Empirical Mode Modeling: A data-driven approach to recover and forecast nonlinear dynamics from noisy data
arXiv (Cornell University)(2022)
摘要
<p>Data-driven, model-free analytics are natural choices for discovery and<br>forecasting of complex, nonlinear systems. Methods that operate in the system<br>state-space require either an explicit multidimensional state-space, or, one ap-<br>proximated from available observations. Since observational data are frequently<br>sampled with noise, it is possible that noise can corrupt the state-space representa-<br>tion degrading analytical performance. Here, we evaluate the synthesis of empirical<br>mode decomposition with empirical dynamic modeling, which we term empirical<br>mode modeling, to increase the information content of state-space representations<br>in the presence of noise. Evaluation of a mathematical, and, an ecologically im-<br>portant geophysical application across three different state-space representations<br>suggests that empirical mode modeling may be a useful technique for data-driven,<br>model-free, state-space analysis in the presence of noise.</p>
更多查看译文
关键词
empirical mode modeling,nonlinear dynamics,forecast
AI 理解论文
溯源树
样例
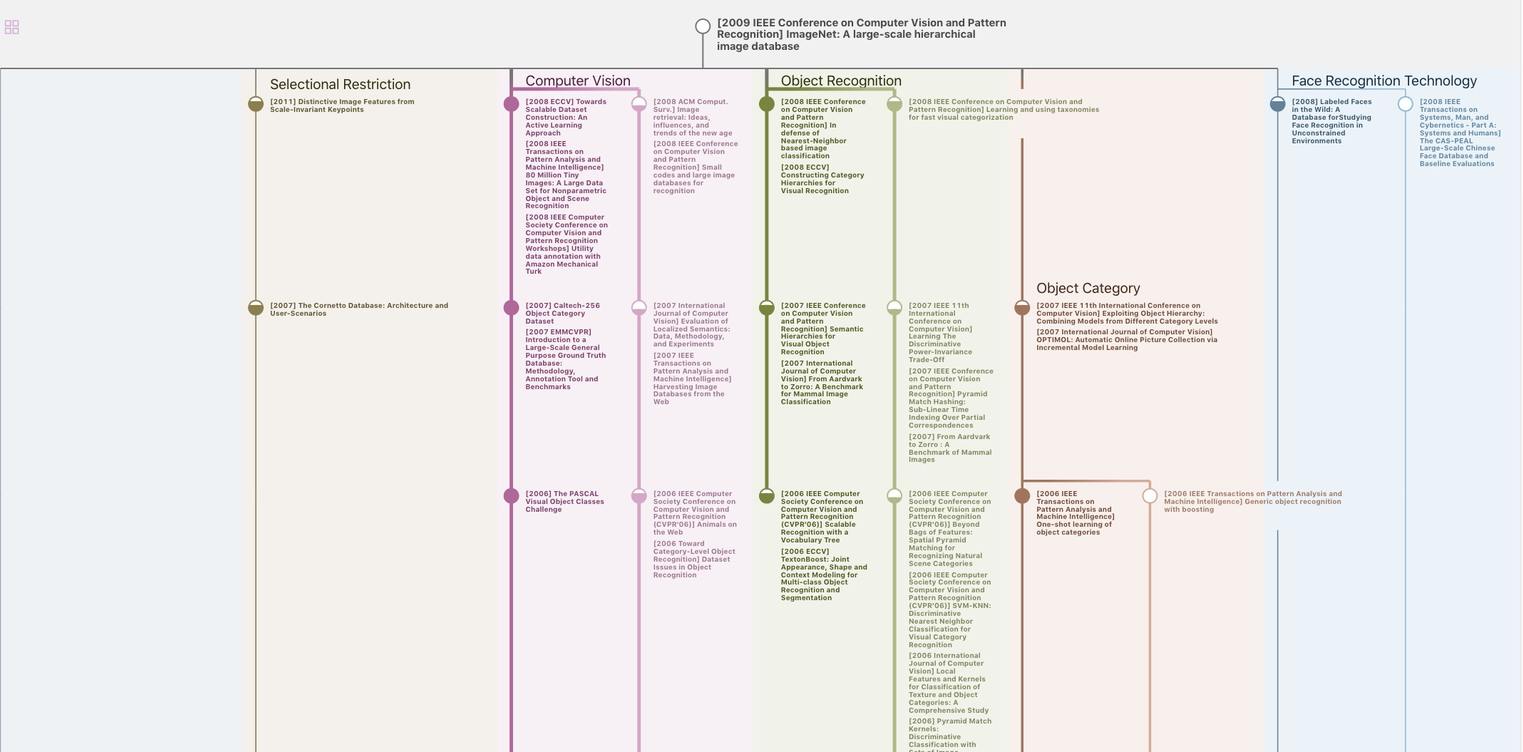
生成溯源树,研究论文发展脉络
Chat Paper
正在生成论文摘要