this, we constrain the language model with the adaptation of two default pre-trained tasks, through the retraining of the model parameters. The obtained configurations were evaluated in six abusive language datasets, showing encouraging results; a remarkable improvement was achieved with the use of the proposed approaches in comparison with its base model. In addition to this, competitive results were obtained with respect to state-of-the-art approaches, thus obtaining a robust and easy-to-train model for the identification of abusive language.
更多查看译文
关键词
language,pre-training
AI 理解论文
溯源树
样例
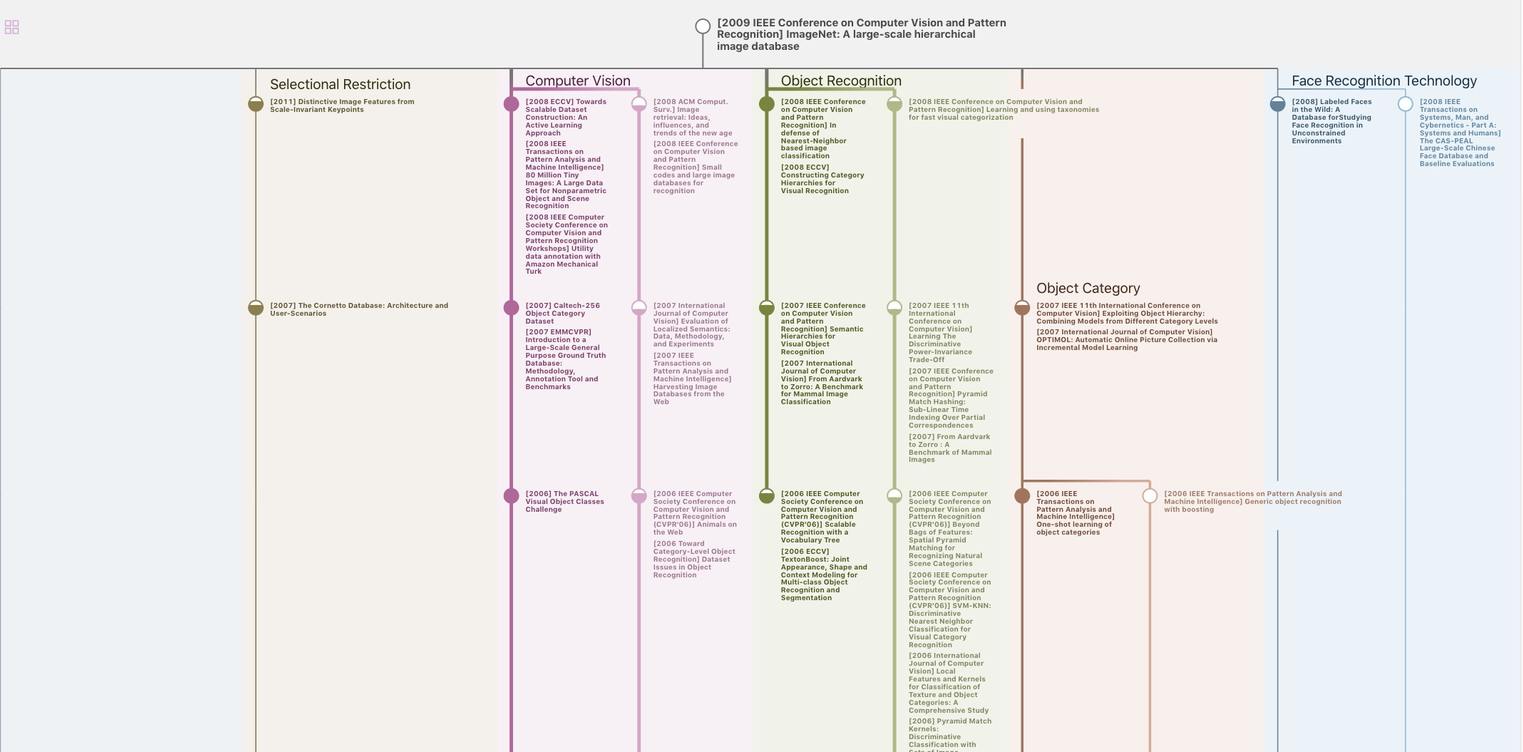
生成溯源树,研究论文发展脉络
Chat Paper
正在生成论文摘要