Enabling carbon farming: presentation of a robust, affordable and scalable method of carbon stock estimates
crossref(2022)
摘要
<p>The main hurdle in instrumentalizing agricultural soils to sequester atmospheric carbon is a development of methods to measure soil carbon stocks on farm level which are robust, scalable and globally available. We present a method for soil carbon stock assessment using satellite data, stratified sampling design, direct soil measurements via mobile soil sensors and machine learning, which fulfills these criteria. The method has been tested and applied for multiple farms in Europe and the United States on agricultural fields with variable crop rotations, soil types and management history. Results show that the estimates are precise, repeatable and that the approach is rapidly scalable and is able to detect variation in soil carbon stock up to a 10 meter resolution. Carbon stocks in the top 30 cm range between 17-55 ton C/hectare. Error propagation analysis showed that the error associated with the soil sensor on the level of farm C stocks is less than 5%. This precision can be achieved with as few as 0.5 field samples per hectare for farms varying from 60 to 250 hectares, ensuring affordability and scalability of the method. The method is globally applicable because it uses covariates which are also globally available. These findings can enable the structural and widespread implementation of carbon farming with a standardized method. In future, as the calibration dataset increases, even less field data will be needed to obtain robust C stock estimates.</p>
更多查看译文
AI 理解论文
溯源树
样例
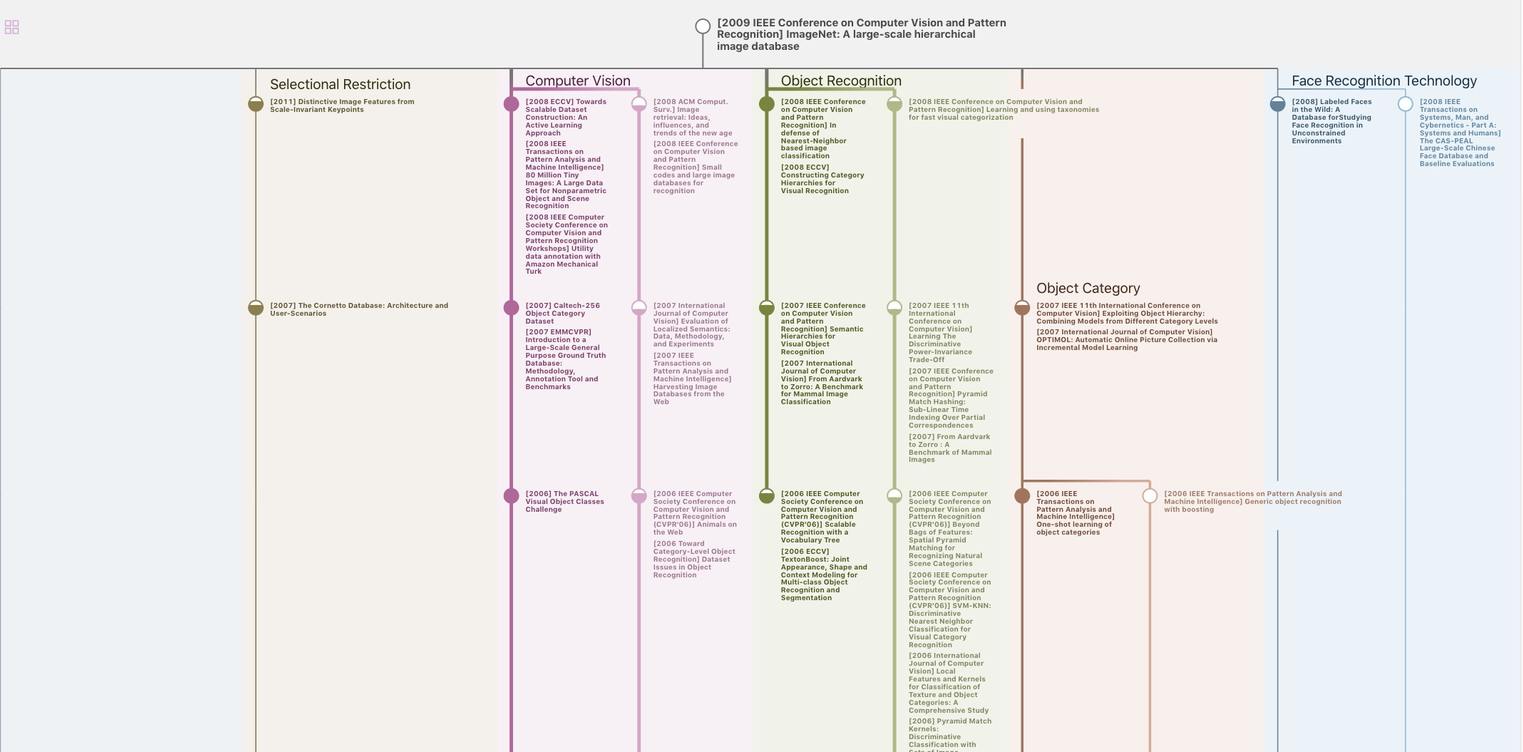
生成溯源树,研究论文发展脉络
Chat Paper
正在生成论文摘要