Remote sensing estimation of sea surface temperature and salinity from multi and hyperspectral ground and satellite ocean colour images.
crossref(2022)
摘要
<p>Sea surface salinity (SSS) and Sea surface temperature (SST) are important measures of Ocean health. They provide information about ocean warming, atmospheric interactions, and acidification, with further effects on the global thermohaline circulation and as a consequence the global water cycle. Temperature and salinity can be linked to ecosystem productivity, can help to stratify different marine zones, while salinity can be used as a tracer for pollutants and is a measure of land influence in the ocean.</p><p>Satellite-derived ocean colour provides the opportunity to process data at a higher temporal and spatial resolution than in-situ monitoring, with its pointwise spatial data limitation, or that of traditional microwave remote sensing, with ocean colour satellites having pixel resolution on the tens to hundreds metre scale compared to microwave km scale.</p><p>This paper explores the methodology to extract SST and SSS from multispectral ocean radiance. Water leaving radiance is linked to the inherent optical properties of the water column, effected by the constituent parts for example from coloured dissolved organic matter, chlorophyll and total suspended matter. These parts contribute to the backscatter, absorption and reflectance coefficients, influencing the spectral signature of the radiance.</p><p> </p><p>The method uses temporally and spatially matched water data of SSS and SST in Patagonia, with spectral radiance. First ground level hyperspectral values are used to train a linear regression model. The model is then able to learn the relationship between the spectral input and SSS/SST values and accurately estimates SSS and SST in test regions using just the spectral signature as input.  Further work is also done on using principle component analysis to reduce the dimensionality of the inputs whilst maintaining predictive accuracy. Feature selection was also examined to understand the spectral bands with the strongest contribution to either the salinity or temperature values, to be used in selecting the band coefficients for converting to the multispectral satellite images. Then to increase the complexity of the model, machine learning algorithms (for example neural networks) are trained to be able to learn more complex relationships and predict values in different optical water types.</p><p>These models are tested using multispectral satellite images matched with the Patagonia field water data, to predict the oceanographic values from top of atmosphere (TOA) satellite image data.</p><p>This paper demonstrates a link between the ocean colour/ radiance values and the sea surface physical properties temperature and salinity, with a model accurately estimating SST and SSS from just radiance. This relationship between sea surface properties and spectral signatures is then tested in satellite ocean colour images which can be used to monitor these environmental properties with more regular temporal sampling, better spatial coverage and in previously inaccessible locations</p><p> </p><p>Acknowledgements: Jose Luis Iriarte for in-situ SST/SST data from Patagonia.</p>
更多查看译文
AI 理解论文
溯源树
样例
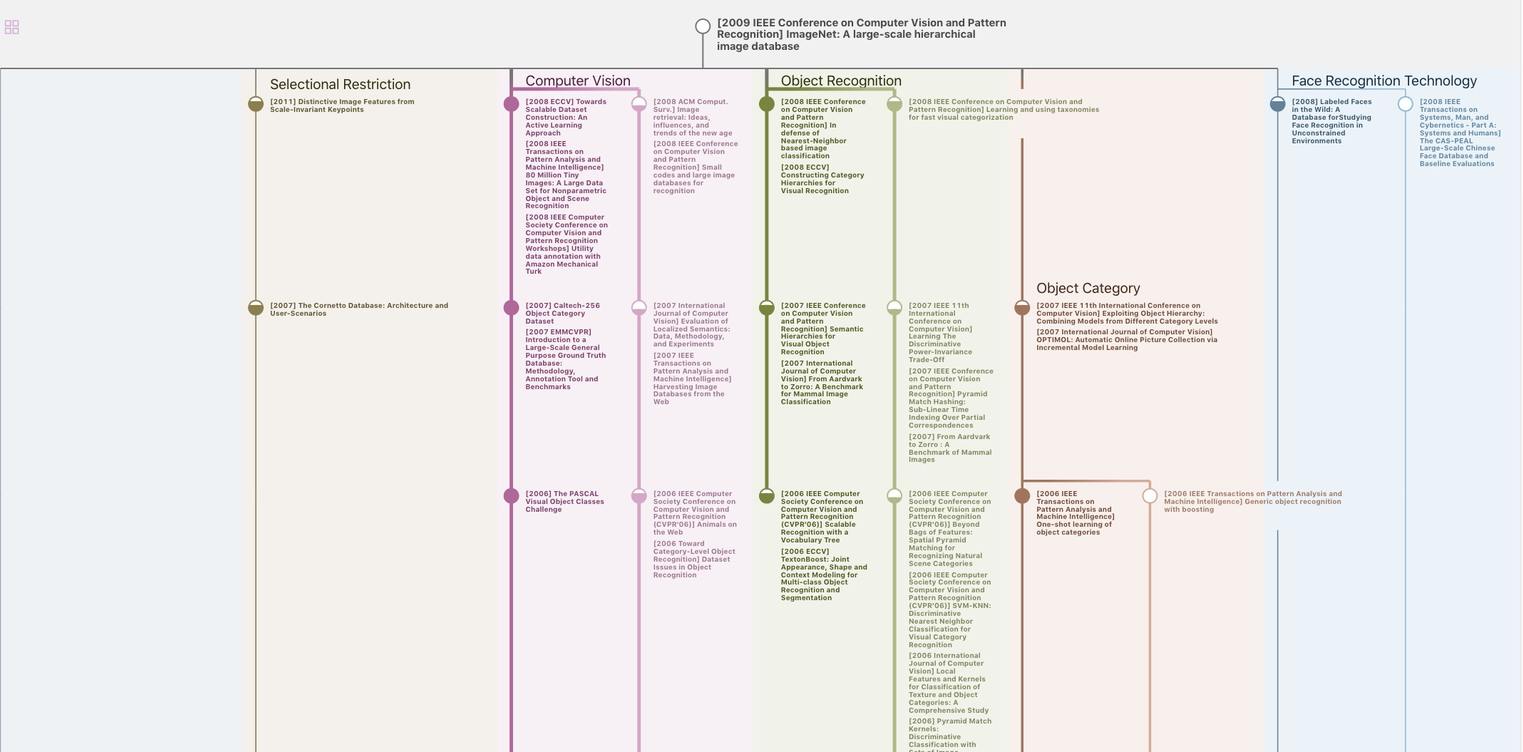
生成溯源树,研究论文发展脉络
Chat Paper
正在生成论文摘要