Geometric Autoencoders -- What You See is What You Decode
ICML 2023(2023)
摘要
Visualization is a crucial step in exploratory data analysis. One possible approach is to train an autoencoder with low-dimensional latent space. Large network depth and width can help unfolding the data. However, such expressive networks can achieve low reconstruction error even when the latent representation is distorted. To avoid such misleading visualizations, we propose first a differential geometric perspective on the decoder, leading to insightful diagnostics for an embedding's distortion, and second a new regularizer mitigating such distortion. Our ``Geometric Autoencoder'' avoids stretching the embedding spuriously, so that the visualization captures the data structure more faithfully. It also flags areas where little distortion could not be achieved, thus guarding against misinterpretation.
更多查看译文
关键词
decode
AI 理解论文
溯源树
样例
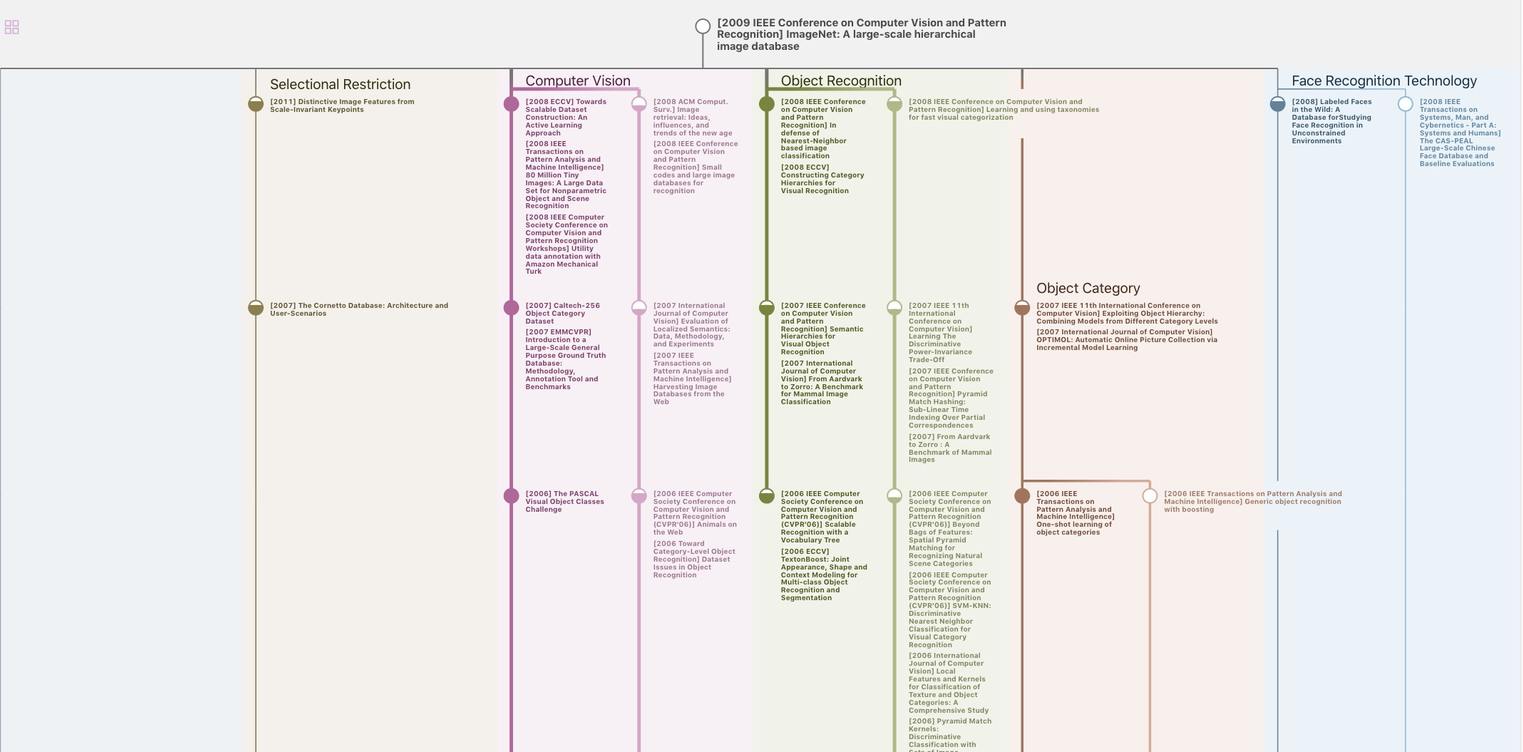
生成溯源树,研究论文发展脉络
Chat Paper
正在生成论文摘要