Optimal estimation of thermodynamic and microphysical profiles within fog events from ground-based microwave radiometer and cloud radar synergy.
crossref(2022)
摘要
<p>
The fog lifecyle and more especially formation and dissipation times are still poorly represented in even the highest resolution operational numerical weather prediction (NWP) models, causing large economic costs in the aviation industry. A new, continuous transmission, 95 GHz cloud radar (CR), sensitive to cloud and fog droplets, opens up the possibility of retrieving vertical profiles of fog microphysical properties with unprecedented capabilities. Additionally, well-know ground-based microwave radiometers (MWR) can provide detailed information on the fog thermodynamics and total liquid water path. This work aims at combining ground-based MWR and CR measurements constrained with short-term-forecasts (called background profiles) from the high-resolution operational model AROME into a one dimensional variational approach (1DVAR) in order to retrieve physically consistent temperature, humidity and liquid water content profiles within fog conditions. Firstly, background and forward model errors during fog conditions are investigated and a methodology of improving the background profile is proposed. Then, a first evaluation of the algorithm using a synthetic dataset will be presented. This evaluation aims at quantifying the capabilities of the algorithm on idealized conditions, providing an upper limit on the performance that could be reached by the algorithm. The algorithm is then applied to real observations from the SOFOG3D <span>(</span><span>SOuth FOGs 3D experiment for fog processes study</span><span>) </span><span>field experiment led by Météo-France during the winter 2019/2020 </span><span>where the 95 GHz B</span><span>ASTA</span><span> cloud radar has been collocated with the HATPRO MWR together with in-situ observations. </span><span>The capability of the algorithm to retrieve liquid water contents with an approximate error of 0.05 g.m</span><sup><span>-</span></sup><sup><span>3</span></sup>  <span>but also temperature and humidity profiles with an accuracy better than 1 K and 1 g.kg</span><sup><span>-</span></sup><sup><span>1</span></sup><span> respectively </span><span>thanks to comparison with in-situ measurements (radiosondes and cloud droplet probe measurements) </span><span>has been demonstrated. </span><span>A sensitivity study will also be presented to identify the major sources of uncertainties in the algorithm (microphysics assumption and 1D-Var background-error-covariance matrix). </span><span>These developments open new capabilities for future assimilation of the retrieved profiles in the new </span><span>AROME ensemble data assimilation scheme </span><span>and to better understand physical processes </span><span>taking part in the fog lifecycle.</span></p>
更多查看译文
AI 理解论文
溯源树
样例
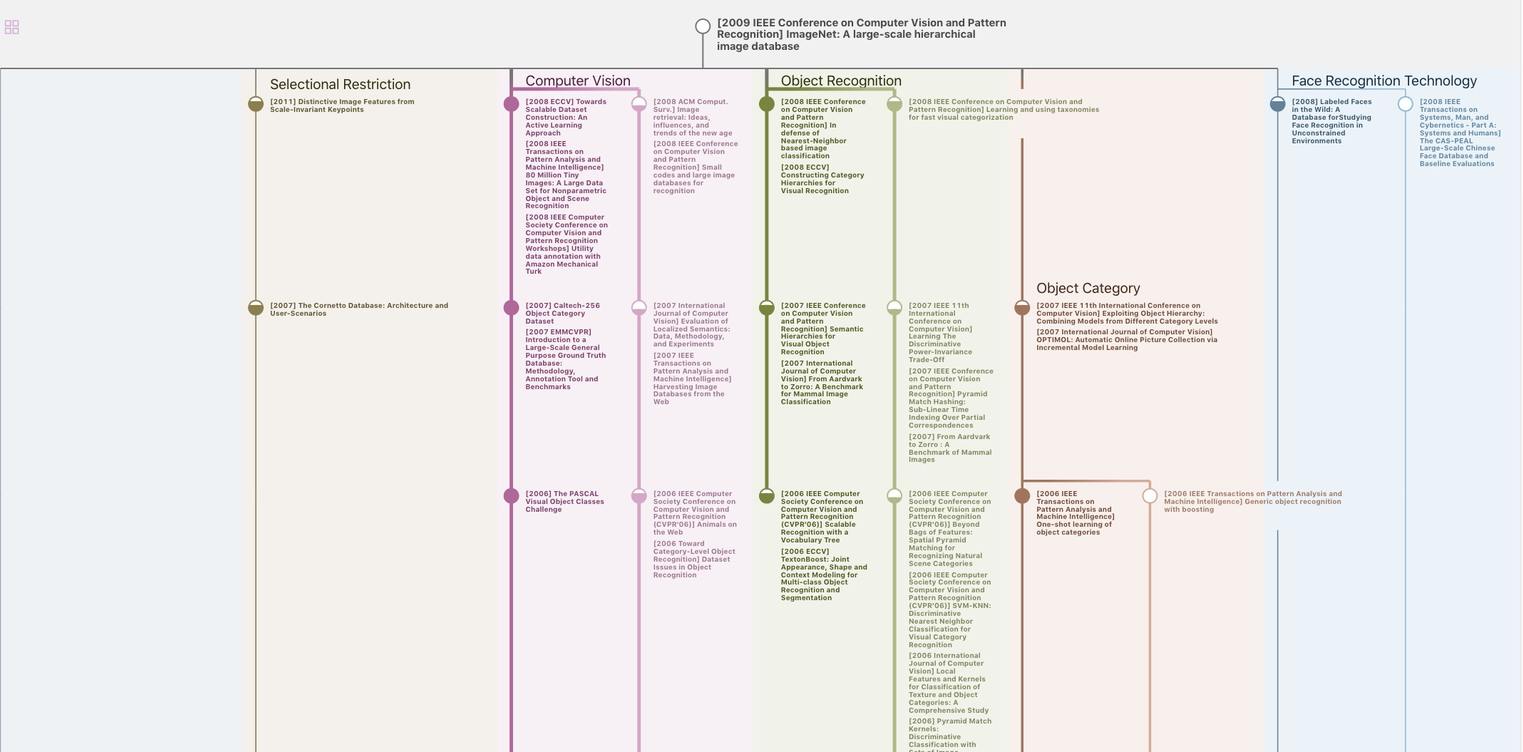
生成溯源树,研究论文发展脉络
Chat Paper
正在生成论文摘要