Assessing Machine Learning algorithms to fill gaps of daily evapotranspiration measured in a citrus orchard using a combination of agro-meteorological and remote sensing data
crossref(2022)
摘要
<p>The knowledge of crop evapotranspiration is crucial for several hydrological processes, including those related to the management of agricultural water sources. Among indirect methods to estimate actual evapotranspiration, ET<sub>a</sub>, the measurements of latent heat fluxes acquired with Eddy Covariance (EC) tower have been largely used. However, the malfunctioning of the commonly installed sensors can cause the loss of data, compromising the temporal continuity of the acquisitions. Machine learning (ML) algorithms can represent a powerful tool to perform gap-filling procedures and provide accurate predictions of missing data.</p><p>The objective of the research was to assess different ML algorithms to fill daily actual evapotranspiration measurements acquired in a Mediterranean citrus orchard, by using a combination of in-situ or ERA5-Land reanalysis agro-meteorological data and two vegetation indices (VIs) retrieved by the Sentinel 2 platform.</p><p>The experimental layout consisted of a standard weather station, an EC tower containing an open-patch gas-analyzer, a three-dimension sonic anemometer, a four-component net radiometer. Four “drill and drop” probes (Sentek Pty Ltd, Stepney, Australia) were also installed in the field. Six different algorithms of machine learning were tested, using in input, as climate variables the global solar radiation, mean air temperature and relative air humidity, as well as two VIs (NDVI e NDWI) characterized by a spatial resolution of 10 m and, finally, the average soil water content measured in the root zone (0-50 cm). The number of daily ET<sub>a</sub> measurements, acquired from March 2019 to September 2021, resulted in about 70% of the total days of the period; the missing data were caused by the malfunctioning of installed instruments, which occurred during the lockdown restrictions caused by the pandemic.</p><p>Among the different ML algorithms, the best performance was associated with the Gaussian Process Regression (GPR) based on nonparametric kernel probabilistic function (Rasmussen, 2006) when, with the other input variables, the average soil water content was included; in this case, the values of root mean square error (RMSE) and determination coefficient (R<sup>2</sup>) associated with the cross-validation resulted equal to 0.42 mm/d and 0.85, respectively. However, suitable results were also associated with the GPR model, when assuming, as input variables, on-site meteorological data and VIs (RMSE=0.49 mm/d and R<sup>2</sup>=0.78), or when considering the ERA5-Land meteorological variables (RMSE=0.56 mm/d and R<sup>2</sup>=0.73). The joint use of agro-meteorological and remote sensing data, associated with a GPR model, can therefore provide the opportunity to fill the gaps of ETa time-series.</p>
更多查看译文
AI 理解论文
溯源树
样例
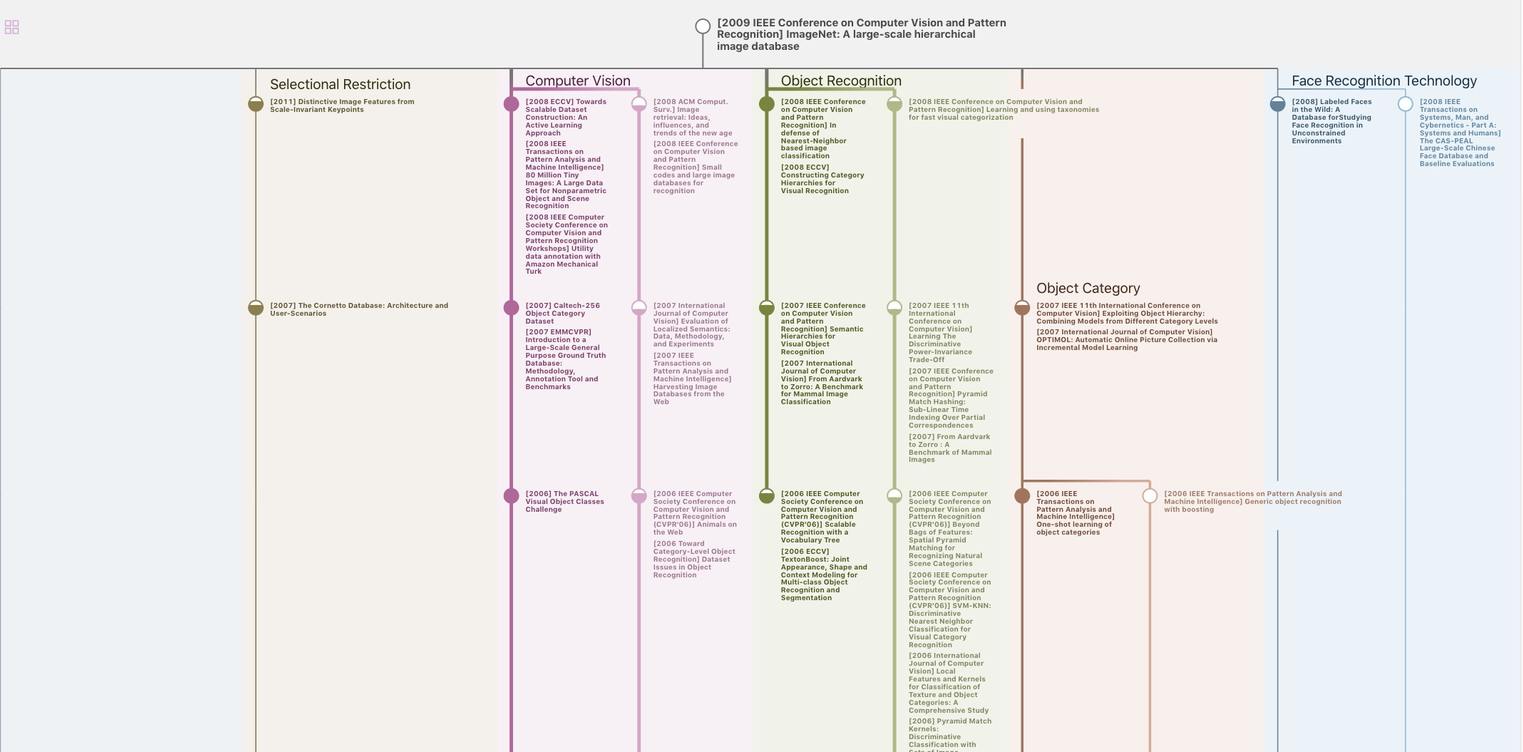
生成溯源树,研究论文发展脉络
Chat Paper
正在生成论文摘要