Instrument-To-Instrument translation: Instrumental advances drive restoration of solar observation series via deep learning
Research Square (Research Square)(2024)
摘要
The constant improvement of astronomical instrumentation provides the
foundation for scientific discoveries. In general, these improvements have only
implications forward in time, while previous observations do not benefit from
this trend. Here we provide a general deep learning method that translates
between image domains of different instruments (Instrument-To-Instrument
translation; ITI). We demonstrate that the available data sets can directly
profit from the most recent instrumental improvements, by applying our method
to five different applications of ground- and space-based solar observations.
We obtain 1) solar full-disk observations with unprecedented spatial
resolution, 2) a homogeneous data series of 24 years of space-based
observations of the solar EUV corona and magnetic field, 3) real-time
mitigation of atmospheric degradations in ground-based observations, 4) a
uniform series of ground-based Hα observations starting from 1973, 5)
magnetic field estimates from the solar far-side based on EUV imagery. The
direct comparison to simultaneous high-quality observations shows that our
method produces images that are perceptually similar and match the reference
image distribution.
更多查看译文
关键词
solar observation series,deep learning,instrumental advances,translation,instrument-to-instrument
AI 理解论文
溯源树
样例
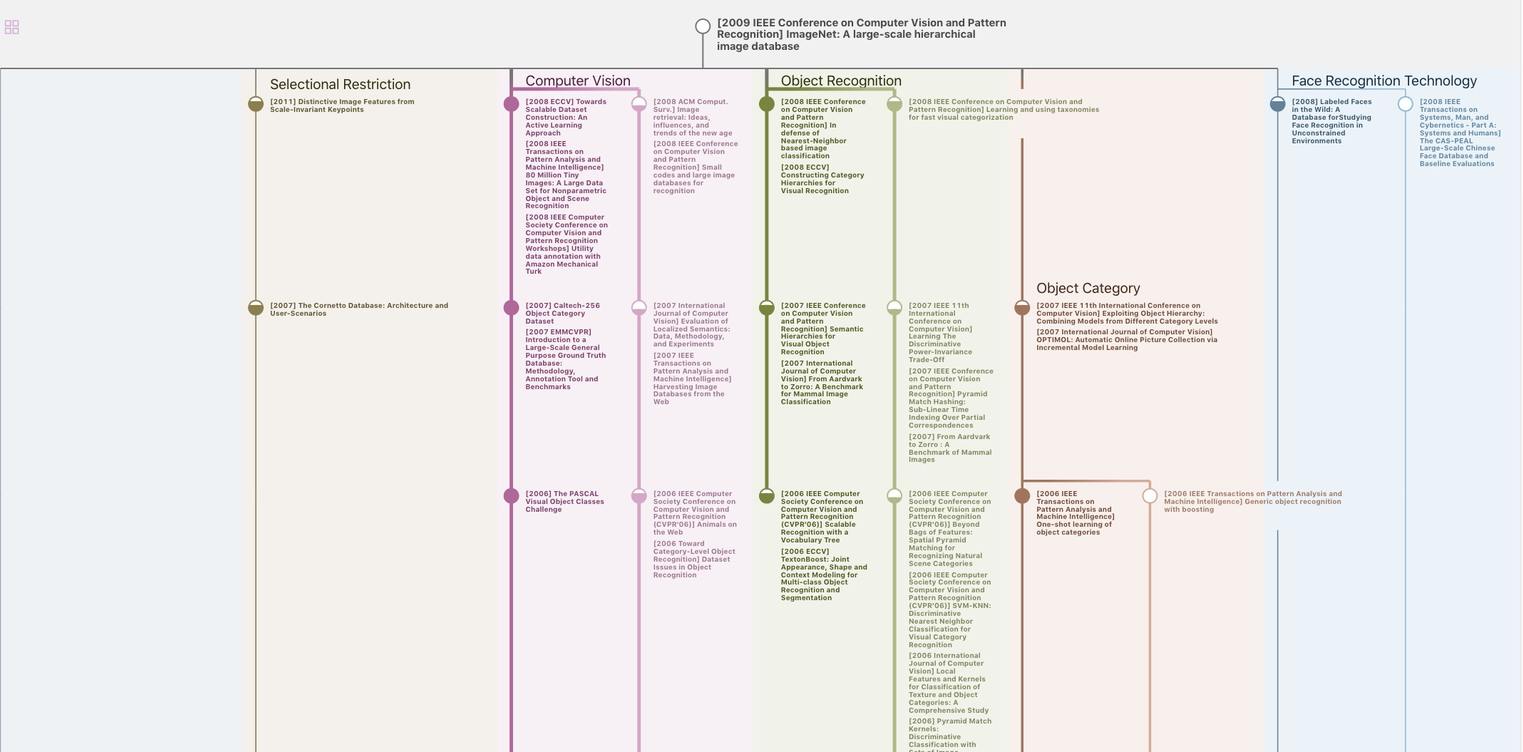
生成溯源树,研究论文发展脉络
Chat Paper
正在生成论文摘要