Predictive Modeling of Galvanotaxis Dynamics at the Single-cell Level with Machine Learning
crossref(2022)
摘要
Abstract Many cell types migrate in response to naturally-generated electric fields. Furthermore, it has been suggested that the external application of an electric field may be used to intervene in and optimize natural processes such as wound healing. Precise cell guidance suitable for such optimization may rely on predictive models of cell migration, which do not generalize. Here, we present a deep learning model that can forecast directedness of cells given a timeseries of previous directedness and electric field values. This model can be used to perform in silico studies by simulating cell migration lines under time-varying and unseen electric fields. Additionally, we show that our modeling approach can be used for a variety of cell types and experimental conditions with very limited training data using transfer learning methods. This predictive approach provides accurate models of cell migration which are suitable for use in control mechanisms with applications in precision medicine.
更多查看译文
AI 理解论文
溯源树
样例
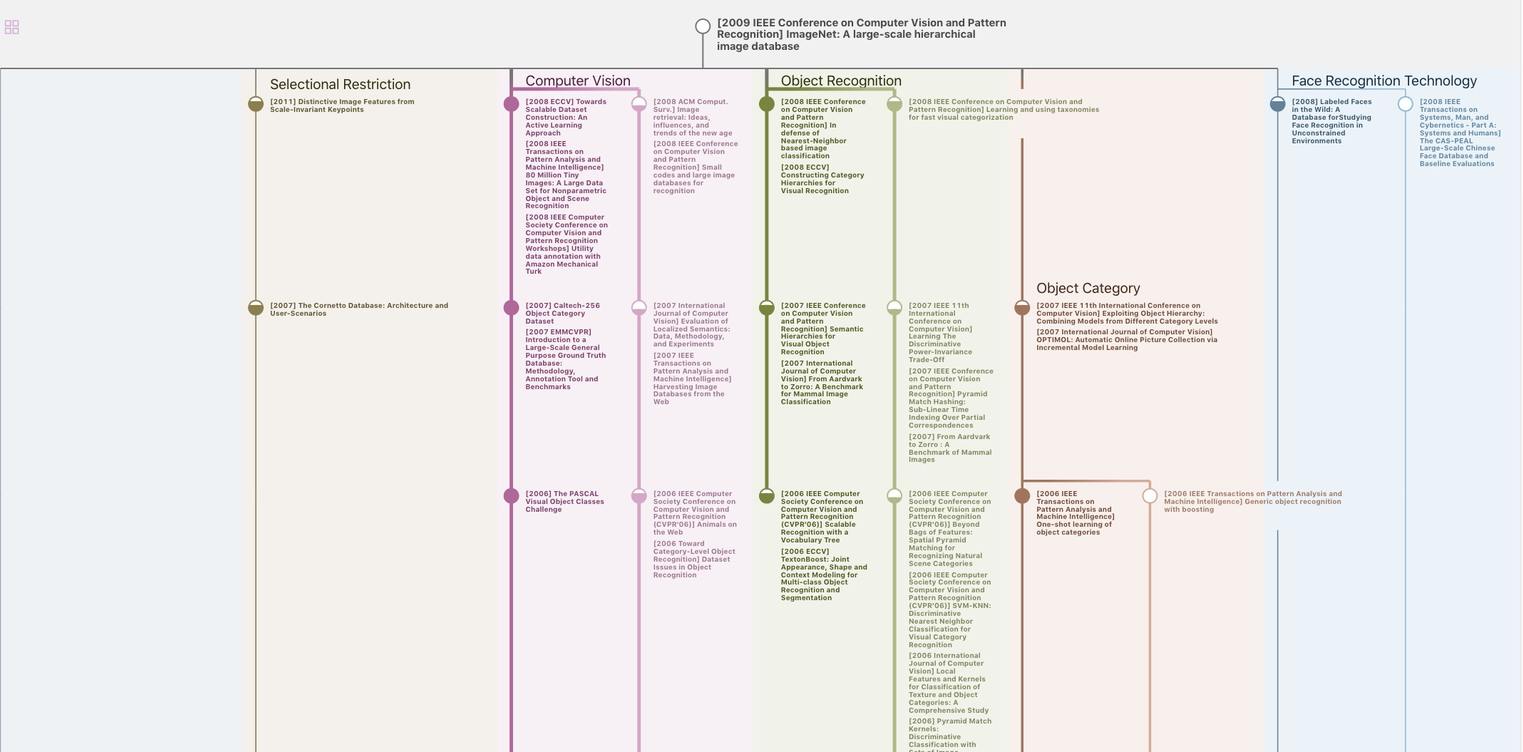
生成溯源树,研究论文发展脉络
Chat Paper
正在生成论文摘要