Diff-Foley: Synchronized Video-to-Audio Synthesis with Latent Diffusion Models
NeurIPS(2023)
摘要
The Video-to-Audio (V2A) model has recently gained attention for its practical application in generating audio directly from silent videos, particularly in video/film production. However, previous methods in V2A have limited generation quality in terms of temporal synchronization and audio-visual relevance. We present Diff-Foley, a synchronized Video-to-Audio synthesis method with a latent diffusion model (LDM) that generates high-quality audio with improved synchronization and audio-visual relevance. We adopt contrastive audio-visual pretraining (CAVP) to learn more temporally and semantically aligned features, then train an LDM with CAVP-aligned visual features on spectrogram latent space. The CAVP-aligned features enable LDM to capture the subtler audio-visual correlation via a cross-attention module. We further significantly improve sample quality with `double guidance'. Diff-Foley achieves state-of-the-art V2A performance on current large scale V2A dataset. Furthermore, we demonstrate Diff-Foley practical applicability and generalization capabilities via downstream finetuning. Project Page: see https://diff-foley.github.io/
更多查看译文
关键词
latent diffusion,synthesis,diff-foley,video-to-audio
AI 理解论文
溯源树
样例
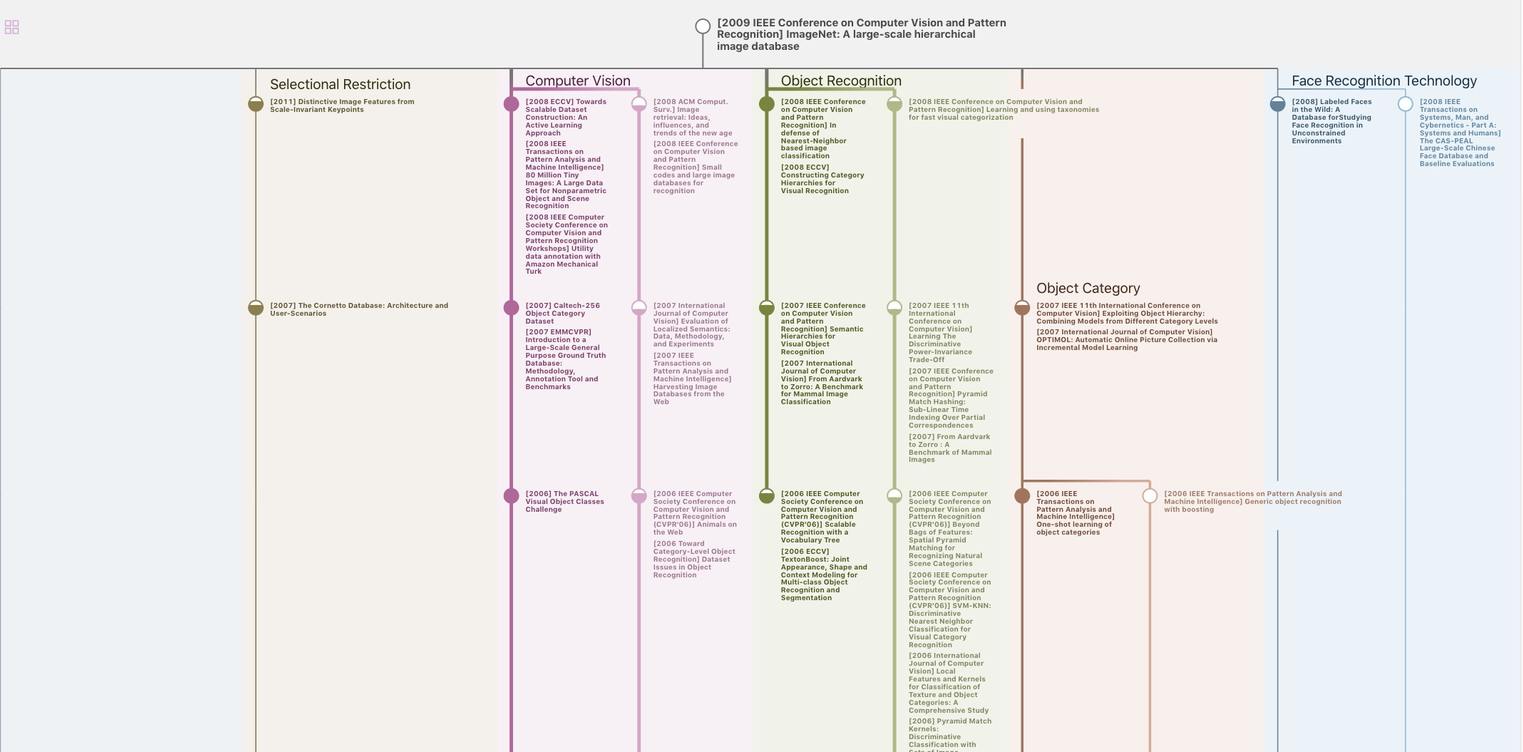
生成溯源树,研究论文发展脉络
Chat Paper
正在生成论文摘要