Non-Invasive Evaluation of MAFLD and the Contribution of Genes: An MRI-PDFF-Based Cross-Sectional Study
Research Square (Research Square)(2022)
摘要
Abstract Objective To investigate the clinical, laboratory and genetic features of MAFLD patients based on MRI-PDFF and liver biopsy in China. Design: Patients with high ALT and with a diagnosis of fatty liver were included in this cross-sectional study. Fasting blood was collected to test biomarkers and SNPs. A total of 266 patients underwent MRI-PDFF and FibroScan examinations, and 38 underwent liver biopsy. Diagnostic models (decision tree, LASSO, and elastic net) were developed based on diagnosis from MRI-PDFF reports. Results Approximately 1/3 were found to have NASH and fibrosis. After quantifying liver steatosis by MRI-PDFF (healthy: N = 47; mild MAFLD: N = 136; moderate/severe MAFLD: N = 83; liver fat content (LFC): 3.6% vs. 8.7% vs. 19.0%), most biomarkers showed significant differences among the three groups, and patients without obesity were found to have a similar LFC as those with obesity (11.1% vs. 12.3%). Variant alleles of PNPLA3, HSD17B13 and MBOAT7 were identified as genetic risk factors causing higher LFC (8.7% vs. 12.3%; 11.0% vs. 14.5%; 8.5% vs. 10.2%, P < 0.05); those with the UQCC1 rs878639 variant allele showed lower LFC (10.4% vs. 8.4%; OR = 0.58, P < 0.05). Patients with more risk alleles had higher LFCs (8.1% vs. 10.7% vs. 11.6% vs. 14.5%). Models including biomarkers showed strong diagnostic ability (accuracy: 0.80–0.91). Conclusions When the effects of genes on liver steatosis were first quantified by MRI-PDFF, the UQCC1 rs878639 G allele was identified as a protective factor, and the MBOAT7 T allele was identified as a risk only among nonobese individuals. Machine learning-based models can accurately predict disease status.
更多查看译文
关键词
mafld,genes,non-invasive,mri-pdff-based,cross-sectional
AI 理解论文
溯源树
样例
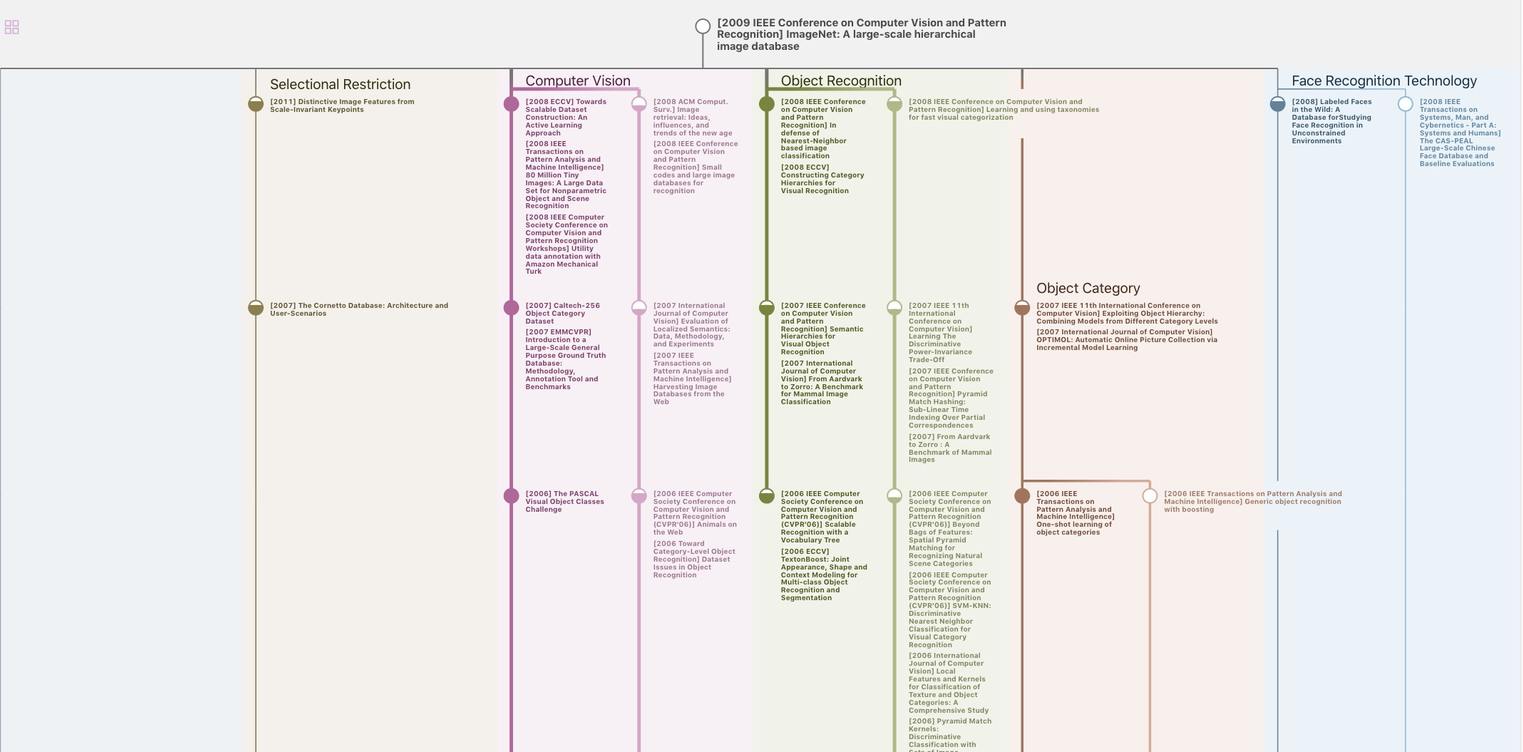
生成溯源树,研究论文发展脉络
Chat Paper
正在生成论文摘要