Mass and tidal parameter extraction from gravitational waves of binary neutron stars mergers using deep learning
JOURNAL OF COSMOLOGY AND ASTROPARTICLE PHYSICS(2024)
摘要
Gravitational Waves (GWs) from coalescing binaries carry crucial information about their component sources, like mass, spin and tidal effects. This implies that the analysis of GW signals from binary neutron star mergers can offer unique opportunities to extract information about the tidal properties of NSs, thereby adding constraints to the NS equation of state. In this work, we use Deep Learning (DL) techniques to overcome the computational challenges confronted in conventional methods of matched-filtering and Bayesian analyses for signal-detection and parameter-estimation. We devise a DL approach to classify GW signals from binary black hole and binary neutron star mergers. We further employ DL to analyze simulated GWs from binary neutron star merger events for parameter estimation, in particular, the regression of mass and tidal deformability of the component objects. The results presented in this work demonstrate the promising potential of DL techniques in GW analysis, paving the way for further advancement in this rapidly evolving field. The proposed approach is an efficient alternative to explore the wealth of information contained within GW signals of binary neutron star mergers, which can further help constrain the NS EoS.
更多查看译文
关键词
gravitational waves,sources,Machine learning,neutron stars
AI 理解论文
溯源树
样例
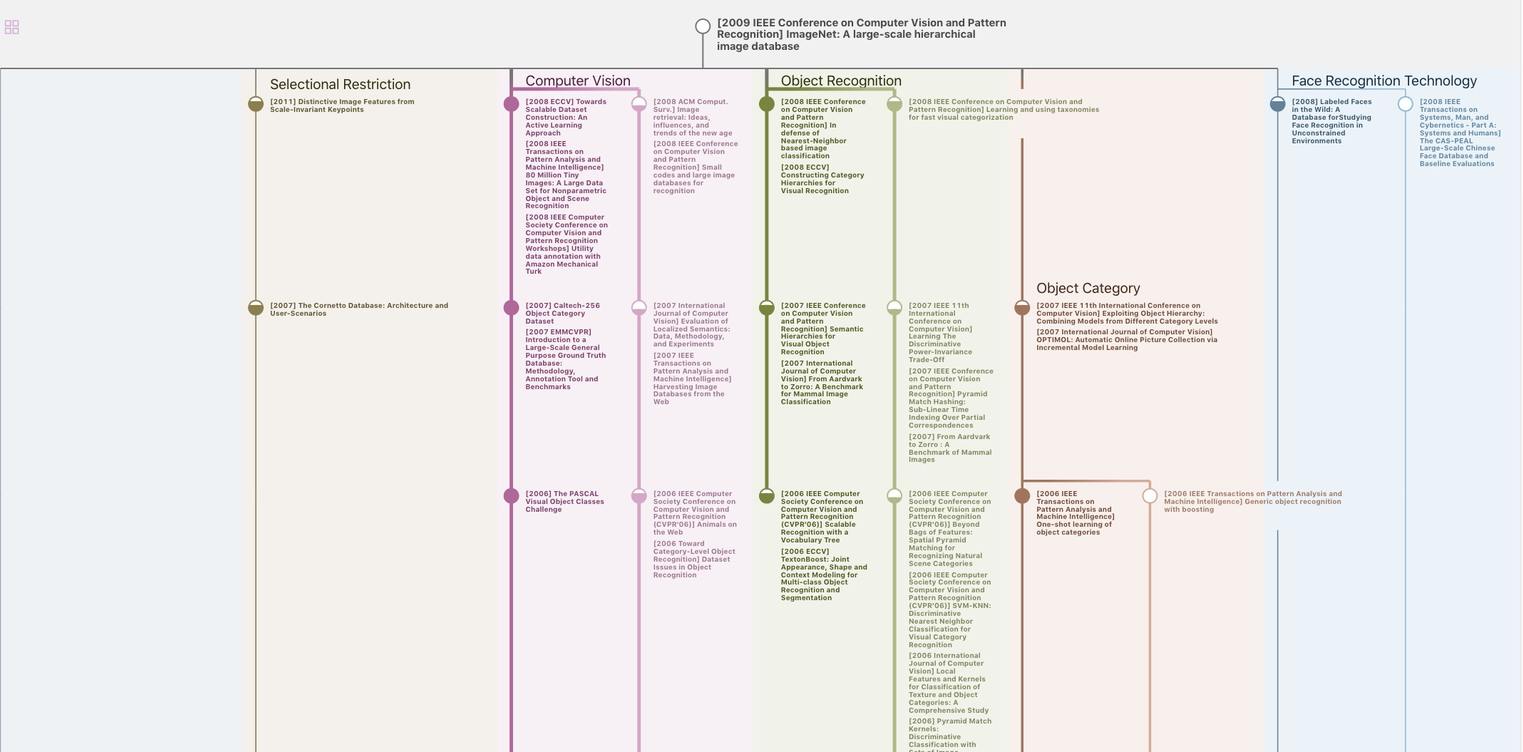
生成溯源树,研究论文发展脉络
Chat Paper
正在生成论文摘要