Detecting COVID-19 in chest CT images based on several pre-trained models
Multimedia Tools and Applications(2024)
摘要
This paper explores the use of chest CT scans for early detection of COVID-19 and improved patient outcomes. The proposed method employs advanced techniques, including binary cross-entropy, transfer learning, and deep convolutional neural networks, to achieve accurate results. The COVIDx dataset, which contains 104,009 chest CT images from 1,489 patients, is used for a comprehensive analysis of the virus. A sample of 13,413 images from this dataset is categorised into two groups: 7,395 CT scans of individuals with confirmed COVID-19 and 6,018 images of normal cases. The study presents pre-trained transfer learning models such as ResNet (50), VGG (19), VGG (16), and Inception V3 to enhance the DCNN for classifying the input CT images. The binary cross-entropy metric is used to compare COVID-19 cases with normal cases based on predicted probabilities for each class. Stochastic Gradient Descent and Adam optimizers are employed to address overfitting issues. The study shows that the proposed pre-trained transfer learning models achieve accuracies of 99.07%, 98.70%, 98.55%, and 96.23%, respectively, in the validation set using the Adam optimizer. Therefore, the proposed work demonstrates the effectiveness of pre-trained transfer learning models in enhancing the accuracy of DCNNs for image classification. Furthermore, this paper provides valuable insights for the development of more accurate and efficient diagnostic tools for COVID-19.
更多查看译文
关键词
CT scan images,Deep Learning,COVID-19,Pre-Trained models,Binary Cross Entropy,SGD, Adam
AI 理解论文
溯源树
样例
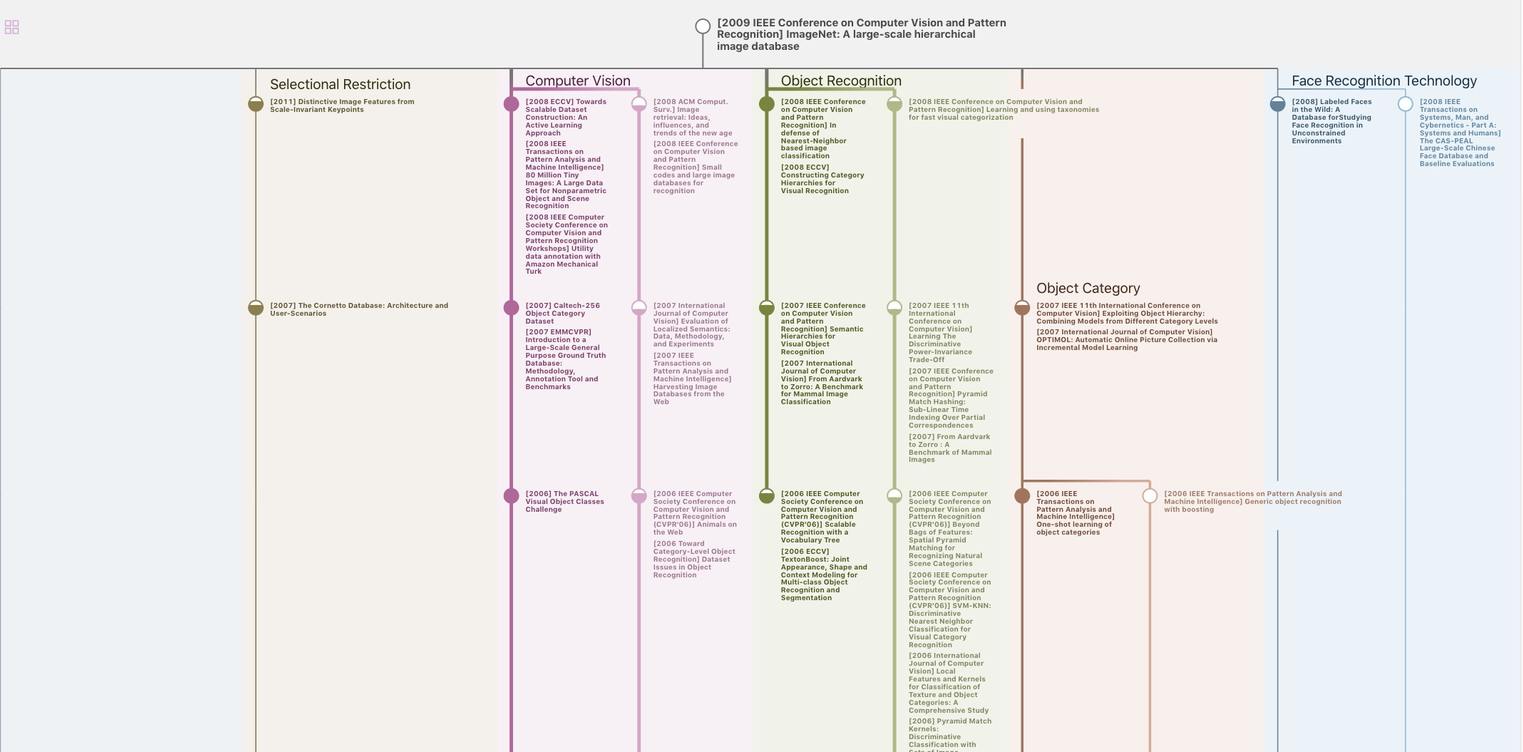
生成溯源树,研究论文发展脉络
Chat Paper
正在生成论文摘要