Non-stationary Bayesian spatial model for disease mapping based on sub-regions
STATISTICAL METHODS IN MEDICAL RESEARCH(2024)
摘要
This paper aims to extend the Besag model, a widely used Bayesian spatial model in disease mapping, to a non-stationary spatial model for irregular lattice-type data. The goal is to improve the model's ability to capture complex spatial dependence patterns and increase interpretability. The proposed model uses multiple precision parameters, accounting for different intensities of spatial dependence in different sub-regions. We derive a joint penalized complexity prior to the flexible local precision parameters to prevent overfitting and ensure contraction to the stationary model at a user-defined rate. The proposed methodology can be used as a basis for the development of various other non-stationary effects over other domains such as time. An accompanying R package fbesag equips the reader with the necessary tools for immediate use and application. We illustrate the novelty of the proposal by modeling the risk of dengue in Brazil, where the stationary spatial assumption fails and interesting risk profiles are estimated when accounting for spatial non-stationary. Additionally, we model different causes of death in Brazil, where we use the new model to investigate the spatial stationarity of these causes.
更多查看译文
关键词
Non-stationary,spatial model,disease mapping,besag model,Integrated Nested Laplace Approximations,penalizing complexity prior
AI 理解论文
溯源树
样例
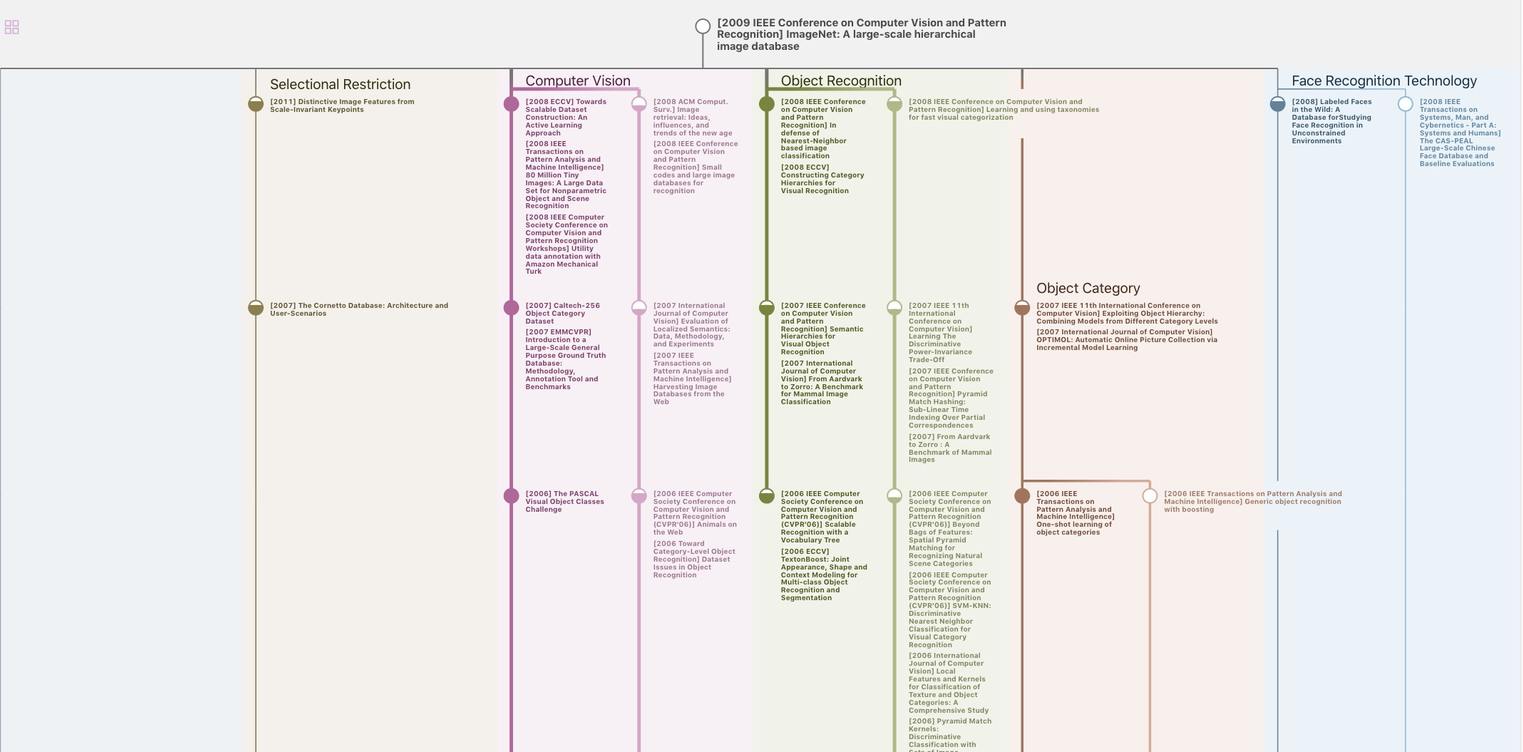
生成溯源树,研究论文发展脉络
Chat Paper
正在生成论文摘要