Comparison of Machine Learning Methods and Conventional Regression for Predicting Renal Function Recovery and Recovery Time among AKI Patients in ICU
Research Square (Research Square)(2022)
摘要
Abstract Background: Acute kidney injury (AKI) is one of the most frequent complications of critical illness. Early predicted renal function recovery can improve AKI prognosis with appropriate interventions. We aimed to derivate and validate two prediction models with high prediction performance and clinical interpretability for renal function recovery and recovery time after AKI, respectively.Methods: We reviewed patients diagnosed with acute kidney disease in intensive care (MIMIC-III) database between 2001 and 2012. The conventional logistic regression and machine algorithm were used to develop prediction models. The performance measures included the area under the receiver operating characteristic curve (AU-ROC), Precision-Recall (PR) curves, and the classification matrix.Results: A total of 3,989 critically-ill AKI adult patients corresponding with Kidney Disease Improving Global Outcomes (KDIGO) with the length of stay in ICU more than 48 hours were included in our analysis cohort. Comparing the model performance, the gradient boosting machine model for predicting renal function recovery had a higher AU-ROC (0.92 [95%CI 0.89-0.94]) than the logistic regression model (0.88 [95%CI 0.84-0.92]). The ExtraTrees machine learning-based model (R2=0.2367) was developed to predict recovery time after AKI, which yielded a better result than the linear regression (R2=0.2006). Conclusions: In this cohort study, machine learning algorithms showed superior ability to predict the prognosis of AKI patients in ICU compared with the traditional logistic and linear regression models. These models may prove clinically helpful and assist clinicians in providing intervention in time, potentially leading to improved prognosis.
更多查看译文
关键词
renal function recovery,machine learning methods,renal function,aki patients,machine learning
AI 理解论文
溯源树
样例
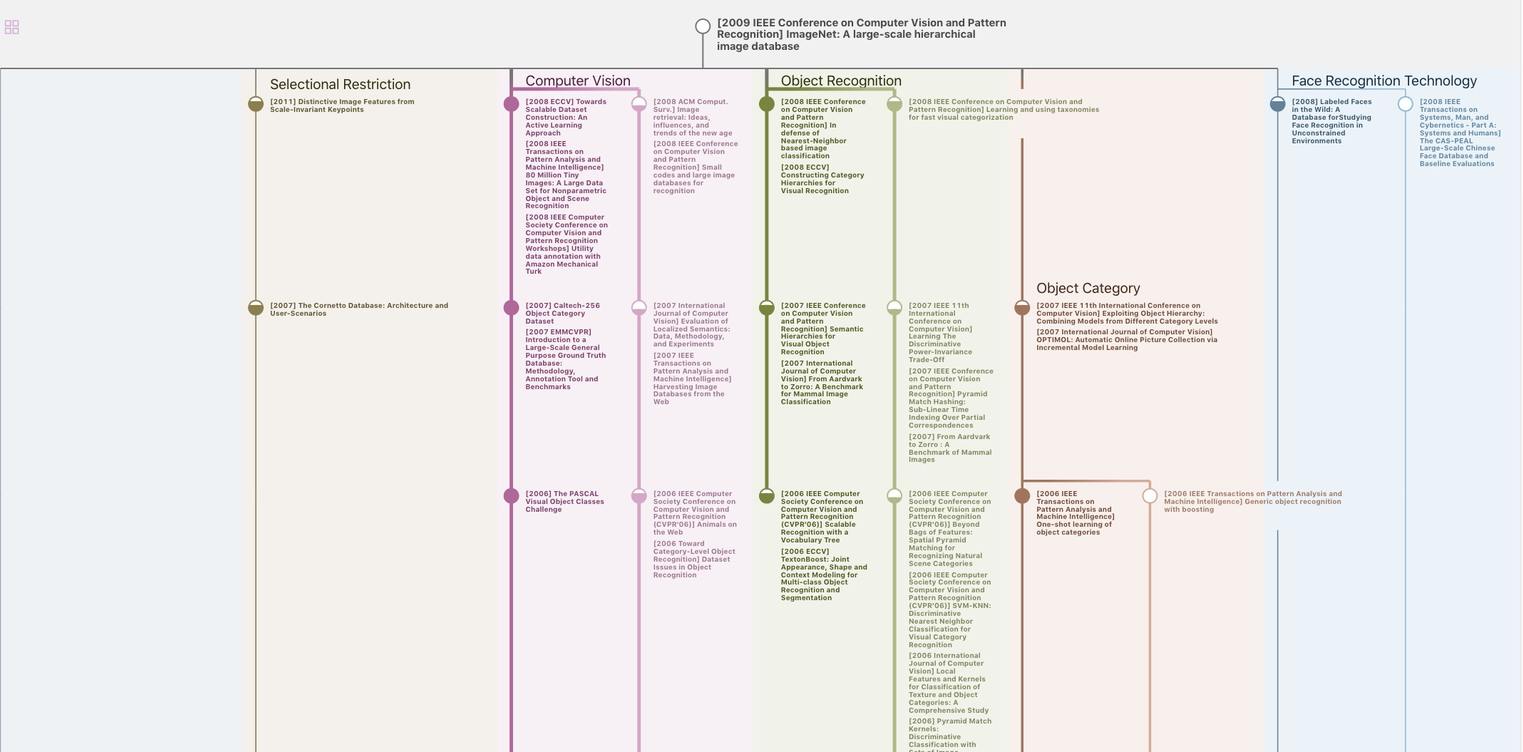
生成溯源树,研究论文发展脉络
Chat Paper
正在生成论文摘要