Exploration and Exploitation of Unlabeled Data for Open-Set Semi-Supervised Learning
CoRR(2023)
摘要
In this paper, we address a complex but practical scenario in semi-supervised learning (SSL) named open-set SSL, where unlabeled data contain both in-distribution (ID) and out-of-distribution (OOD) samples. Unlike previous methods that only consider ID samples to be useful and aim to filter out OOD ones completely during training, we argue that the exploration and exploitation of both ID and OOD samples can benefit SSL. To support our claim, i) we propose a prototype-based clustering and identification algorithm that explores the inherent similarity and difference among samples at feature level and effectively cluster them around several predefined ID and OOD prototypes, thereby enhancing feature learning and facilitating ID/OOD identification; ii) we propose an importance-based sampling method that exploits the difference in importance of each ID and OOD sample to SSL, thereby reducing the sampling bias and improving the training. Our proposed method achieves state-of-the-art in several challenging benchmarks, and improves upon existing SSL methods even when ID samples are totally absent in unlabeled data.
更多查看译文
关键词
unlabeled data,learning,open-set,semi-supervised
AI 理解论文
溯源树
样例
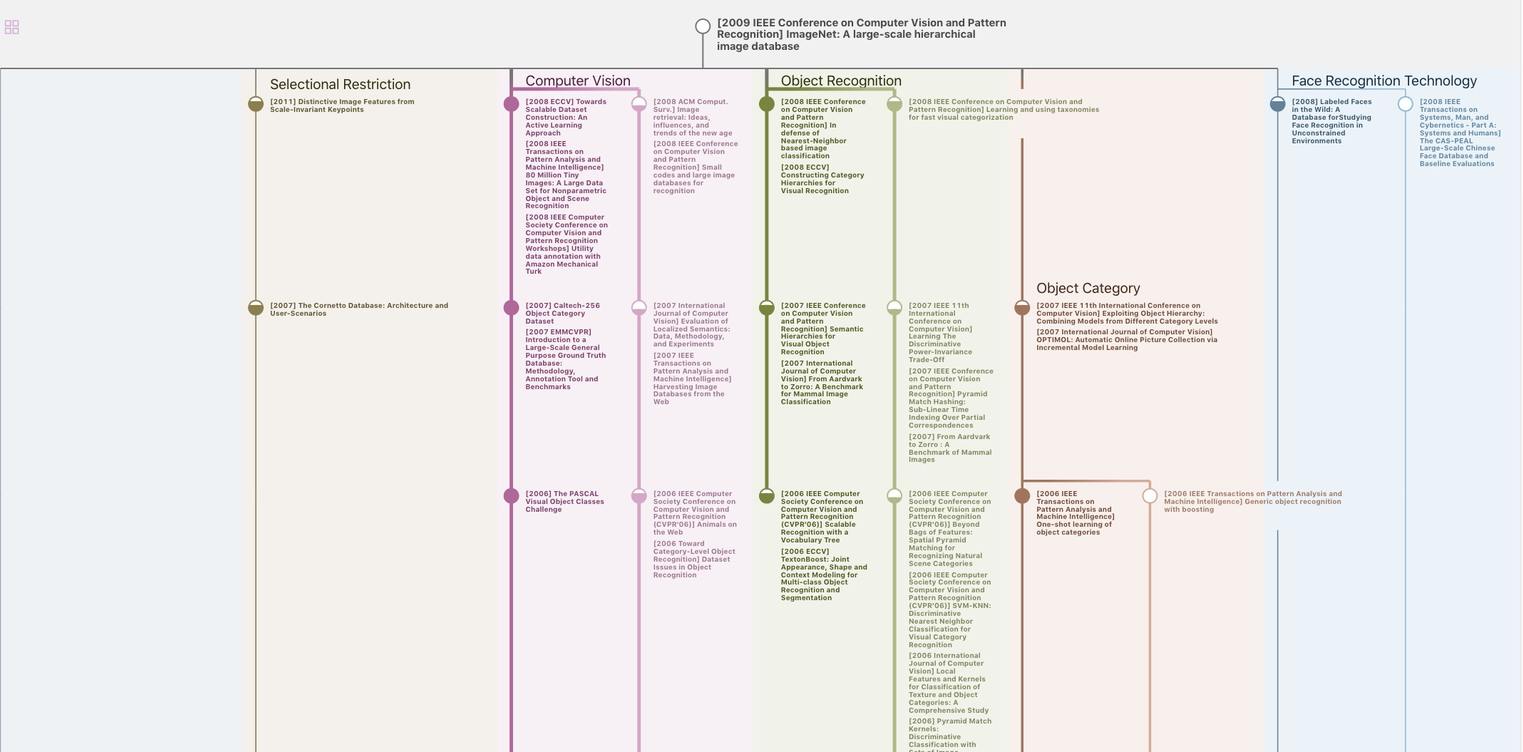
生成溯源树,研究论文发展脉络
Chat Paper
正在生成论文摘要