Machine learning based estimation of regional Net Ecosystem Exchange (NEE) constrained by atmospheric inversions and ecosystem observations
crossref(2022)
摘要
<p>Accurate estimates and predictions of the global carbon fluxes are critical for our understanding of the global carbon cycle and climate change. Reducing the uncertainty of the terrestrial carbon sink and closing the budget imbalance between sources and sinks would improve our ability to accurately project future climate change. Net Ecosystem Exchange (NEE), the net flux of biogenic carbon from the land surface to the atmosphere, is only directly measured at a sparse set of globally distributed eddy-covariance measurement sites. To estimate the terrestrial carbon flux at the regional and global scale, a global gridded estimate of NEE must be accurately upscaled from a model trained at the ecosystem level. In this study, the Fluxcom system* is used to train a site-level model on remotely-sensed and meteorological variables derived from site measurements, MODIS and ECMWF ERA5 atmospheric reanalysis data. The non-representative distribution of these site-level data along with missing disturbance histories impart known biases to current upscaling efforts. Observations of atmospheric carbon may provide important additional information, improving the accuracy of the upscaled flux estimate. </p><p>This study adds an atmospheric observational operator to the model training process that connects the ecosystem-level flux model to top-down observations of atmospheric carbon by adding an additional term to the objective function. The target data are regionally integrated fluxes from an ensemble of atmospheric inversions corrected for fossil-fuel emissions and lateral fluxes.  Calculating the regionally integrated flux estimate at each training step is computationally infeasible. Our hypothesis is that the regional flux can be modeled with a limited set of points and that this sparse model preserves sufficient information about the phenomena to act as a constraint for the underlying ecosystem-level model, improving regional and global upscaled products.  Experimental results show improvements in the machine learning based regional estimates of NEE while preserving features such as the seasonal variability in the estimated flux.</p><p> </p><p>*Jung, Martin, Christopher Schwalm, Mirco Migliavacca, Sophia Walther, Gustau Camps-Valls, Sujan Koirala, Peter Anthoni, et al. 2020. “Scaling Carbon Fluxes from Eddy Covariance Sites to Globe: Synthesis and Evaluation of the FLUXCOM Approach.” <em>Biogeosciences</em> 17 (5): 1343–65. </p><p> </p>
更多查看译文
AI 理解论文
溯源树
样例
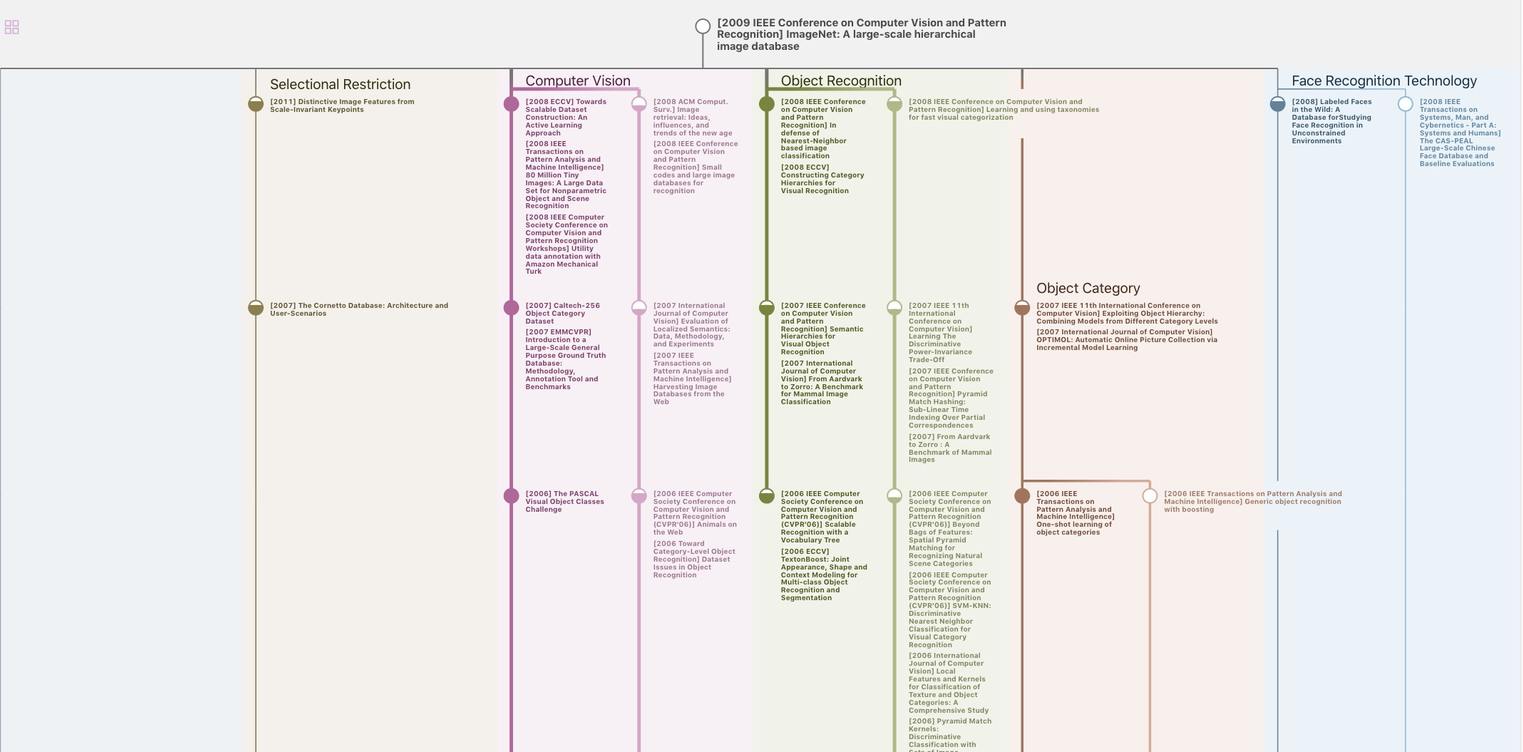
生成溯源树,研究论文发展脉络
Chat Paper
正在生成论文摘要