MTGPVM: Adaptive Active Learning Assisted Virtual Metrology Modeling in Chemical Vapor Deposition Systems
Research Square (Research Square)(2022)
摘要
Abstract Virtual metrology estimates the quality using processing variables collected from the multi-sensor system and enhances the detection efficiency compared with manual real metrology. However, the multitask learning problem and few labeled sets from available real metrology are challenges for virtual metrology modeling. Accordingly, this paper presents the improved method to combine multi-task Gaussian process and adaptive active learning for the application of virtual metrology in intelligent manufacturing. Initially, a multitask Gaussian processes-based virtual metrology (MTGPVM) model is built by combining the coregionalization matrix and two basic kernel functions and sharing latent correlation characteristics among tasks. Furthermore, three query functions utilizing uncertain sampling and diversity sampling are proposed for the active learning of multitask virtual metrology. Additionally, the adaptive active learning algorithm is proposed for virtual metrology model training with the few labeled data of real metrology. Finally, the case study on the proposed methods is carried out based on the coating processing of solar silicon wafers with the technology of plasma-enhanced chemical vapor deposition (PECVD). The experimental results indicate that the MTGPVM model is based on the combination of outperformed Martern kernel and linear kernel other multitask regression models, the proposed adaptive active learning algorithm prompted the learning accuracy of MTGPVM model with a small amount of labeled training data.
更多查看译文
关键词
active learning,modeling,vapor
AI 理解论文
溯源树
样例
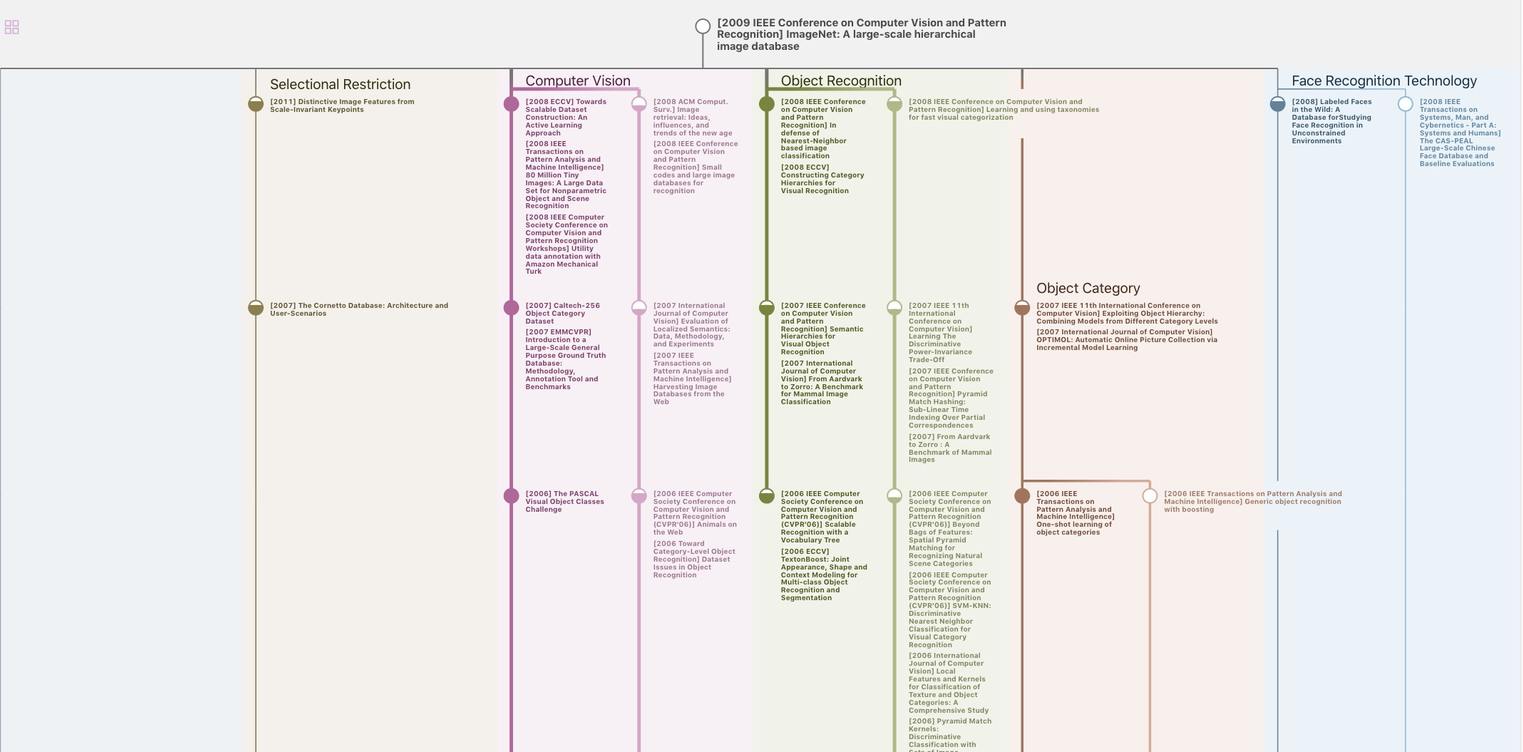
生成溯源树,研究论文发展脉络
Chat Paper
正在生成论文摘要