Performance analysis of missing data imputation methods for daily groundwater hydrographs using typical gap patterns
crossref(2022)
摘要
<p>Regular and gapless observations are necessary to perform a range of statistical analysis on the parameter of interest. Groundwater level (GWL) hydrographs  are often recorded at irregular frequencies and have time periods without any observations. As a result, groundwater level hydrographs have missing values. Typically groundwater hydrographs are removed from further analysis if large gaps are present, while each groundwater observation point is valuable and methods exist that can impute (fill in) the missing observations. </p><p>This study aims to evaluate performance of machine learning methods to prepare gapless daily groundwater level hydrographs and to assess the imputation error according to various approaches. Filled groundwater level hydrographs will further be used  to identify typical groundwater level patterns in the Baltic region.</p><p>The performance of two machine learning imputation methods - missForest and missMDA - along with conventional approaches (linear interpolation, mean imputation) - were tested. A subset of the GWL observation data from Lithuania, Latvia and Estonia were used for the time period from 2011 to mid-2019 comprising 283 groundwater monitoring wells. Cluster analysis of the temporal distribution of actual missing values in the GWL time series provided 13 different gap patterns. Next a corresponding number of artificially generated gap distribution scenarios were defined. The performance of various gap-filing approaches were then evaluated by imputing each artificially generated gap pattern in each hydrograph. Results indicated that imputation performance varies among different clusters of missing value patterns, while generally the best performance was achieved by the missForest algorithm.</p><p>This research is funded by the Latvian Council of Science, project “Spatial and temporal prediction of groundwater drought with mixed models for multilayer sedimentary basin under climate change”, project No. lzp-2019/1-0165.</p>
更多查看译文
AI 理解论文
溯源树
样例
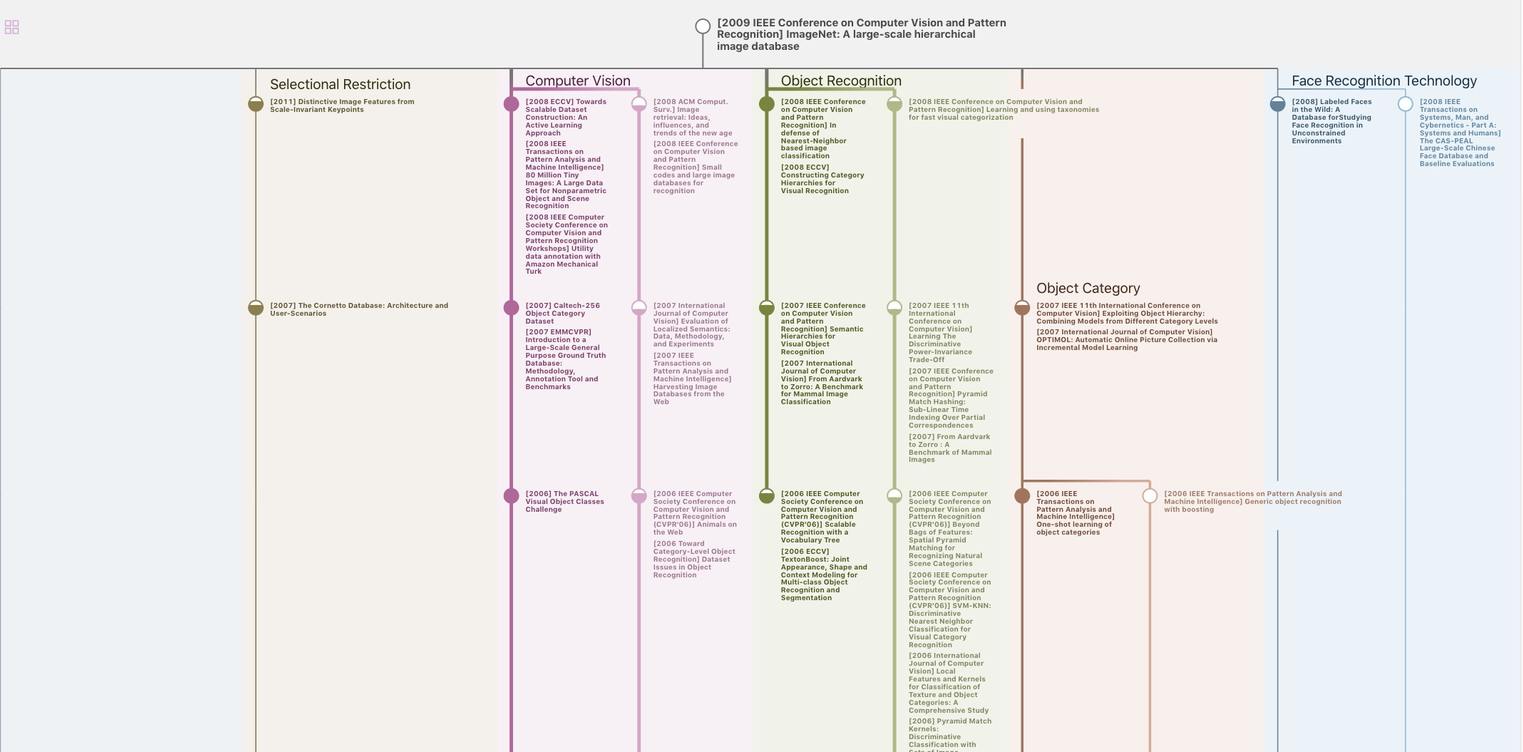
生成溯源树,研究论文发展脉络
Chat Paper
正在生成论文摘要