Building saturated hydraulic conductivity maps with machine learning and geostatistics
crossref(2022)
摘要
<p>Hydraulic conductivity (K<sub>s</sub>) is one of the most challenging, time-consuming, and expensive soil hydraulic properties to estimate. Pedotransfer functions (PTF<sub>s</sub>) of general use for K<sub>s</sub> estimation are often site and sample-scale specific and perform poorly when extrapolated to different regions and extents. The present work develops a stepwise methodology for topsoil K<sub>s</sub> mapping at a catchment scale based on easy, fast, and inexpensive measurements and auxiliary data (Aguilera et al., 2022). It includes a double-scale sampling of the K<sub>s</sub> to account for small-scale variability in the spatial geostatistical interpolation. A supervised selection of variables through correlation analysis and hierarchical clustering of variables precedes the development of site-specific PTFs with machine learning (ML) techniques. The ML model is then used to generate new K<sub>s</sub> point data predictions to extend the spatial coverage for mapping. Finally, the consistency of the final K<sub>s</sub> map is assessed in terms of a geomorphological base map.</p><p>The variable selection process filtered out four predictor variables from the initial pool of fourteen predictors. An artificial neural network (ANN) provided the best K<sub>s</sub> prediction model with one hidden layer and six input variables (latitude, longitude, silt, clay, medium sand, and land use). Latitude and longitude coordinates and land use are surrogates for other physical and environmental (e.g., anthropic) factors. The relative importance of input variables in the ANN was determined as the sum of the product of raw input-hidden, hidden-output connection weights across all hidden neurons using Olden's algorithm. Longitude, percentage of clay, and percentage of medium sand presented a stronger positive relationship with K<sub>s</sub>, while irrigated and dry land uses together with the percent of silt were the variables with a more significant negative influence on K<sub>s</sub>. The fact that K<sub>s</sub> was positively related to clay content is surprising, and it appears to be related to soil plowing before sampling.</p><p>The ANN was used to estimate new K<sub>s</sub> values from a subsequent sampling of model covariates, which doubled the input information for spatial interpolation using ordinary kriging. Overall, the spatial distribution of K<sub>s</sub> was consistent with the lithological variability and other superimposed anthropic factors, as the method adequately considers the spatial variability of K<sub>s</sub> added by anthropization to the already high natural heterogeneity. The produced maps will help in the hydraulic planning and flood risk management in the study area where high and low K<sub>s</sub>, respectively, are clearly outlined.</p><p>Reference:</p><ul><li>Aguilera, C. Guardiola-Albert, L. Moreno Merino, C. Baquedano, E. Díaz-Losada, P. Agustín Robledo Ardila, J.J. Durán Valsero, Building inexpensive topsoil saturated hydraulic conductivity maps for land planning based on machine learning and geostatistics, CATENA, Volume 208, 2022, 105788, https://doi.org/10.1016/j.catena.2021.105788.</li> </ul><p>This work is performed within the framework of the RESERVOIR project, part of the PRIMA Programme supported by the European Union. The PRIMA programme is supported under Horizon 2020 the European Union's Framework Programme for Research and Innovation. PRIMA RESERVOIR Grant Agreement number is 1924.</p>
更多查看译文
AI 理解论文
溯源树
样例
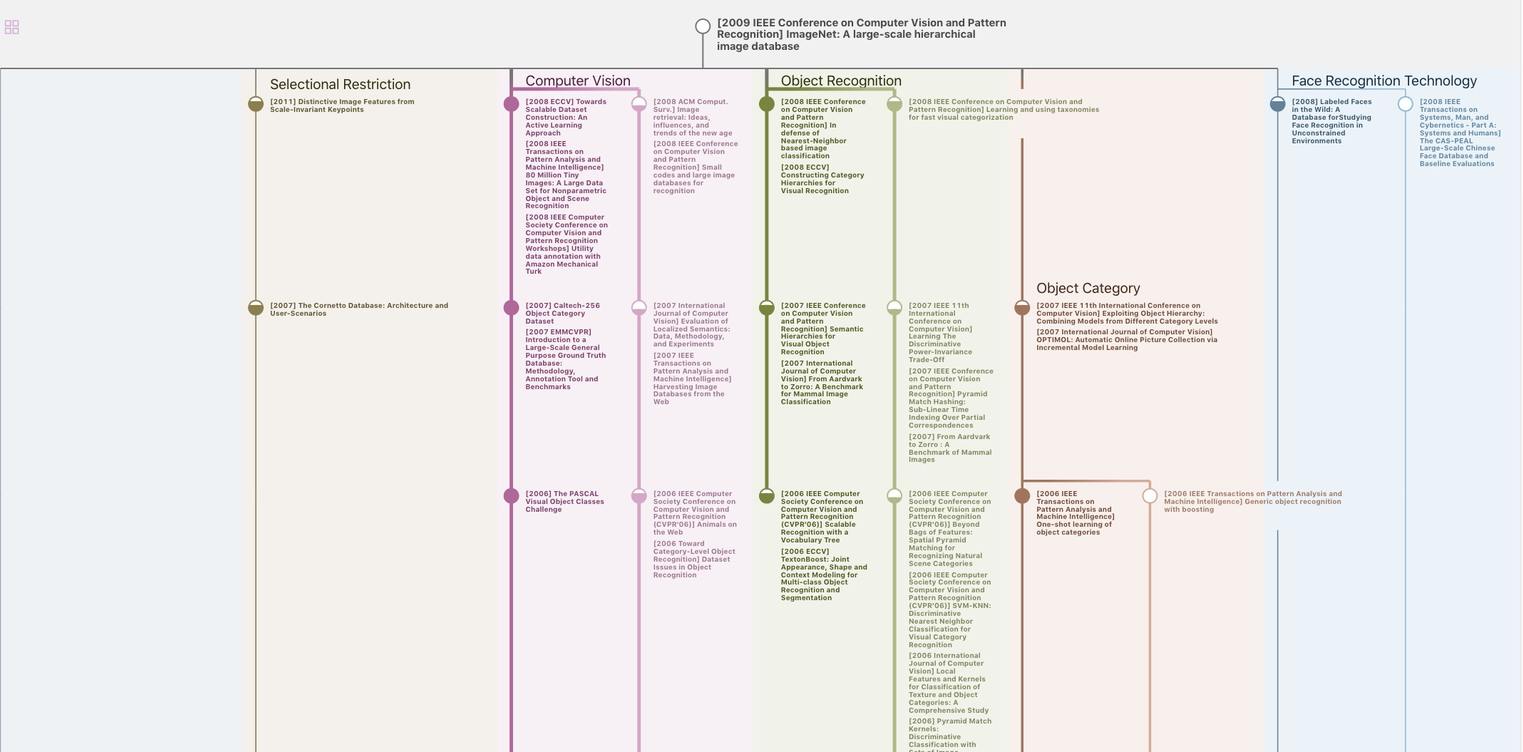
生成溯源树,研究论文发展脉络
Chat Paper
正在生成论文摘要