Effect of different pretreatment methods on classification of serum samples measured with 1 H-NMR
Research Square (Research Square)(2022)
摘要
Abstract Extracting accurate biological information from complex datasets is a main challenge in 1H-NMR-based metabolomics research. One of the crucial steps to achieve this goal is to apply an appropriate pretreatment method before multivariate data analysis in 1H-NMR based metabolomics studies. One of the most important pretreatment methods in metabolomics studies is scaling techniques. In this study, the effect of different pretreatment approaches such as auto-, pareto-, level-, range- and vast-scaling in addition to mean-centering are investigated on both experimental and simulated datasets. The goal is linear classification modeling of the toxicity induced by different doses of graphene oxide (GO) in metabolomics context, employing partial least squares- discriminant analysis) PLS-DA). The experimental dataset includes 1H-NMR spectra of mice serum samples exposed to different doses of GO nano-sheets. Here, it is shown that type of applied pre-treatment method has a considerable effect on data analysis results. In this study, auto-, pareto- and vast-scaling lead to a better separation of classes using PLS-DA modeling and PCA. From the results of this study, it was concluded that there is no general rule for the selection of the best scaling method in the analysis of 1H-NMR metabolomics datasets, and different ways of scaling should be tested.
更多查看译文
关键词
serum samples,different pretreatment methods,h-nmr
AI 理解论文
溯源树
样例
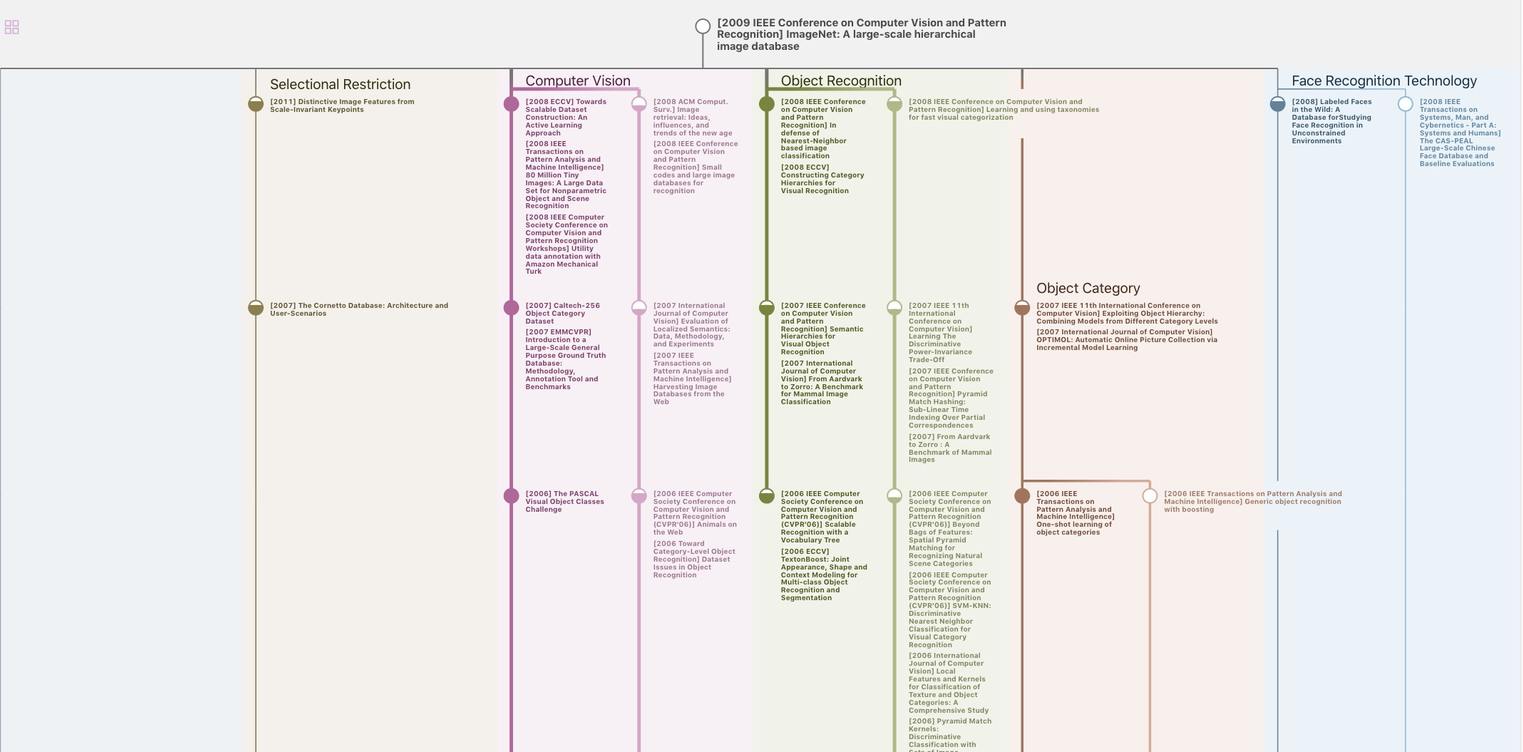
生成溯源树,研究论文发展脉络
Chat Paper
正在生成论文摘要