A study on the performance of low-cost sensors for source apportionment at an urban background site
crossref(2022)
摘要
Abstract. While the measurements of atmospheric pollutants are useful in understanding the level of the air quality at a given area, receptor models are equally important in assessing the sources of these pollutants and the extent of their effect, helping in policy making to deal with air pollution problems. Such analyses were limited and were attempted until recently only with the use of expensive regulatory-grade instruments. In the present study we applied a two-step Positive Matrix Factorisation (PMF) receptor analysis at a background site using data acquired by low-cost sensors (LCS). Using PMF, the identification of the sources that affect the air quality at the background site in Birmingham provided results that were consistent with a previous study at the site, even though in different measuring periods, but also clearly separated the anticipated sources of particulate matter (PM) and pollution. Additionally, the method supplied a metric for the contribution of different sources to the overall air quality at the site, thus providing pollution source apportionment. The use of data from regulatory-grade (RG) instruments further confirmed the reliability of the results, as well as further clarifying the particulate matter composition and origin. Comparing the results from a previous analysis, in which a k-means clustering algorithm was used, a good consistency between the results was found, and the potential and limitations of each method when used with low-cost sensor data are highlighted. The analysis presented in this study paves the way for more extensive use of LCS for atmospheric applications and receptor modelling. Here, we present the infrastructure for understanding the factors that affect the air quality at a significantly lower cost that previously possible, thus opening up multiple new opportunities for regulatory and indicative monitoring for both scientific and industrial applications.
更多查看译文
AI 理解论文
溯源树
样例
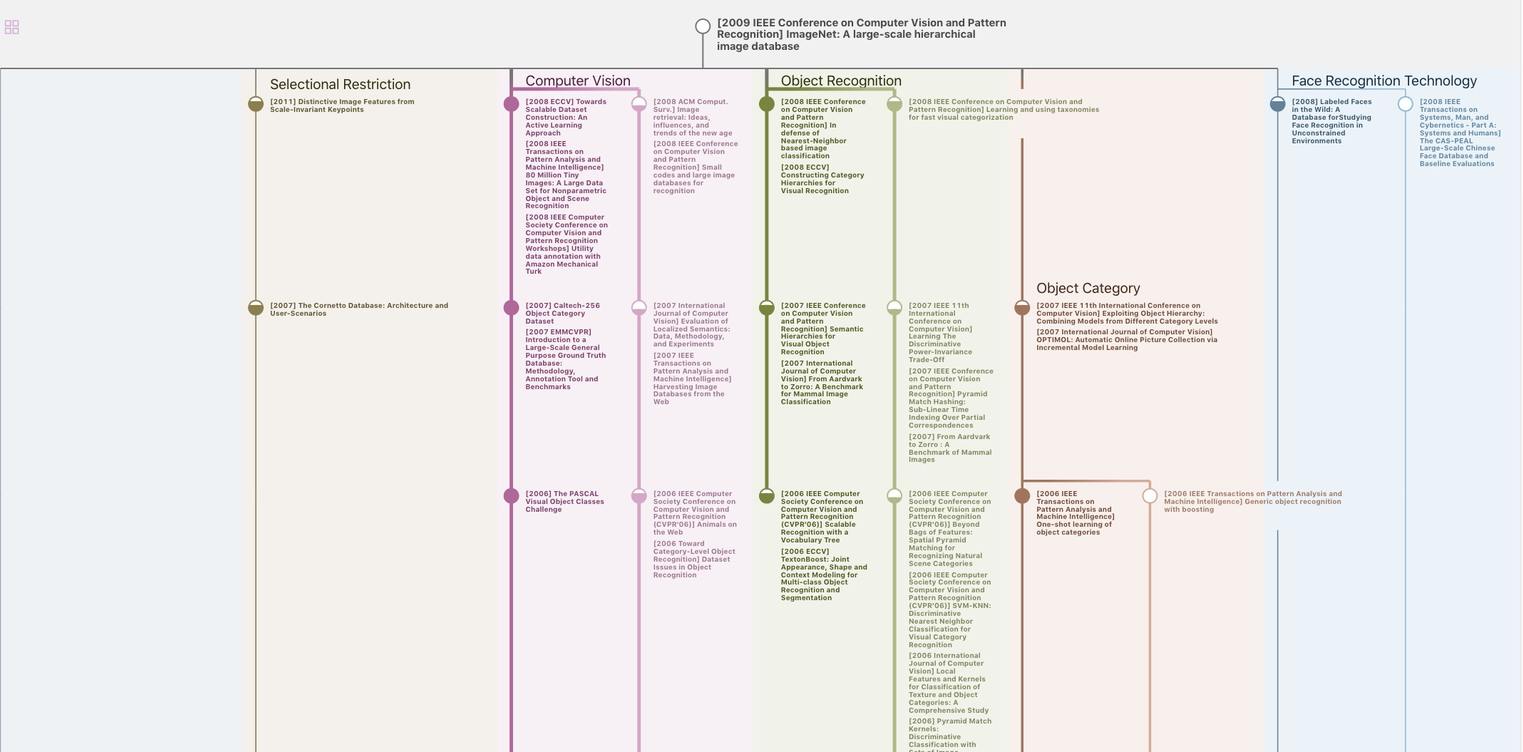
生成溯源树,研究论文发展脉络
Chat Paper
正在生成论文摘要