Urban Flood Mapping Uncertainty Justification using Variable Drop-off in RF and XGBoost Algorithms: A Case Study of Marib City, Yemen
crossref(2022)
摘要
<p>Urban flooding hazards are thought to be linked to climatic variability changes and population expansion, land use, elevation, slope, curvature, distance to river or canal, inadequate drainage, and more severe rainfall patterns. Therefore, urban flood modeling and uncertainty in the mapping of risk prediction are critical and should be considered. For this purpose, demystifying uncertainty in urban flood susceptibility mapping was modeled using a variable drop-off in random forest (RF) and XGBoost algorithms. This paper investigates the uncertainty that may arise in prediction mapping analysis when the predictive relevance level of the predictor factors varies significantly and particularly when the receiver operating characteristic fails to represent the resultant susceptibility map sensitivity with the influence of predictive factors.</p><p>We used Sentinel-1 images and Landsat satellite data to create a flood inventory map to map flooded areas. In total, 240 random flood and non-flood points were chosen, with 75% used for training and 25% for validation. The area under the curve, overall accuracy, confusion matrix, number of parameters, overfitting, and significant distribution were compared. For both models, drainage density was the most important, followed by the elevation, rainfall, normalized difference vegetation index, and road distance. By contrast, topographic wetness index, slope, aspect, and curvature were the least critical variables. After implementing the episode, stopping at nine variables, and testing the overfitting, the XGBoost model outperforms the RF model in the final map output. By contrast, the RF algorithm shows the ability of the RF model to overcome overfitting when assessing maps from a single factor. The study results could lead to a new research path considering the uncertainty related to different parts of the susceptibility modeling procedure and possible solutions to quantifying it.</p>
更多查看译文
AI 理解论文
溯源树
样例
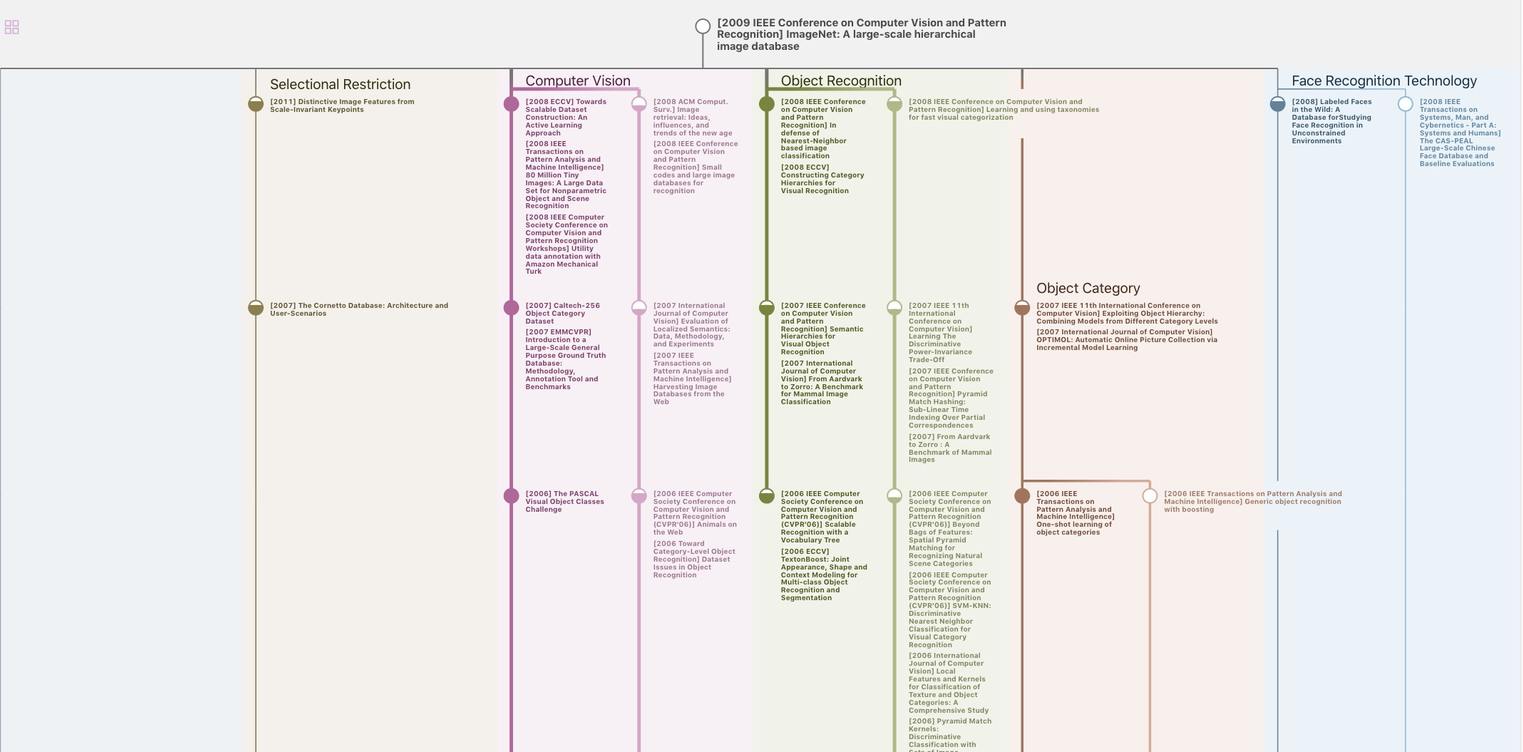
生成溯源树,研究论文发展脉络
Chat Paper
正在生成论文摘要